ANF - Attention-Based Noise Filtering Strategy for Unsupervised Few-Shot Classification.
PRICAI(2021)
摘要
How to learn concepts from few-shot samples remains an open challenge in the deep learning era. The previous meta-learning methods require a large number of annotated samples in the training phase, which still contributes to high manual-labeling costs. In this paper, we propose a unsupervised few-shot learning framework and pointed out that negative queue constructed via randomly sampling contains many false-negative samples (noise), which has negative impacts on the model’s generalized performance especially when only few samples are available. Specially, we propose an Attention-based Noise Filtering (ANF) strategy to make momentum contrastive loss more applicable to few-shot learning scenario. In addition, we also propose a dynamic momentum update method, which can greatly improve the classification accuracy. Our evaluations demonstrate state-of-the-art unsupervised few-shot learning performance, which is comparable to supervised baseline models.
更多查看译文
关键词
Self-supervised, Unsupervised few-shot learning, Attention mechanism, Computer vision
AI 理解论文
溯源树
样例
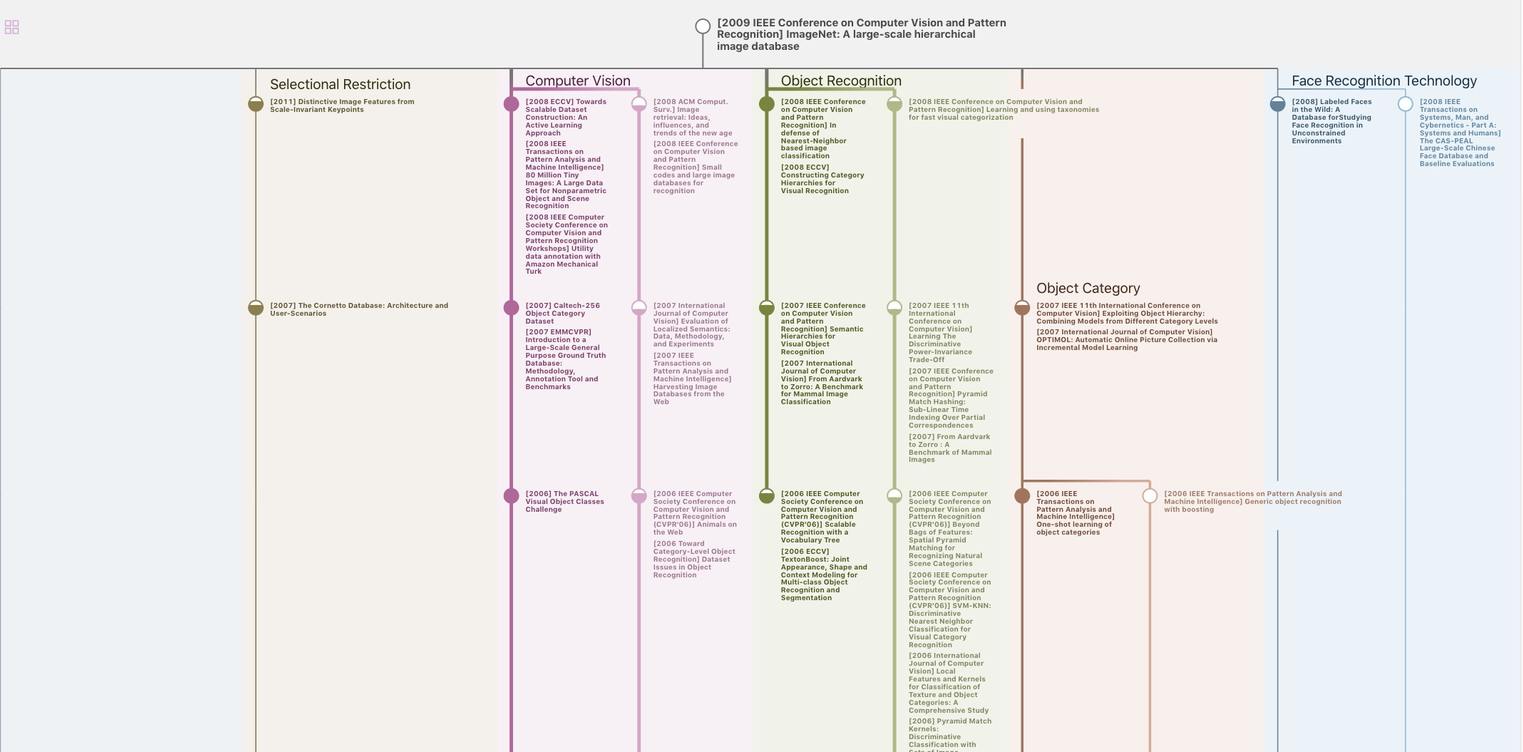
生成溯源树,研究论文发展脉络
Chat Paper
正在生成论文摘要