Asynchronous Collaborative Localization by Integrating Spatiotemporal Graph Learning with Model-Based Estimation
IEEE International Conference on Robotics and Automation(2022)
摘要
Collaborative localization is an essential capability for a team of robots such as connected vehicles to collaboratively estimate object locations from multiple perspectives with reliant cooperation. To enable collaborative localization, four key challenges must be addressed, including modeling complex relationships between observed objects, fusing observations from an arbitrary number of collaborating robots, quantifying localization uncertainty, and addressing latency of robot communications. In this paper, we introduce a novel approach that integrates uncertainty-aware spatiotemporal graph learning and model-based state estimation for a team of robots to collaboratively localize objects. Specifically, we introduce a new uncertainty-aware graph learning model that learns spatiotemporal graphs to represent historical motions of the objects observed by each robot over time and provides uncertainties in object localization. Moreover, we propose a novel method for integrated learning and model-based state estimation, which fuses asynchronous observations obtained from an arbitrary number of robots for collaborative localization. We evaluate our approach in two collaborative object localization scenarios in simulations and on real robots. Experimental results show that our approach outperforms previous methods and achieves state-of-the-art performance on asynchronous collaborative localization.
更多查看译文
关键词
object locations,observed objects,arbitrary number,collaborating robots,localization uncertainty,robot communications,uncertainty-aware spatiotemporal graph learning,model-based state estimation,uncertainty-aware graph learning model,learns spatiotemporal graphs,integrated learning,asynchronous observations,collaborative object localization scenarios,asynchronous collaborative localization,model-based estimation
AI 理解论文
溯源树
样例
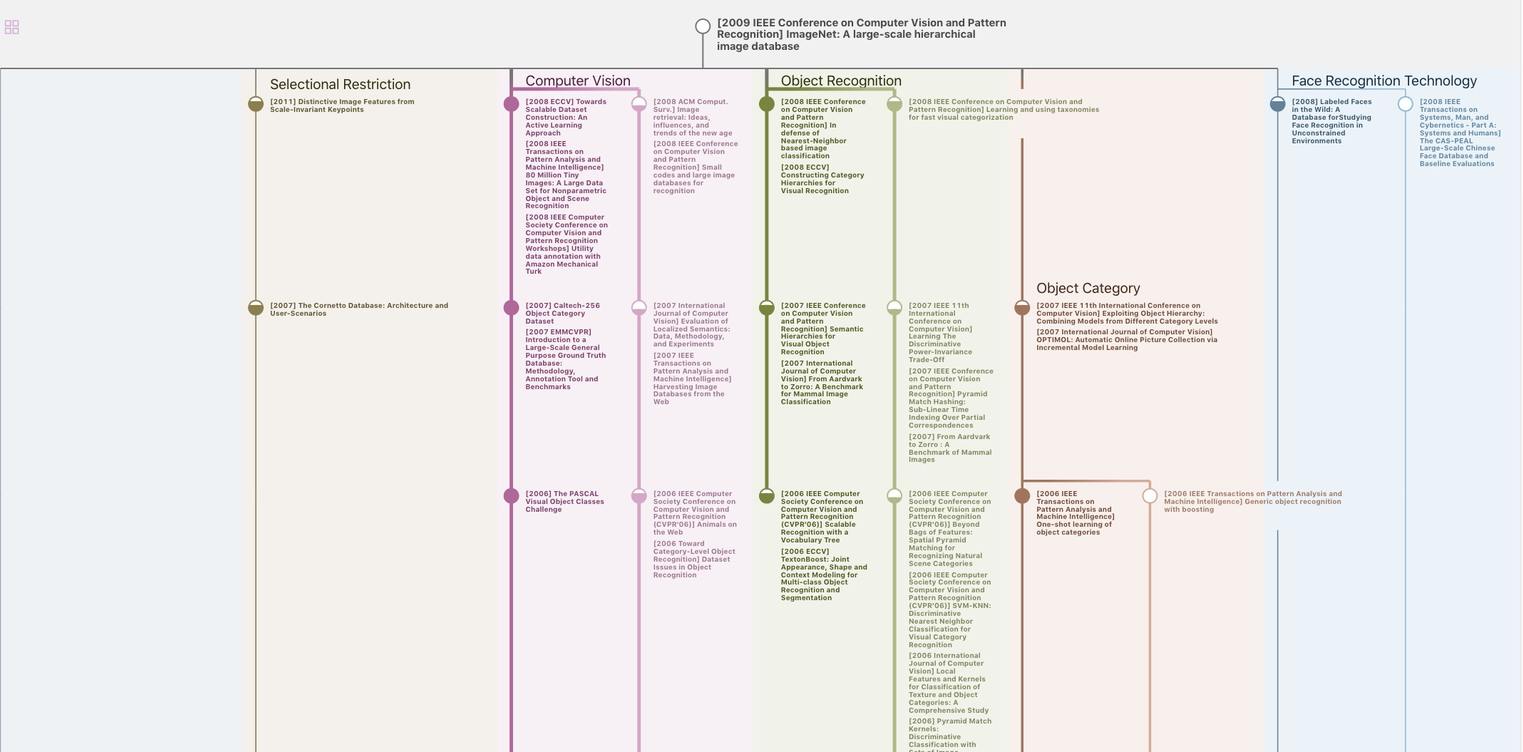
生成溯源树,研究论文发展脉络
Chat Paper
正在生成论文摘要