Generalized radiograph representation learning via cross-supervision between images and free-text radiology reports
NATURE MACHINE INTELLIGENCE(2022)
摘要
Pre-training lays the foundation for recent successes in radiograph analysis supported by deep learning. It learns transferable image representations by conducting large-scale fully- or self-supervised learning on a source domain; however, supervised pre-training requires a complex and labour-intensive two-stage human-assisted annotation process, whereas self-supervised learning cannot compete with the supervised paradigm. To tackle these issues, we propose a cross-supervised methodology called reviewing free-text reports for supervision (REFERS), which acquires free supervision signals from the original radiology reports accompanying the radiographs. The proposed approach employs a vision transformer and is designed to learn joint representations from multiple views within every patient study. REFERS outperforms its transfer learning and self-supervised learning counterparts on four well-known X-ray datasets under extremely limited supervision. Moreover, REFERS even surpasses methods based on a source domain of radiographs with human-assisted structured labels; it therefore has the potential to replace canonical pre-training methodologies.
更多查看译文
关键词
representation,images,cross-supervision,free-text
AI 理解论文
溯源树
样例
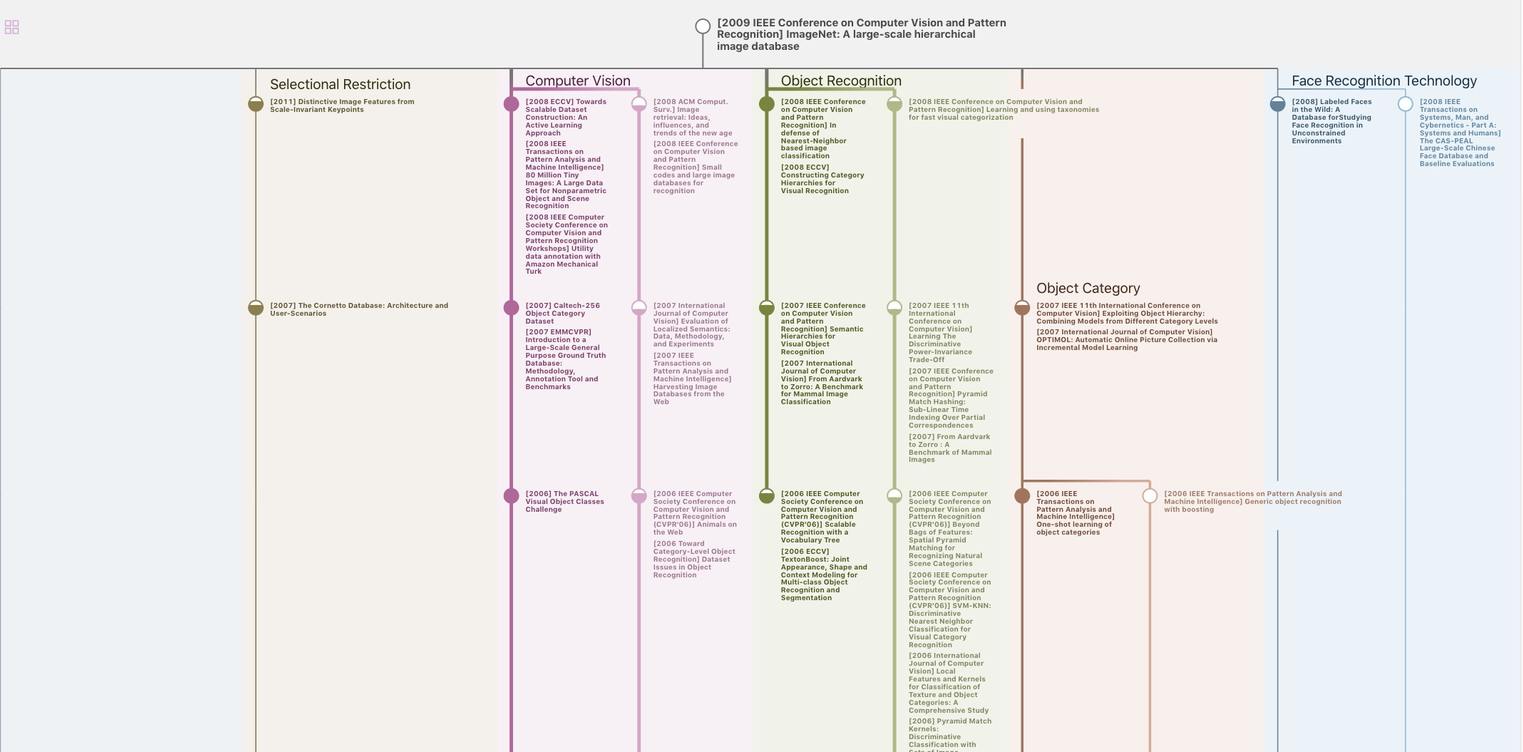
生成溯源树,研究论文发展脉络
Chat Paper
正在生成论文摘要