Chebyshev approaches for imbalanced data streams regression models
DATA MINING AND KNOWLEDGE DISCOVERY(2021)
摘要
In recent years data stream mining and learning from imbalanced data have been active research areas. Even though solutions exist to tackle these two problems, most of them are not designed to handle challenges inherited from both problems. As far as we are aware, the few approaches in the area of learning from imbalanced data streams fall in the context of classification, and no efforts on the regression domain have been reported yet. This paper proposes a technique that uses sampling strategies to cope with imbalanced data streams in a regression setting, where the most important cases have rare and extreme target values. Specifically, we employ under-sampling and over-sampling strategies that resort to Chebyshev’s inequality value as a heuristic to disclose the type of incoming cases (i.e. frequent or rare). We have evaluated our proposal by applying it in the training of models by four well-known regression algorithms over fourteen benchmark data sets. We conducted a series of experiments with different setups on both synthetic and real-world data sets. The experimental results confirm our approach’s effectiveness by showing the models’ superior performance trained by each of the sampling strategies compared with their baseline pairs.
更多查看译文
关键词
Data streams,Imbalanced data streams,Regression
AI 理解论文
溯源树
样例
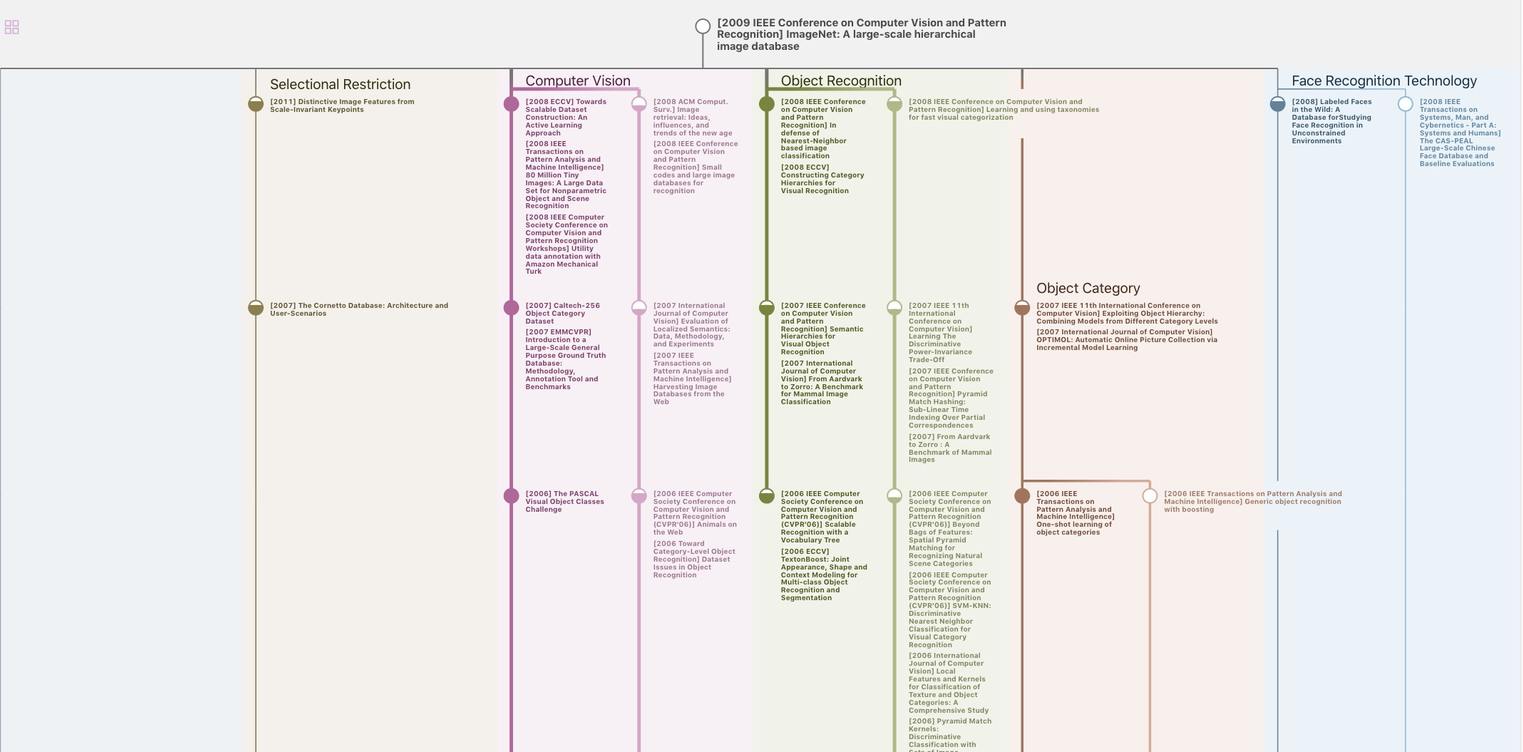
生成溯源树,研究论文发展脉络
Chat Paper
正在生成论文摘要