HEp-2 Cell Image Recognition with Transferable Cross-Dataset Synthetic Samples.
CAIP(2021)
摘要
The paper examines the possibilities of using synthetic HEp-2 cell images as a means of data augmentation. The common problem of biomedical datasets is the shortage of annotated samples required for the training of deep learning techniques. Traditional approaches based on image rotation and mirroring have their limitations, and alternative techniques based on generative adversarial networks (GANs) are currently being explored. Instead of looking solely at a single dataset or the creation of a recognition model with applicability for multiple datasets, this study focuses on the transferability of synthetic HEp-2 samples among publicly available datasets. The paper offers a workflow where the quality of synthetic samples is confirmed via an independent fine-tuned neural network. The subsequent combination of synthetic samples with original images outperforms traditional augmentation approaches and leads to state-of-the-art performance on both publicly available HEp-2 cell image datasets employed in this study.
更多查看译文
关键词
Transfer learning, Generative adversarial network, Deep learning, DCGAN, HEp-2 cell images
AI 理解论文
溯源树
样例
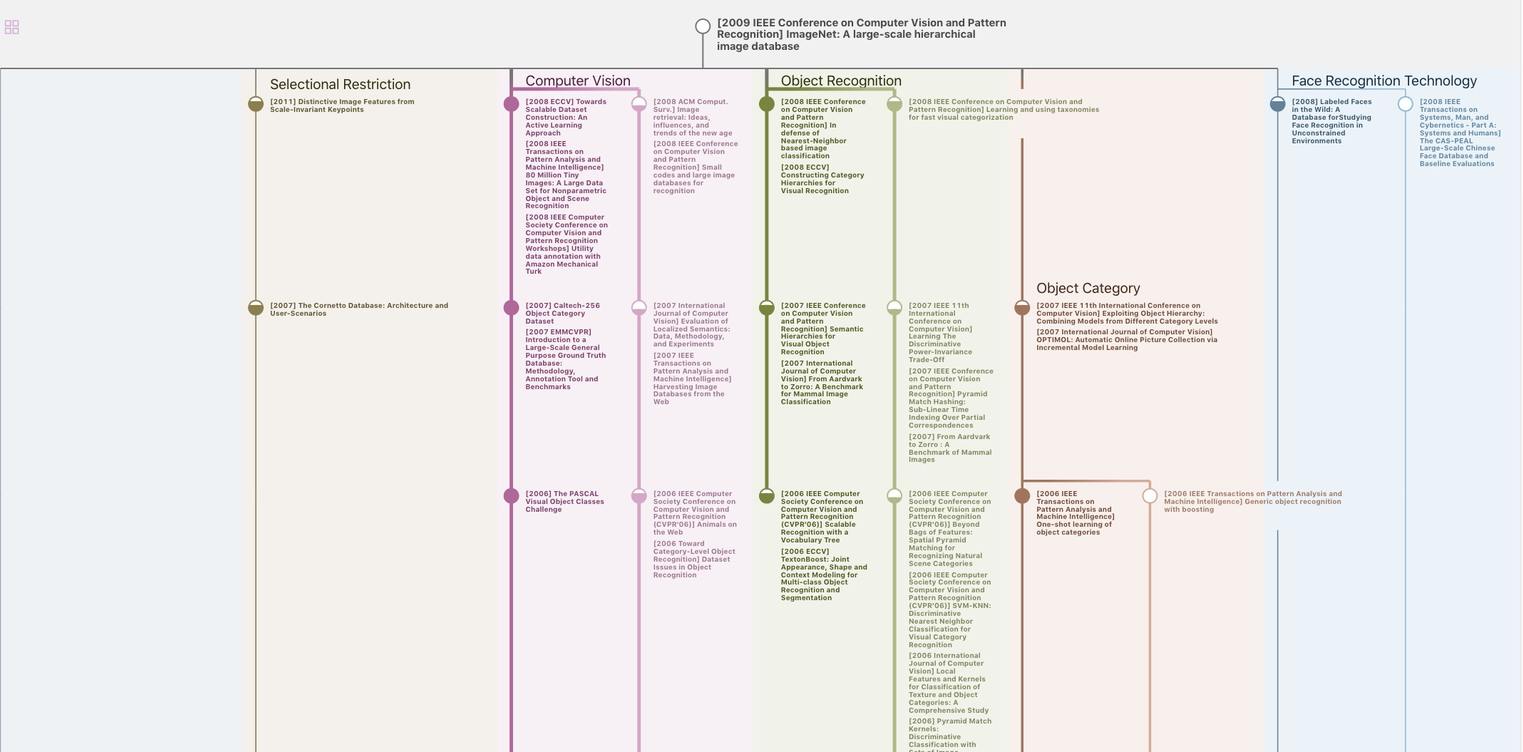
生成溯源树,研究论文发展脉络
Chat Paper
正在生成论文摘要