Self-Supervised Pretraining For Transferable Quantitative Phase Image Cell Segmentation
BIOMEDICAL OPTICS EXPRESS(2021)
摘要
In this paper, a novel U-Net-based method for robust adherent cell segmentation for quantitative phase microscopy image is designed and optimised. We designed and evaluated four specific post-processing pipelines. To increase the transferability to different cell types, non-deep learning transfer with adjustable parameters is used in the post-processing step. Additionally, we proposed a self-supervised pretraining technique using nonlabelled data, which is trained to reconstruct multiple image distortions and improved the segmentation performance from 0.67 to 0.70 of object-wise intersection over union. Moreover, we publish a new dataset of manually labelled images suitable for this task together with the unlabelled data for self-supervised pretraining. (c) 2021 Optical Society of America under the terms of the OSA Open Access Publishing Agreement
更多查看译文
关键词
pretraining,cell,phase,self-supervised
AI 理解论文
溯源树
样例
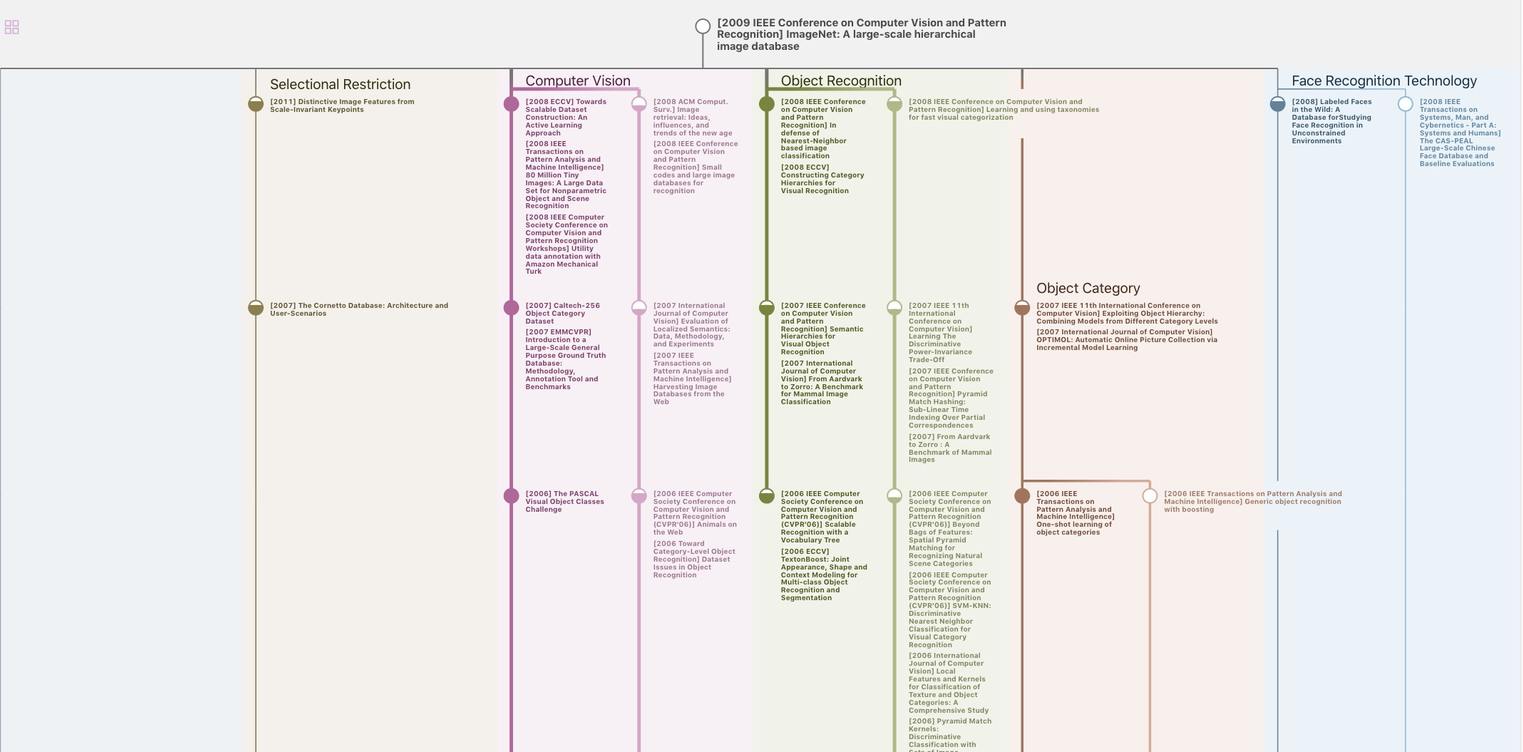
生成溯源树,研究论文发展脉络
Chat Paper
正在生成论文摘要