Autoregressive Pre-training Model-Assisted Low-Resource Neural Machine Translation.
PRICAI(2021)
摘要
Pre-training methods have been proven to significantly improve language understanding ability of the model. However, when dealing with machine translation tasks involving two or more languages, the pre-training method can only handle a single language and prevent further improvement of machine translation performance. Therefore, there are two main methods to improve the quality of machine translation model by using the pre-training model. One is to use the word embedding generated by the pre-training model as the modeling unit. Second is to make the machine translation model learn the probability distribution of the pre-training model through the knowledge distillation method. In addition, the self-attention based pre-training model affects the effect of machine translation due to the “training-fine-tuning” difference and limited by the assumption of conditional independence. For this reason, we proposed a XLNet based pre-training method, that corrects the defects of the general self-encoding based pre-training model, and enhance NMT model for context feature extraction. We conducted experiments on the CCMT2019 Mongolian-Chinese (Mo-Zh), Uyghur-Chinese (Ug-Zh) and Tibetan-Chinese (Ti-Zh) tasks, our method significantly improves the quality compared to the baseline (Transformer), which fully verifies the effectiveness.
更多查看译文
关键词
Pre-training, XLNet, Low-resource, Machine translation
AI 理解论文
溯源树
样例
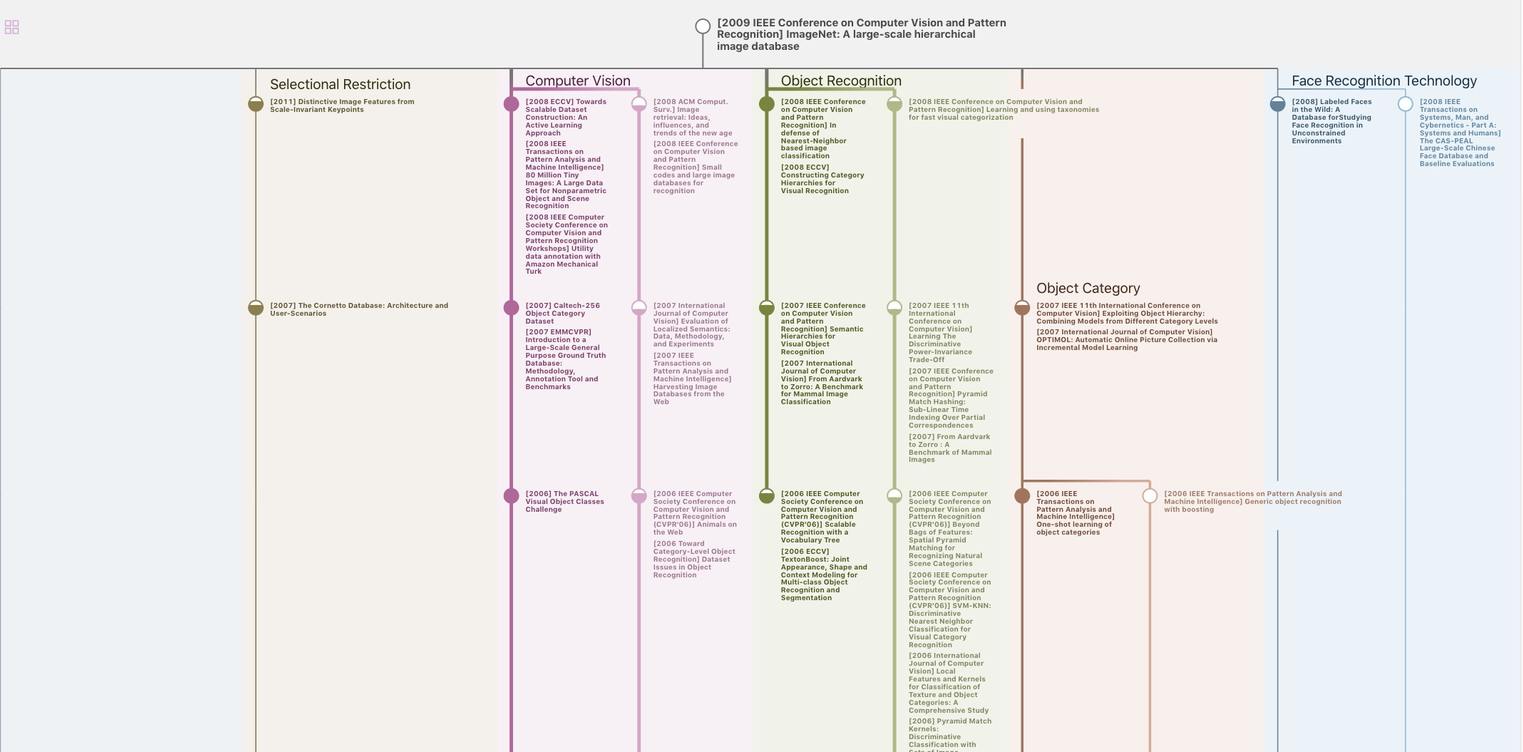
生成溯源树,研究论文发展脉络
Chat Paper
正在生成论文摘要