Predicting video engagement using heterogeneous DeepWalk
Neurocomputing(2021)
摘要
Video engagement is important in online advertisements where there is no physical interaction with the consumer. Engagement can be directly measured as the number of seconds after which a consumer skips an advertisement. In this paper, we propose a model to predict video engagement of an advertisement using only a few samples. This allows for early identification of poor quality videos. This can also help identify advertisement frauds where a robot runs fake videos behind the name of well-known brands. We leverage on the fact that videos with high engagement have similar viewing patterns over time. Hence, we can create a similarity network of videos and use a graph-embedding model called DeepWalk to cluster videos into significant communities. The learned embedding is able to identify viewing patterns of fraud and popular videos. In order to assess the impact of a video, we also consider how the view counts increase or decrease over time. This results in a heterogeneous graph where an edge indicates similar video engagement or history of view counts between two videos. Since it is difficult to find labelled samples for ‘fraud’ video, we leverage on a one-class model that can determine ‘fraud’ videos with outlier or abnormal behavior. The proposed model outperforms baselines in F-measure by over 20%.
更多查看译文
关键词
Video engagement,DeepWalk,Online behavior,One-class model
AI 理解论文
溯源树
样例
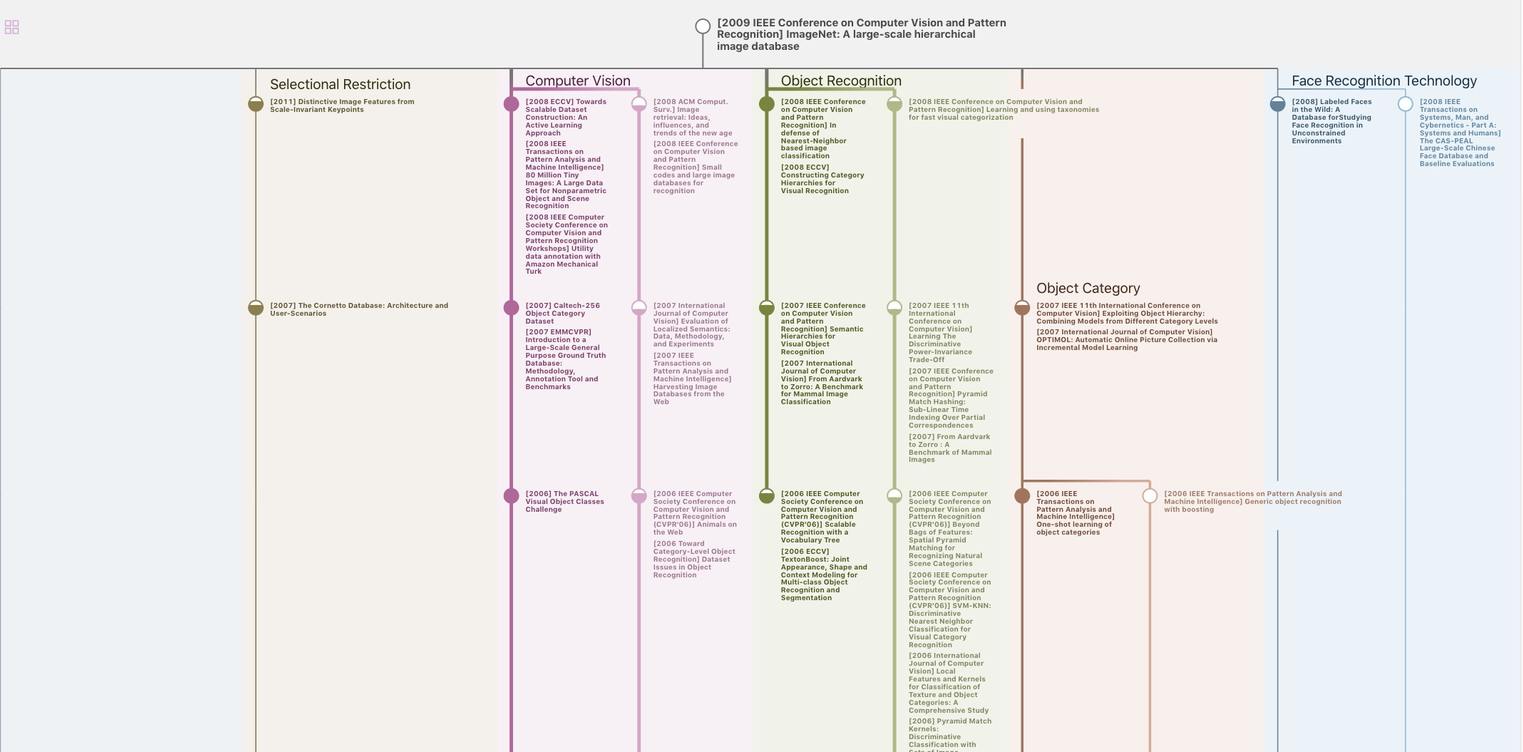
生成溯源树,研究论文发展脉络
Chat Paper
正在生成论文摘要