Mitigating Domain Shift in AI-Based TB Screening With Unsupervised Domain Adaptation
IEEE ACCESS(2022)
摘要
We demonstrate that Domain Invariant Feature Learning (DIFL) can improve the out-of-domain generalizability of a deep learning Tuberculosis (TB) screening algorithm. It is well known that state of the art deep learning algorithms often have difficulty generalizing to unseen data distributions due to "domain shift." In the context of medical imaging, this could lead to unintended biases such as the inability to generalize from one patient population to another. We analyze the performance of a ResNet-50 classifier for the purposes of TB screening using the four most popular public datasets with geographically diverse sources of imagery. We show that without domain adaptation, ResNet-50 has difficulty in generalizing between imaging distributions from a number of public TB screening datasets with imagery from geographically distributed regions. However, with the incorporation of DIFL, the out-of-domain performance is greatly enhanced. Analysis criteria includes a comparison of accuracy, sensitivity, specificity and AUC over both the baseline, as well as the DIFL enhanced algorithms. We conclude that DIFL improves generalizability of TB screening while maintaining acceptable accuracy over the source domain imagery when applied across a variety of public datasets.
更多查看译文
关键词
Biomedical imaging, Task analysis, Deep learning, X-ray imaging, Statistics, Sociology, Diseases, Tuberculosis, X-ray imaging, domain adaptation, domain invariant feature learning, generative adversarial networks, deep learning, computer vision, computer aided diagnosis, DIFL
AI 理解论文
溯源树
样例
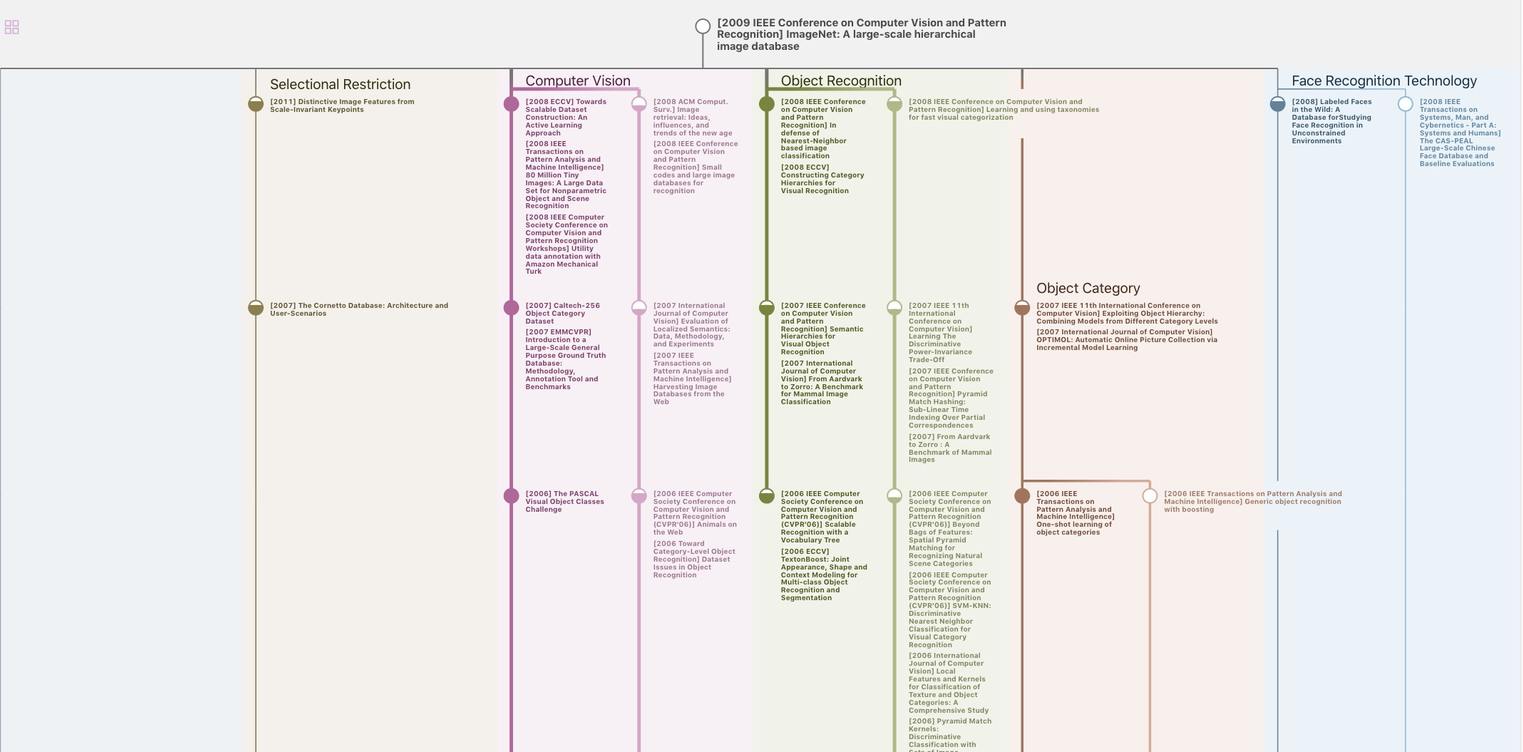
生成溯源树,研究论文发展脉络
Chat Paper
正在生成论文摘要