TADPOLE - Task ADapted Pre-Training via AnOmaLy DEtection.
EMNLP(2021)
摘要
The paradigm of pre-training followed by finetuning has become a standard procedure for NLP tasks, with a known problem of domain shift between the pre-training and downstream corpus. Previous works have tried to mitigate this problem with additional pre-training, either on the downstream corpus itself when it is large enough, or on a manually curated unlabeled corpus of a similar domain. In this paper, we address the problem for the case when the downstream corpus is too small for additional pre-training. We propose TADPOLE, a task adapted pre-training framework based on data selection techniques adapted from Domain Adaptation. We formulate the data selection as an anomaly detection problem that unlike existing methods works well when the downstream corpus is limited in size. It results in a scalable and efficient unsupervised technique that eliminates the need for any manual data curation. We evaluate our framework on eight tasks across four different domains: Biomedical, Computer Science, News, and Movie reviews, and compare its performance against competitive baseline techniques from the area of Domain Adaptation. Our framework outperforms all the baseline methods. On small datasets with less than 5K training examples, we get a gain of 1:82% in performance with additional pre-training for only 5% steps. It also compliments some of the other techniques such as data augmentation known for boosting performance when downstream corpus is small; highest performance is achieved when data augmentation is combined with task adapted pre-training.
更多查看译文
关键词
anomaly detection,task,pre-training
AI 理解论文
溯源树
样例
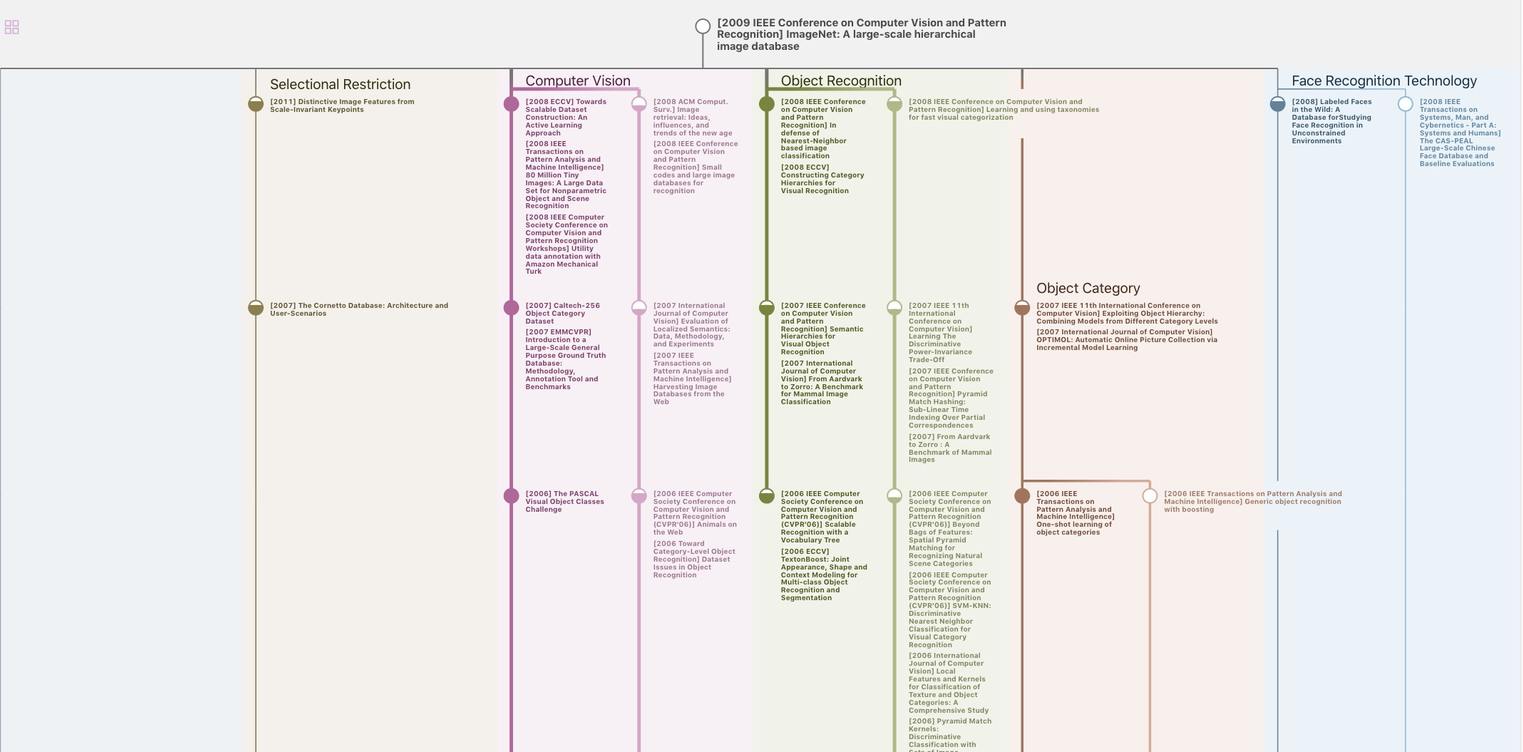
生成溯源树,研究论文发展脉络
Chat Paper
正在生成论文摘要