DP-REC: Private & Communication-Efficient Federated Learning
arxiv(2021)
摘要
Privacy and communication efficiency are important challenges in federated training of neural networks, and combining them is still an open problem. In this work, we develop a method that unifies highly compressed communication and differential privacy (DP). We introduce a compression technique based on Relative Entropy Coding (REC) to the federated setting. With a minor modification to REC, we obtain a provably differentially private learning algorithm, DP-REC, and show how to compute its privacy guarantees. Our experiments demonstrate that DP-REC drastically reduces communication costs while providing privacy guarantees comparable to the state-of-the-art.
更多查看译文
关键词
private,learning,dp-rec,communication-efficient
AI 理解论文
溯源树
样例
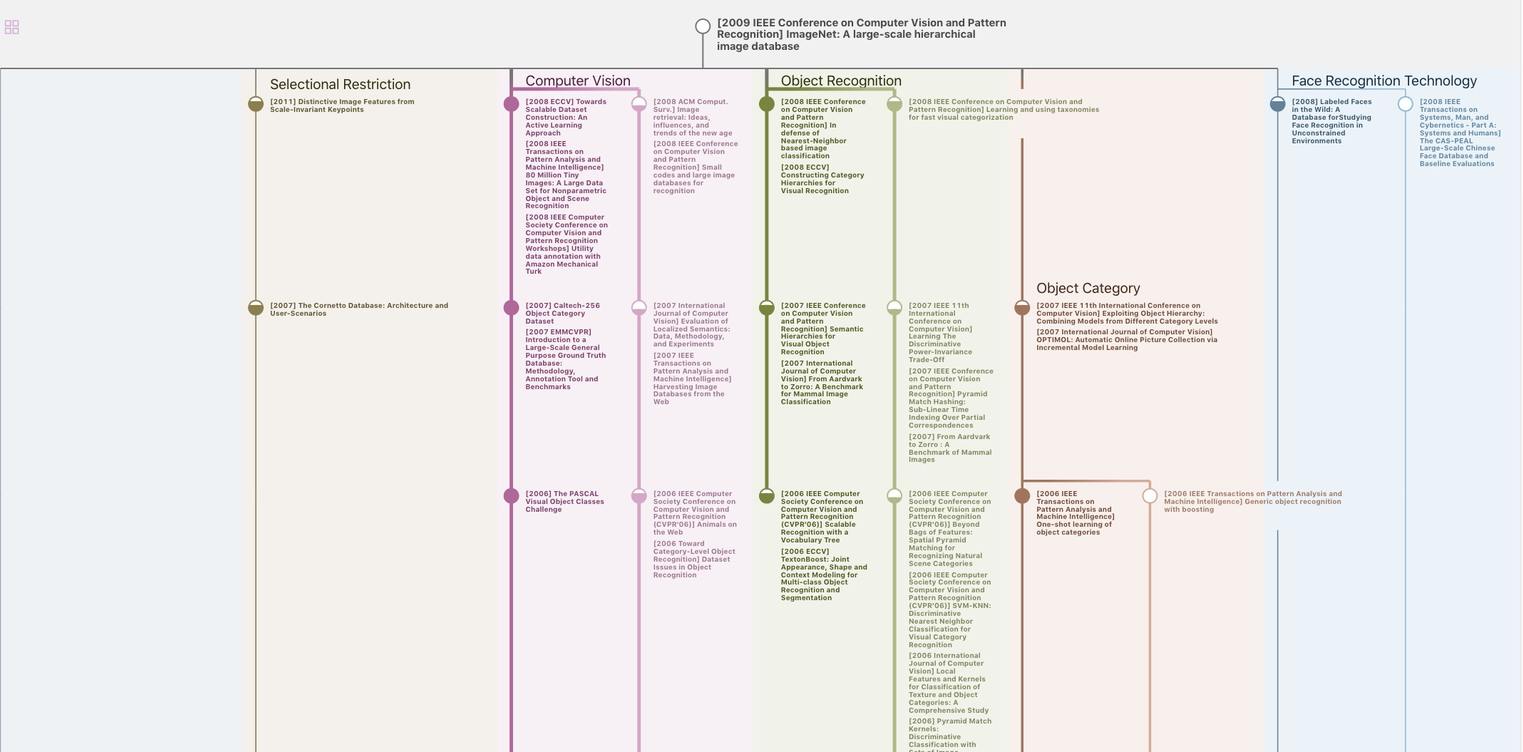
生成溯源树,研究论文发展脉络
Chat Paper
正在生成论文摘要