Tuning Materials-Binding Peptide Sequences toward Gold- and Silver-Binding Selectivity with Bayesian Optimization
ACS NANO(2021)
摘要
Peptide sequence engineering can potentially deliver materials-selective binding capabilities, which would be highly attractive in numerous biotic and abiotic nanomaterials applications. However, the number of known materials-selective peptide sequences is small, and identification of new sequences is laborious and haphazard. Previous attempts have sought to use machine learning and other informatics approaches that rely on existing data sets to accelerate the discovery of materials-selective peptides, but too few materials-selective sequences are known to enable reliable prediction. Moreover, this knowledge base is expensive to expand. Here, we combine a comprehensive and integrated experimental and modeling effort and introduce a Bayesian Effective Search for Optimal Sequences (BESOS) approach to address this challenge. Through this combined approach, we significantly expand the data set of Au-selective peptide sequences and identify an additional Ag-selective peptide sequence. Analysis of the binding motifs for the Ag-binders offers a roadmap for future prediction with machine learning, which should guide identification of further Ag-selective sequences. These discoveries will enable wider and more versatile integration of Ag nanoparticles in biological platforms.
更多查看译文
关键词
materials-selective peptides, Bayesian optimization, nanoparticles, noble metals, interfaces
AI 理解论文
溯源树
样例
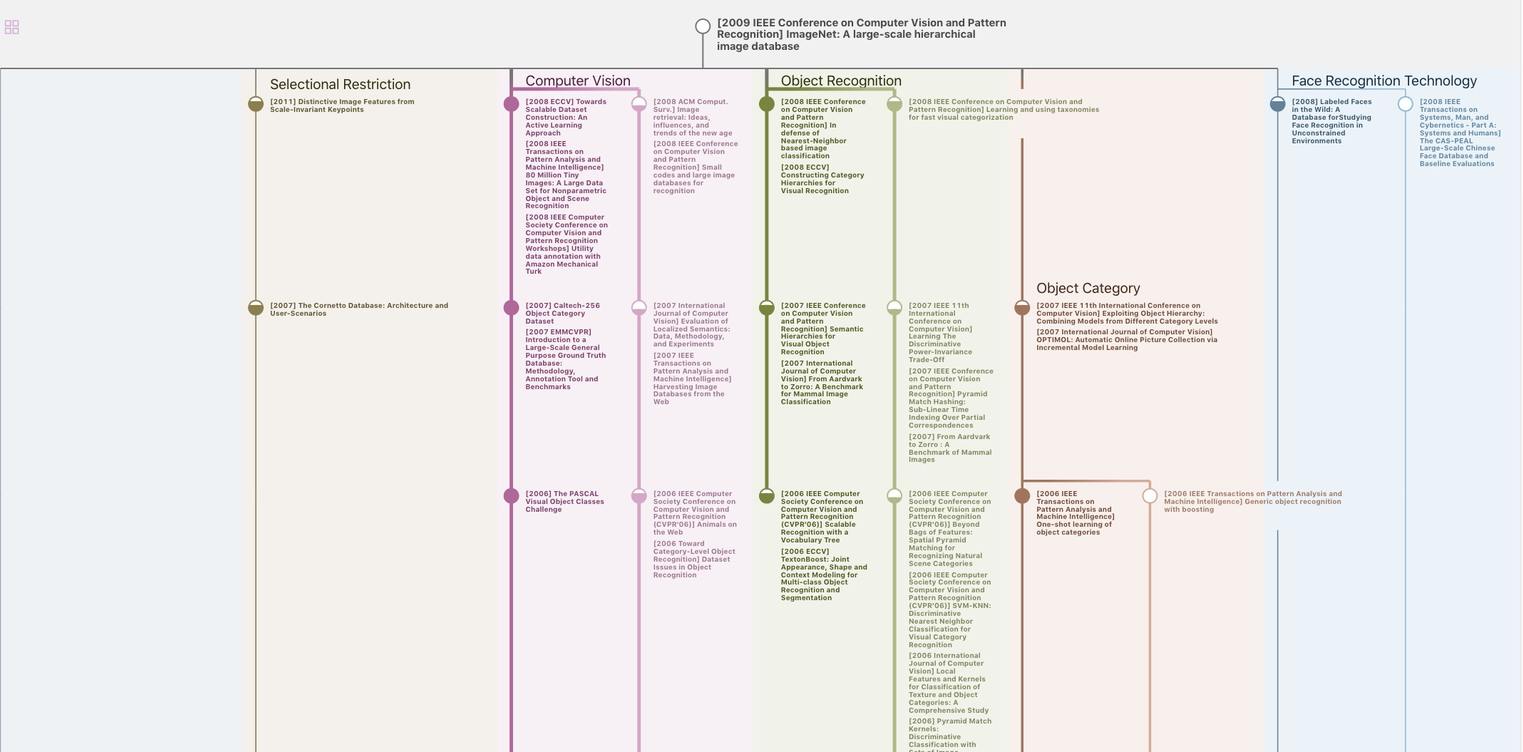
生成溯源树,研究论文发展脉络
Chat Paper
正在生成论文摘要