Contextual Unsupervised Outlier Detection in Sequences
arxiv(2021)
摘要
This work proposes an unsupervised learning framework for trajectory (sequence) outlier detection that combines ranking tests with user sequence models. The overall framework identifies sequence outliers at a desired false positive rate (FPR), in an otherwise parameter-free manner. We evaluate our methodology on a collection of real and simulated datasets based on user actions at the websites last.fm and msnbc.com, where we know ground truth, and demonstrate improved accuracy over existing approaches. We also apply our approach to a large real-world dataset of Pinterest and Facebook users, where we find that users tend to re-share Pinterest posts of Facebook friends significantly more than other types of users, pointing to a potential influence of Facebook friendship on sharing behavior on Pinterest.
更多查看译文
关键词
contextual unsupervised outlier detection,sequences
AI 理解论文
溯源树
样例
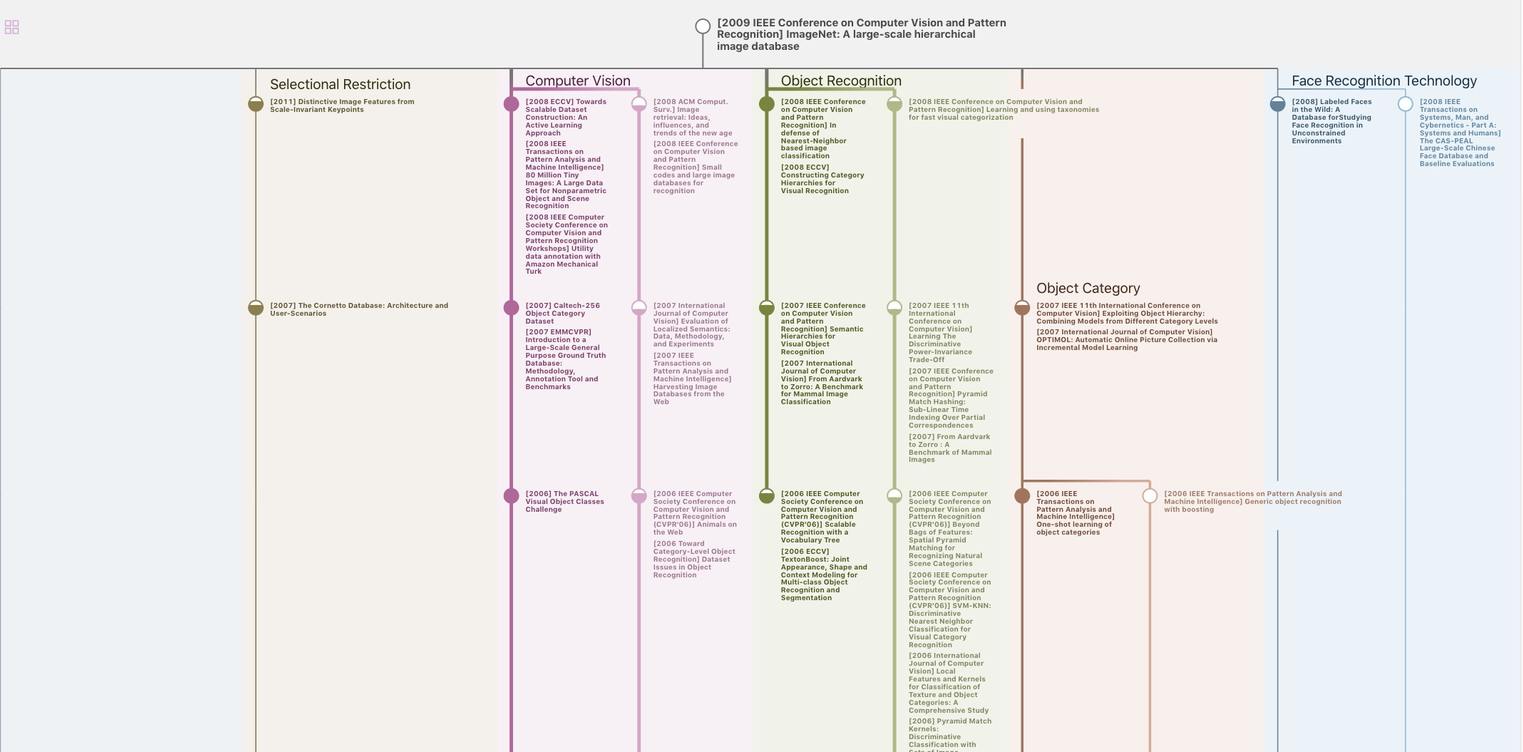
生成溯源树,研究论文发展脉络
Chat Paper
正在生成论文摘要