How Systematic Is The Environmental Sustainability Index 2002 As A Tool For Grouping Countries In Terms Of Their Environmental Sustainability?
2017 INTERNATIONAL JOINT CONFERENCE ON NEURAL NETWORKS (IJCNN)(2017)
摘要
With the aim of exploring the evolution of the Environmental Sustainability Index (ESI, 2000-2005), the earliest complete version of the index - namely, the ESI 2002 - is examined. In this piece of research, the investigation is specifically focused on the clustering of the participating countries into groups that demonstrate distinct ES-related characteristics, as these have been put forward and described in the primary relevant literature. The main two directions of this study cover (a) whether (and how accurately) the proposed clustering can be replicated, and (b) to what extent the reported clusters are consistent, i.e. how well the underlying groupings can be generalised to other (novel) countries. To this end, a variety of parametric and non-parametric clustering techniques - which tackle the problems of cluster creation as well as of cluster prediction - are applied, initially to the entire dataset and, subsequently to subsets of the dataset that are systematically derived via cross-validation. While self-organising maps are revealed as the most appropriate technique for recreating the clusters put forward in the relevant literature, probabilistic neural networks constitute the most accurate methodology for cluster prediction. The results concerning the overall performance attained by these techniques demonstrate that, although the means of creating the original (reported) clusters cannot be derived using either parametric or non-parametric methods, the generalisation/prediction potential of the proposed clustering is satisfactory, especially when using artificial neural network-based techniques.(1)
更多查看译文
关键词
Environmental Sustainability Index 2002, raw data, indicators, components, scores, country groups/clusters, verification, consistency, generalisation, prediction, parametric and non-parametric methodologies, statistical clustering, fuzzy clustering, self organising maps, probabilistic neural networks
AI 理解论文
溯源树
样例
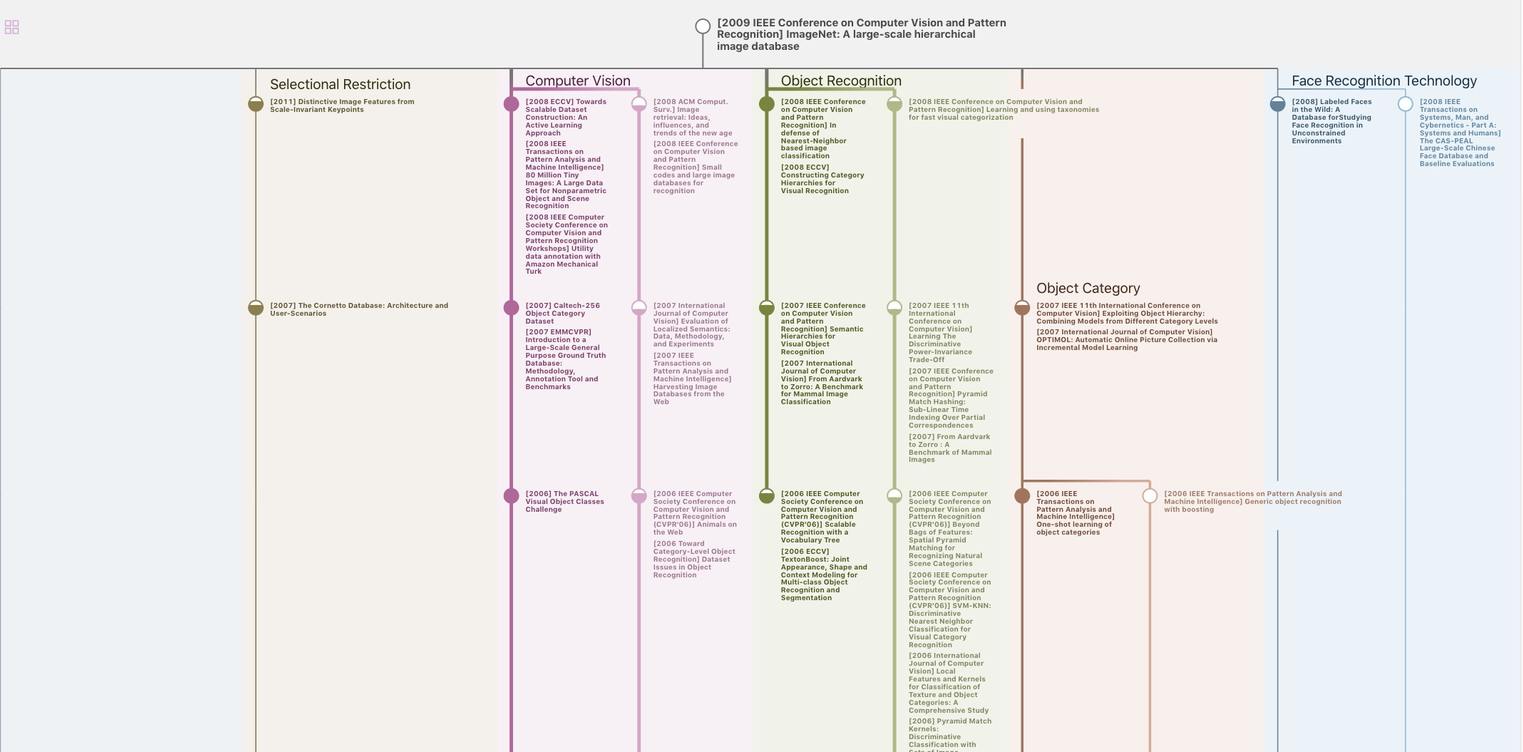
生成溯源树,研究论文发展脉络
Chat Paper
正在生成论文摘要