CTFN: Hierarchical Learning for Multimodal Sentiment Analysis Using Coupled-Translation Fusion Network.
Annual Meeting of the Association for Computational Linguistics(2021)
摘要
In this work, we demonstrate that the contextualized word vectors derived from pretrained masked language model-based encoders share a common, perhaps undesirable pattern across layers. Namely, we find cases of persistent outlier neurons within BERT and RoBERTa’s hidden state vectors that consistently bear the smallest or largest values in said vectors. In an attempt to investigate the source of this information, we introduce a neuron-level analysis method, which reveals that the outliers are closely related to information captured by positional embeddings. We also pre-train the RoBERTa-base models from scratch and find that the outliers disappear without using positional embeddings. These outliers, we find, are the major cause of anisotropy of encoders’ raw vector spaces, and clipping them leads to increased similarity across vectors. We demonstrate this in practice by showing that clipped vectors can more accurately distinguish word senses, as well as lead to better sentence embeddings when mean pooling. In three supervised tasks, we find that clipping does not affect the performance.
更多查看译文
关键词
multimodal sentiment analysis,hierarchical learning,ctfn,fusion,coupled-translation
AI 理解论文
溯源树
样例
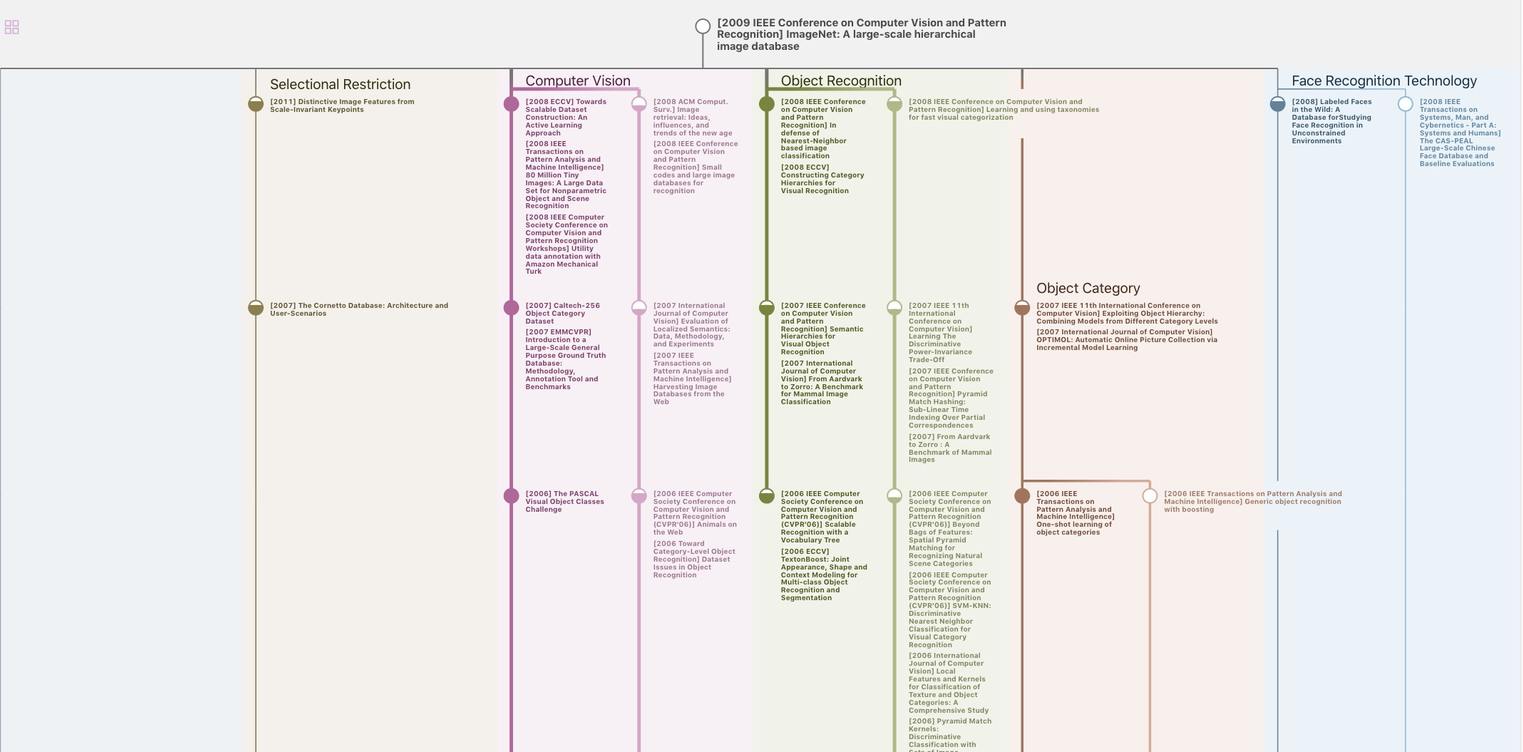
生成溯源树,研究论文发展脉络
Chat Paper
正在生成论文摘要