Robust Estimation for Random Graphs.
Annual Conference on Computational Learning Theory(2022)
摘要
We study the problem of robustly estimating the parameter $p$ of an Erdős-Rényi random graph on $n$ nodes, where a $\gamma$ fraction of nodes may be adversarially corrupted. After showing the deficiencies of canonical estimators, we design a computationally-efficient spectral algorithm which estimates $p$ up to accuracy $\tilde O(\sqrt{p(1-p)}/n + \gamma\sqrt{p(1-p)} /\sqrt{n}+ \gamma/n)$ for $\gamma < 1/60$. Furthermore, we give an inefficient algorithm with similar accuracy for all $\gamma<1/2$, the information-theoretic limit. Finally, we prove a nearly-matching statistical lower bound, showing that the error of our algorithms is optimal up to logarithmic factors.
更多查看译文
关键词
robust estimation,random graphs
AI 理解论文
溯源树
样例
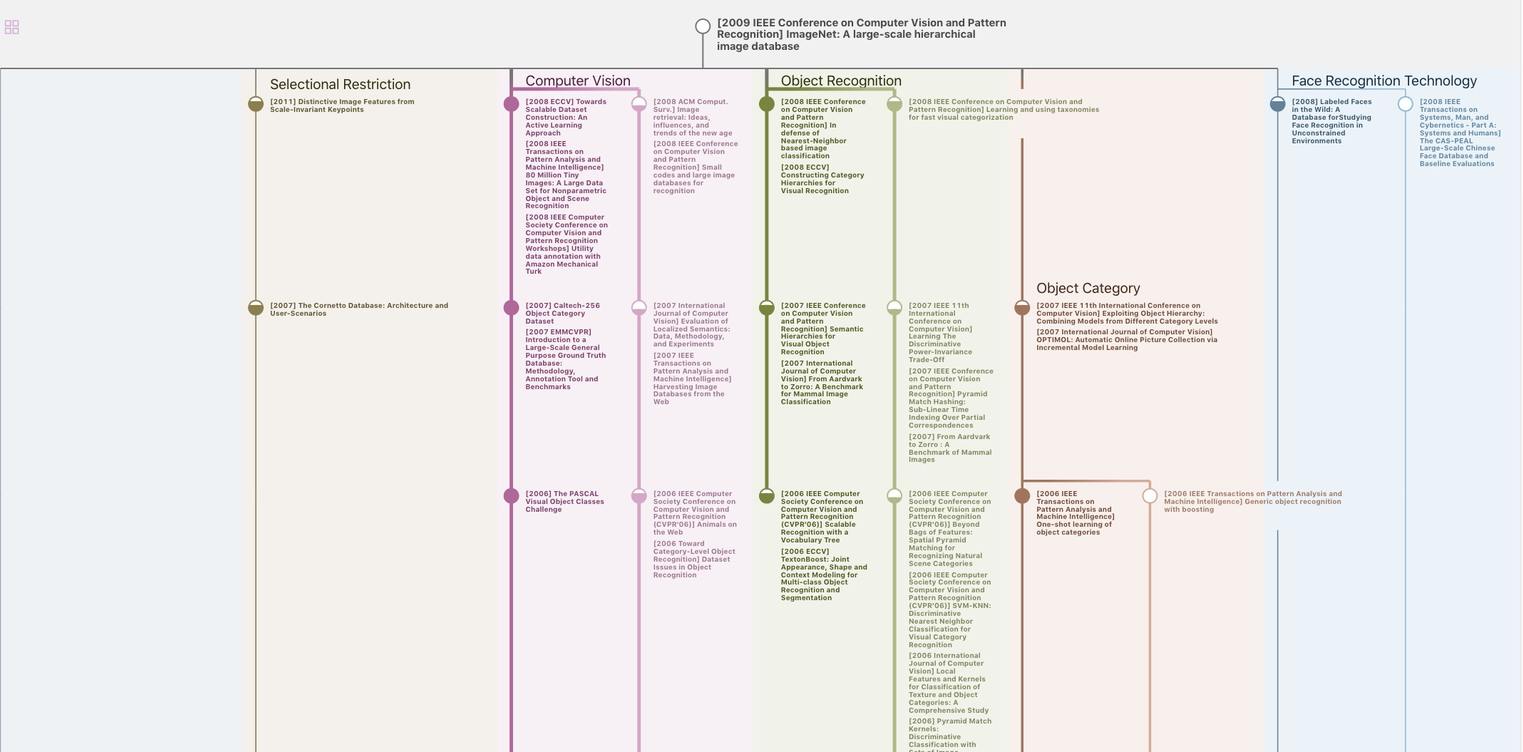
生成溯源树,研究论文发展脉络
Chat Paper
正在生成论文摘要