Towards Realistic Few-Shot Relation Extraction.
EMNLP(2021)
摘要
In recent years, few-shot models have been applied successfully to a variety of NLP tasks. Han et al. (2018) introduced a few-shot learning framework for relation classification, and since then, several models have surpassed human performance on this task, leading to the impression that few-shot relation classification is solved. In this paper we take a deeper look at the efficacy of strong few-shot classification models in the more common relation extraction setting, and show that typical few-shot evaluation metrics obscure a wide variability in performance across relations. In particular, we find that state of the art few-shot relation classification models overly rely on entity type information, and propose modifications to the training routine to encourage models to better discriminate between relations involving similar entity types.
更多查看译文
关键词
relation,few-shot
AI 理解论文
溯源树
样例
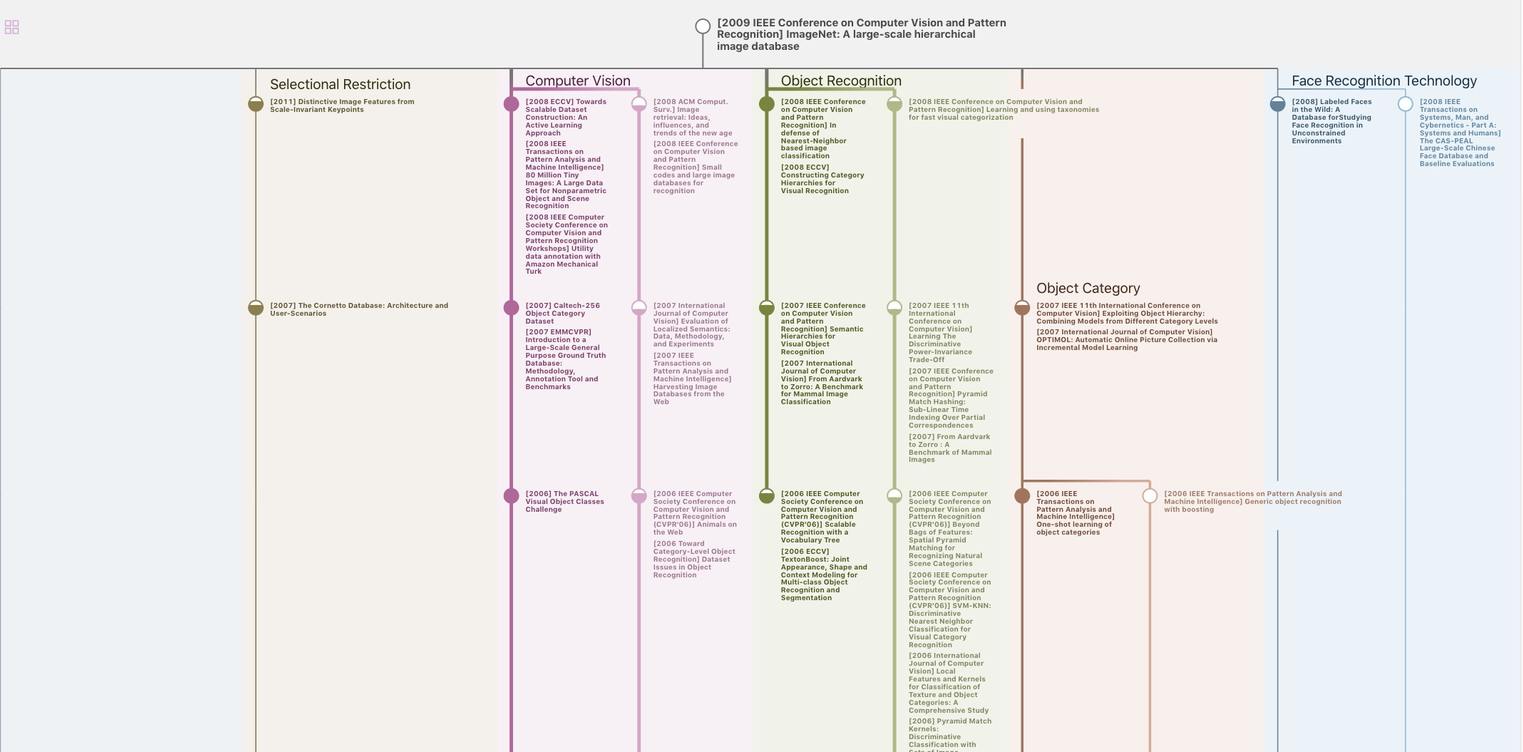
生成溯源树,研究论文发展脉络
Chat Paper
正在生成论文摘要