Weighting schemes and incomplete data: A generalized Bayesian framework for chance-corrected interrater agreement.
Psychological methods(2022)
摘要
Van Oest (2019) developed a framework to assess interrater agreement for nominal categories and complete data. We generalize this framework to all four situations of nominal or ordinal categories and complete or incomplete data. The mathematical solution yields a chance-corrected agreement coefficient that accommodates any weighting scheme for penalizing rater disagreements and any number of raters and categories. By incorporating Bayesian estimates of the category proportions, the generalized coefficient also captures situations in which raters classify only subsets of items; that is, incomplete data. Furthermore, this coefficient encompasses existing chance-corrected agreement coefficients: the S-coefficient, Scott's pi, Fleiss' kappa, and Van Oest's uniform prior coefficient, all augmented with a weighting scheme and the option of incomplete data. We use simulation to compare these nested coefficients. The uniform prior coefficient tends to perform best, in particular, if one category has a much larger proportion than others. The gap with Scott's pi and Fleiss' kappa widens if the weighting scheme becomes more lenient to small disagreements and often if more item classifications are missing; missingness biases play a moderating role. The uniform prior coefficient often performs much better than the S-coefficient, but the S-coefficient sometimes performs best for small samples, missing data, and lenient weighting schemes. The generalized framework implies a new interpretation of chance-corrected weighted agreement coefficients: These coefficients estimate the probability that both raters in a pair assign an item to its correct category without guessing. Whereas Van Oest showed this interpretation for unweighted agreement, we generalize to weighted agreement. (PsycInfo Database Record (c) 2023 APA, all rights reserved).
更多查看译文
关键词
interrater agreement,categorical data,weighting schemes,incomplete data
AI 理解论文
溯源树
样例
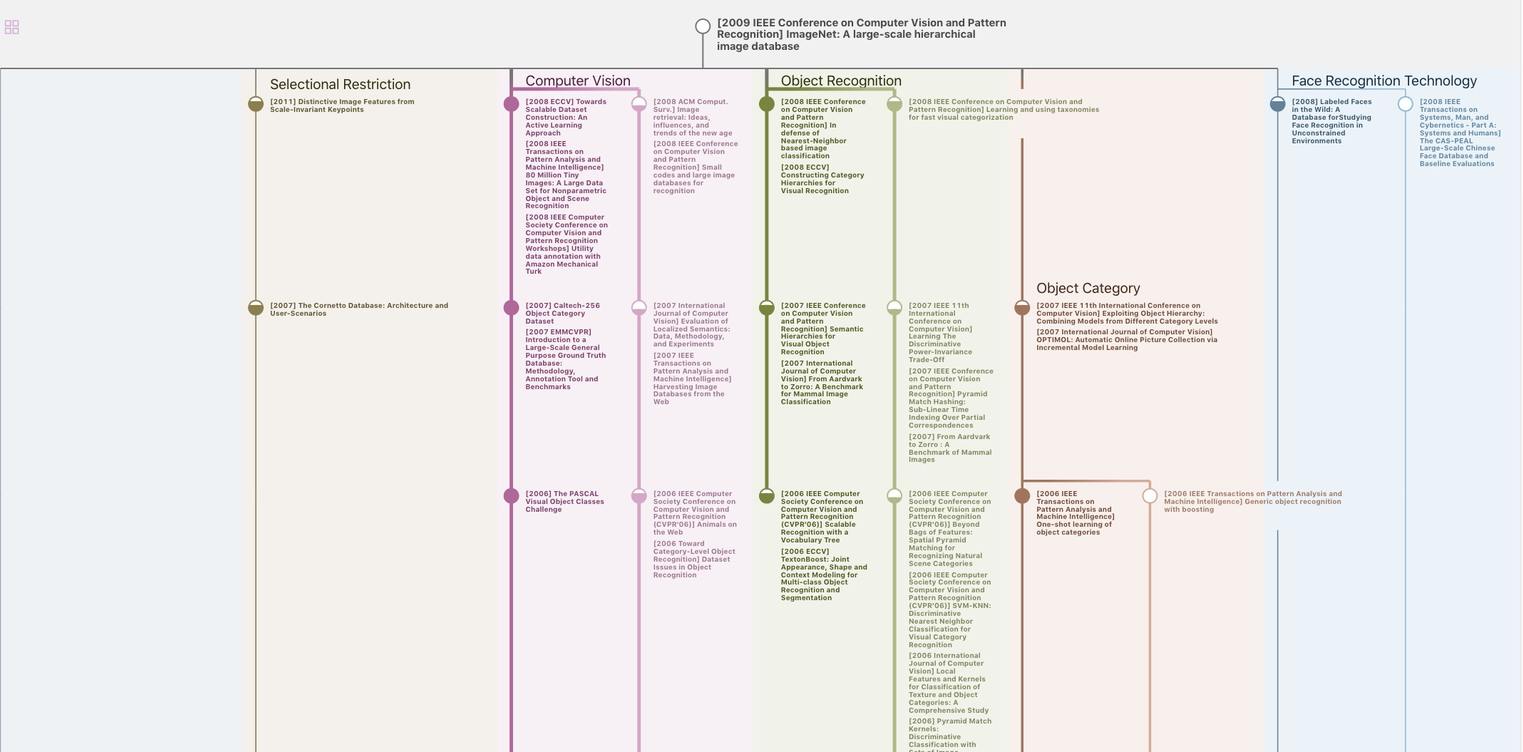
生成溯源树,研究论文发展脉络
Chat Paper
正在生成论文摘要