Dimensional reduction based on peak fitting of Raman micro spectroscopy data improves detection of prostate cancer in tissue specimens
JOURNAL OF BIOMEDICAL OPTICS(2021)
摘要
Significance: Prostate cancer is the most common cancer among men. An accurate diagnosis of its severity at detection plays a major role in improving their survival. Recently, machine learning models using biomarkers identified from Raman micro-spectroscopy discriminated intraductal carcinoma of the prostate (IDC-P) from cancer tissue with a >85% detection accuracy and differentiated high-grade prostatic intraepithelial neoplasia (HGPIN) from IDC-P with a >= 97.8% accuracy. Aim: To improve the classification performance of machine learning models identifying different types of prostate cancer tissue using a new dimensional reduction technique. Approach: A radial basis function (RBF) kernel support vector machine (SVM) model was trained on Raman spectra of prostate tissue from a 272-patient cohort (Centre hospitalier de l'Universite de Montreal, CHUM) and tested on two independent cohorts of 76 patients [University Health Network (UHN)] and 135 patients (Centre hospitalier universitaire de Quebec-Universite Laval, CHUQc-UL). Two types of engineered features were used. Individual intensity features, i.e., Raman signal intensity measured at particular wavelengths and novel Raman spectra fitted peak features consisting of peak heights and widths. Results: Combining engineered features improved classification performance for the three aforementioned classification tasks. The improvements for IDC-P/cancer classification for the UHN and CHUQc-UL testing sets in accuracy, sensitivity, specificity, and area under the curve (AUC) are (numbers in parenthesis are associated with the CHUQc-UL testing set): +4% (+8%), +7% (+9%), +2% (6%), +9 (+9) with respect to the current best models. Discrimination between HGPIN and IDC-P was also improved in both testing cohorts: +2.2% (+1.7%), +4.5% (+3.6%), +0% (+0%), +2.3 (+0). While no global improvements were obtained for the normal versus cancer classification task [+0% (-2%), +0% (-3%), +2% (-2%), +4 (+3)1 the AUC was improved in both testing sets. Conclusions: Combining individual intensity features and novel Raman fitted peak features, improved the classification performance on two independent and multicenter testing sets in comparison to using only individual intensity features. (C) The Authors.
更多查看译文
关键词
machine learning, Raman micro-spectroscopy, prostate cancer, feature selection, feature reduction
AI 理解论文
溯源树
样例
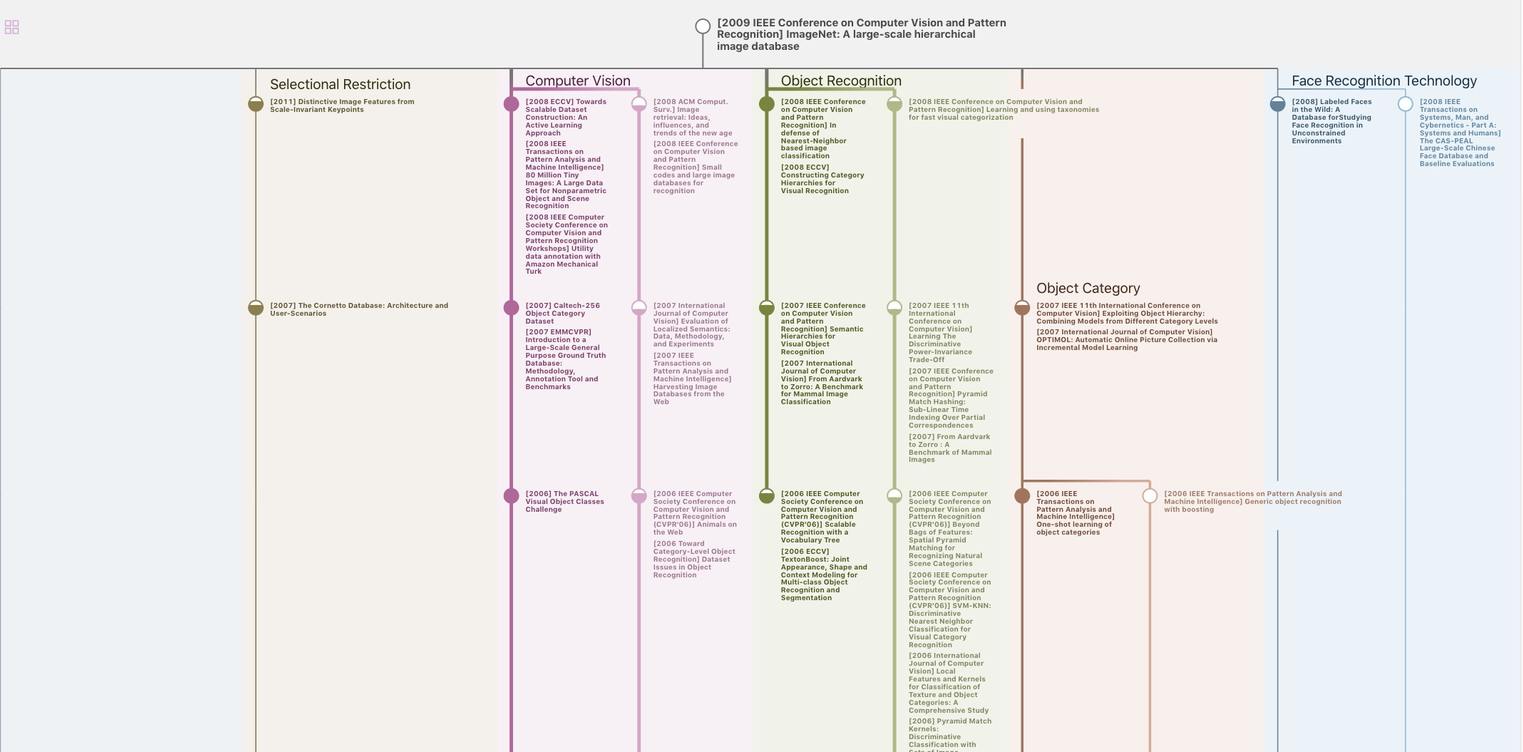
生成溯源树,研究论文发展脉络
Chat Paper
正在生成论文摘要