Syntax-aware neural machine translation directed by syntactic dependency degree
NEURAL COMPUTING & APPLICATIONS(2021)
摘要
There are various ways to incorporate syntax knowledge into neural machine translation (NMT). However, quantifying the dependency syntactic intimacy (DSI) between word pairs in a dependency tree has not being considered to use in attentional and transformer-based NMT. In this paper, we innovatively propose a variant of Tree-LSTM to capture the syntactic dependency degree (SDD) between word pairs in dependency trees. Two syntax-aware distances, including a tuned syntax distance and a ρ -dependent distance, are proposed. For attentional NMT, two syntax-aware attentions based on two syntax-aware distances are proposed for attentional NMT, and we also design a dual attention to simultaneously generate global context and dependency syntactic context. For transformer-based NMT, we explicitly incorporate the dependency syntax into self-attention network (SAN) to propose a syntax-aware SAN. Experiments on IWSLT’17 English–German, IWSLT Chinese–English and WMT’15 English–Finnish translation tasks show that our syntax-aware NMT significantly improves translation quality by comparing with baseline methods, even the state-of-the-art transformer-based NMT.
更多查看译文
关键词
Syntactic dependency degree,Syntax-aware distance,Syntax-aware attentions,Neural machine translation
AI 理解论文
溯源树
样例
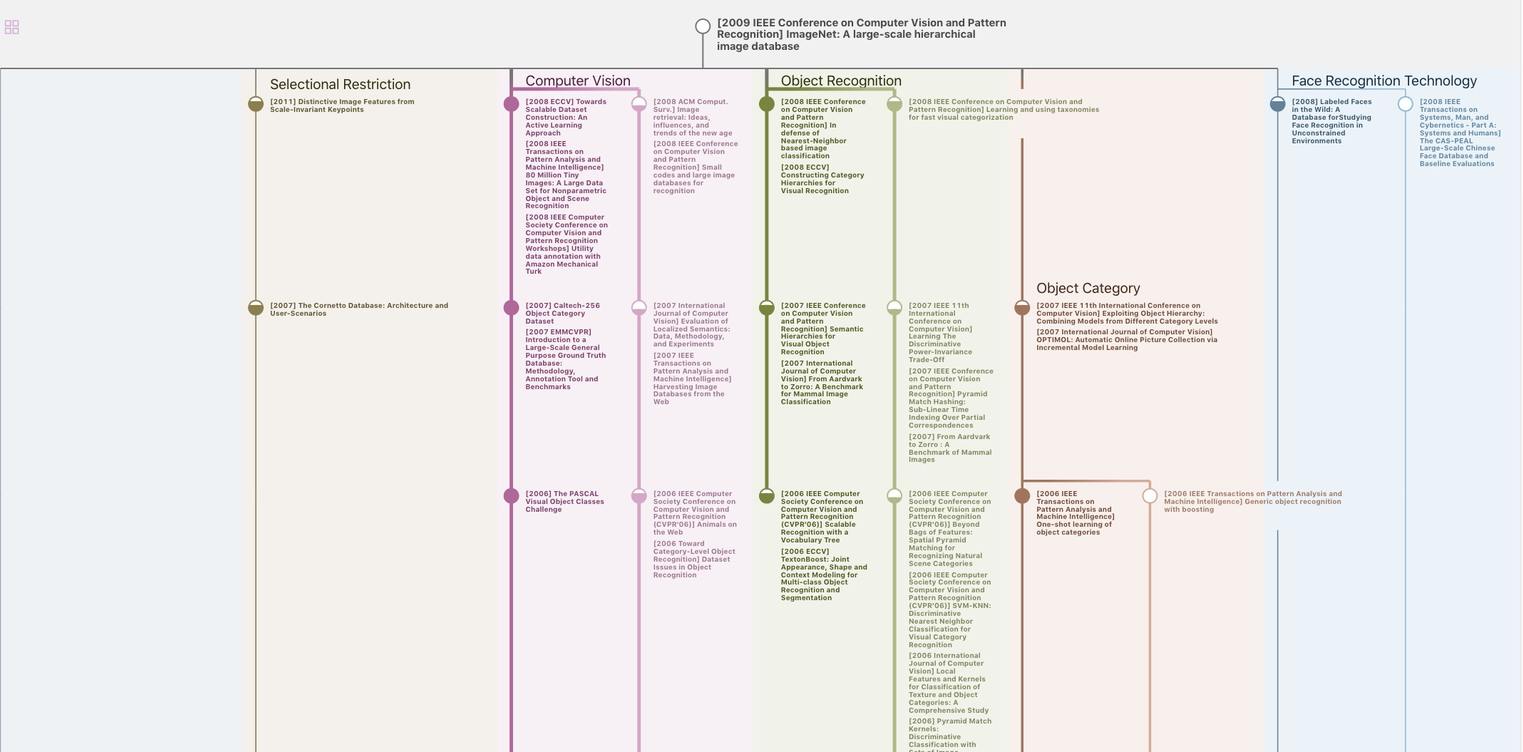
生成溯源树,研究论文发展脉络
Chat Paper
正在生成论文摘要