Learning Video Actions In Two Stream Recurrent Neural Network
PATTERN RECOGNITION LETTERS(2021)
摘要
The paper investigates the Long short term memory (LSTM) networks for human action recognition in videos. In spite of significant progress in the field, recognizing actions in real-world videos is a challenging task due to the spatial and temporal variations within and across video clips. We propose a novel two-stream deep network for action recognition by applying the LSTM for learning the fusion of spatial and temporal feature streams. The LSTM type of Recurrent neural network by design possess unique capability to preserve long range context in temporal streams. The proposed method capitalizes on LSTMs memory attribute to fuse the input streams in high-dimensional space exploring the spatial and temporal correlations. The temporal stream input is defined on the LSTM learned deep features summarizing the input frame sequence. Our approach of combining the convolutional features based spatial stream and the deep features based temporal stream in LSTM network efficiently captures the long range temporal dependencies in video streams. We perform primary evaluation of the proposed approach on UCF101, HMBD51 and Kinetics400 datasets achieving competitive recognition accuracy of 93.1%, 71.3% and 74.6% respectively. (c) 2021 Elsevier B.V. All rights reserved.
更多查看译文
关键词
Action recognition, Two-stream deep network, LSTM, Feature fusion
AI 理解论文
溯源树
样例
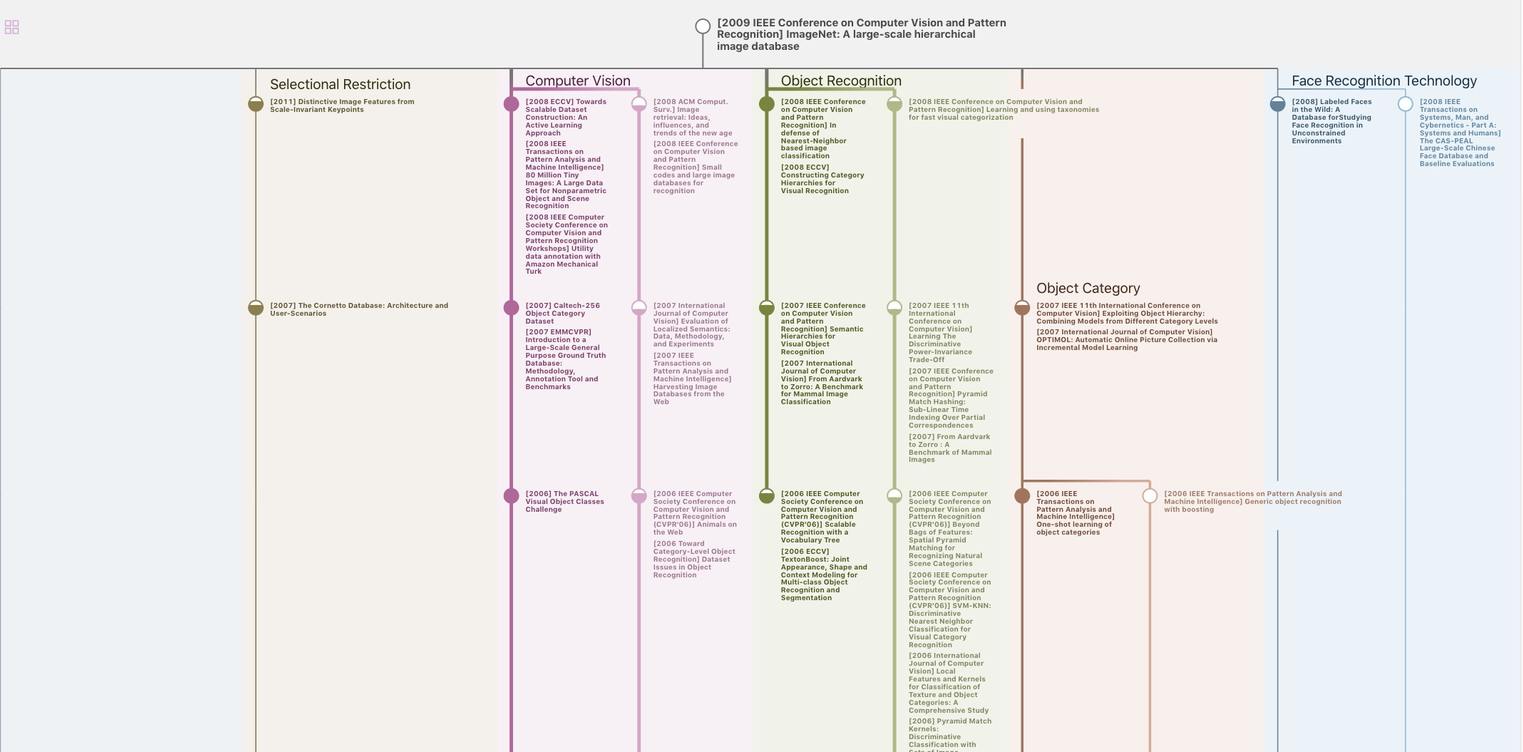
生成溯源树,研究论文发展脉络
Chat Paper
正在生成论文摘要