Neuro-Symbolic Approaches for Text-Based Policy Learning.
EMNLP(2021)
摘要
Text-Rased Games (TBGs) have emerged as important testbeds for reinforcement learning (RL) in the natural language domain. Previous methods using LSTM-based action policies are uninterpretable and often overfit the training games showing poor performance to unseen test games. We present SymboLic Action policy for Textual Environments (SLATE), that learns interpretable action policy rules from symbolic abstractions of textual observations for improved generalization. We outline a method for end-to-end differentiable symbolic rule learning and show that such symbolic policies outperform previous stateof-the-art methods in text-based RL for the coin collector environment from 5 - 10x fewer training games. Additionally, our method provides human-understandable policy rules that can be readily verified for their logical consistency and can be easily debugged.
更多查看译文
关键词
policy learning,neuro-symbolic,text-based
AI 理解论文
溯源树
样例
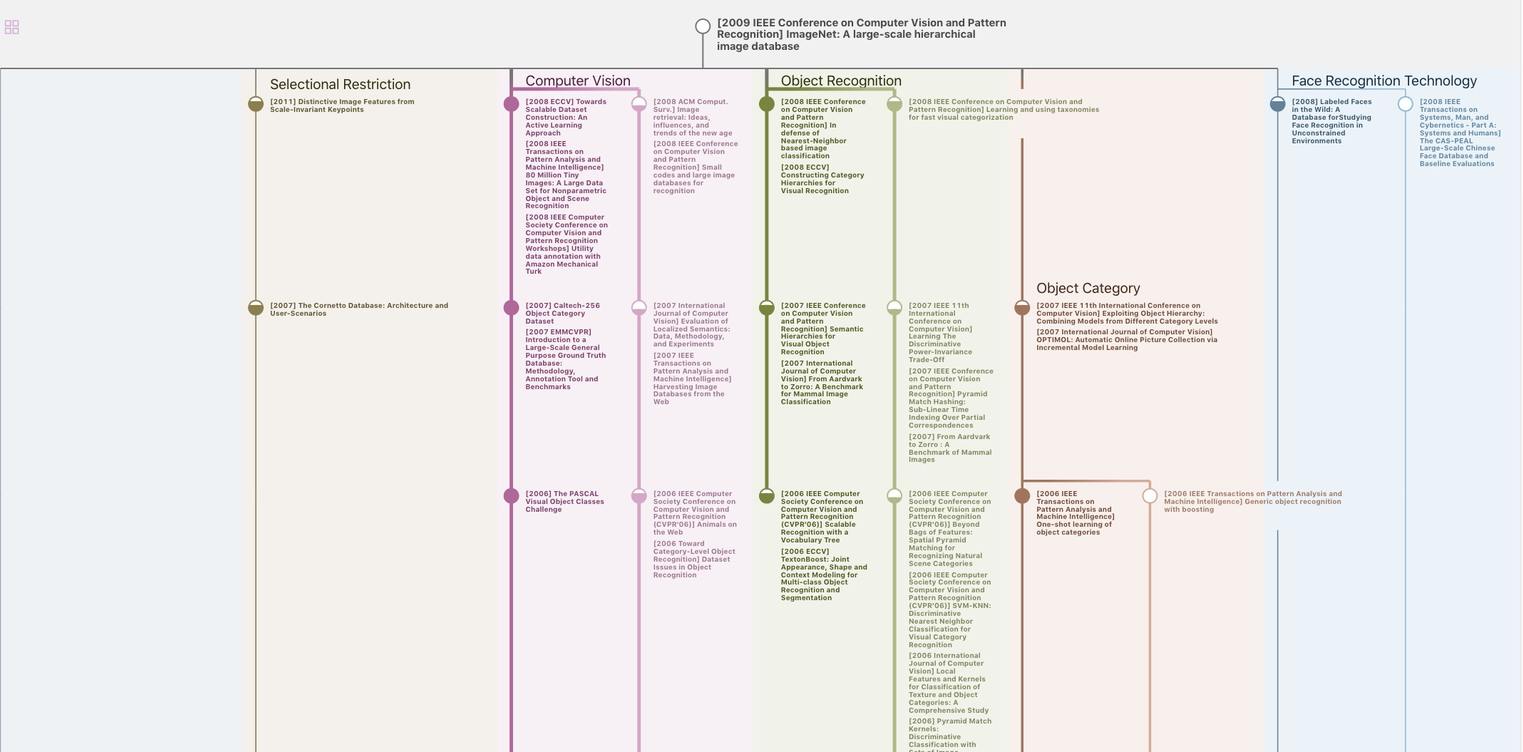
生成溯源树,研究论文发展脉络
Chat Paper
正在生成论文摘要