Privacy Attacks for Automatic Speech Recognition Acoustic Models in A Federated Learning Framework.
IEEE International Conference on Acoustics, Speech, and Signal Processing (ICASSP)(2022)
摘要
This paper investigates methods to effectively retrieve speaker information from the personalized speaker adapted neural network acoustic models (AMs) in automatic speech recognition (ASR). This problem is especially important in the context of federated learning of ASR acoustic models where a global model is learnt on the server based on the updates received from multiple clients. We propose an approach to analyze information in neural network AMs based on a neural network footprint on the so-called Indicator dataset. Using this method, we develop two attack models that aim to infer speaker identity from the updated personalized models without access to the actual users' speech data. Experiments on the TED-LIUM 3 corpus demonstrate that the proposed approaches are very effective and can provide equal error rate (EER) of 1-2%.
更多查看译文
关键词
Privacy,federated learning,acoustic models,attack models,speech recognition,speaker verification
AI 理解论文
溯源树
样例
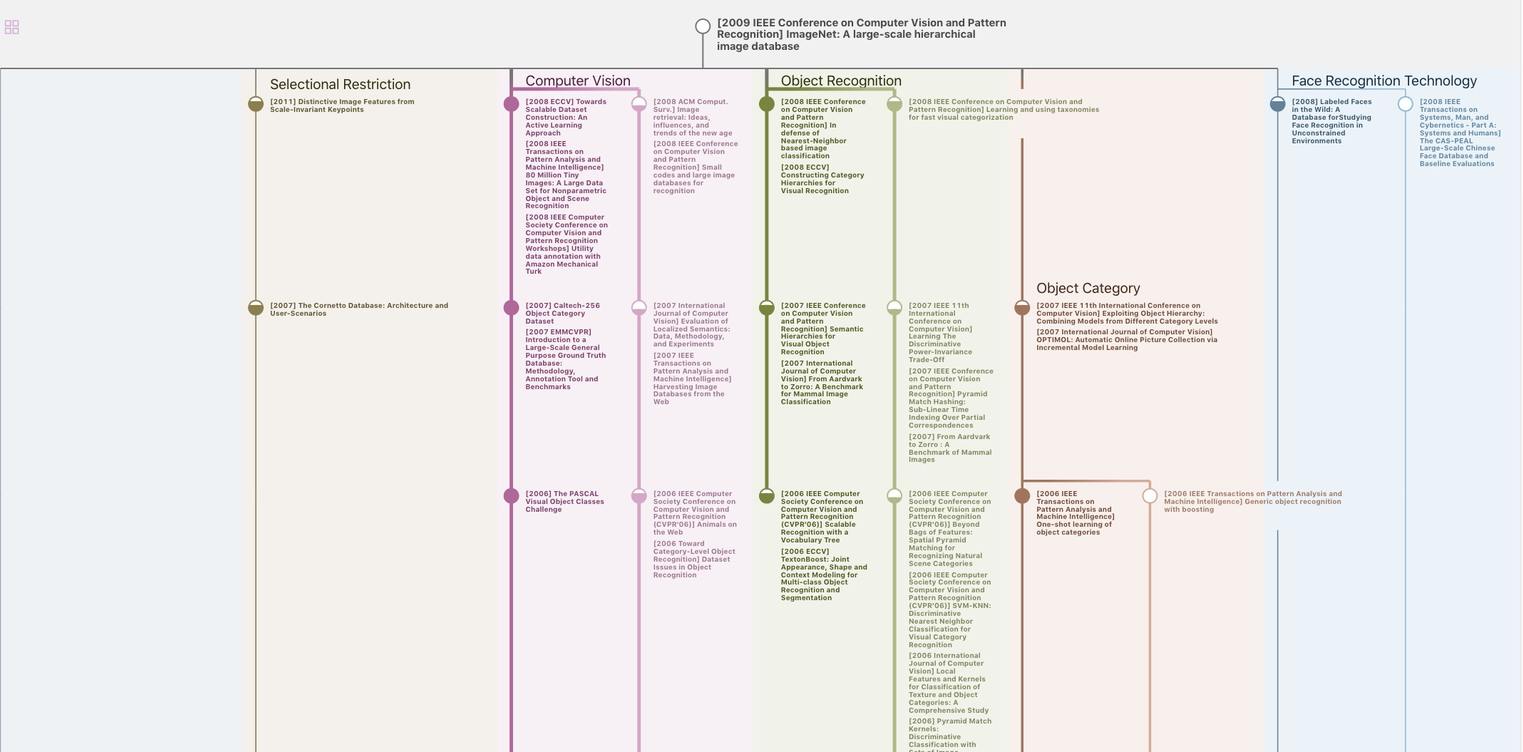
生成溯源树,研究论文发展脉络
Chat Paper
正在生成论文摘要