Drone-based Generation of Sensor Reference and Training Data for Highly Automated Vehicles.
ITSC(2021)
摘要
Highly automated vehicles require complex sensor systems to accurately perceive their environment and safely reach a destination. To achieve the necessary perception quality, these sensor systems typically consist of a combination of several sensor types. Due to the resulting complexity in hardware and software, it is necessary to use large amounts of highly accurate reference data for evaluation. Previous approaches to generate these data (e.g. RTK-GNSS-INS) are limited regarding the applicability in real traffic or the achievable accuracy. We present a drone-based approach to circumvent these problems. A drone follows a sensor system under test on a test vehicle in real traffic and captures its surroundings using a camera. After spatio-temporal synchronization, reference data can be generated from the camera imagery using deep learning-based object detection and tracking. We show that this approach and the quality of the derived data not only allows the evaluation of a LiDAR-based road user detection system, but can also be used to improve the system if used as training data. Within the scope of the conducted experiments, the average precision for cars was improved by 30 percentage points, which resulted in the false negative rate reduction by more than 60% for mid-range distances on a selected route.
更多查看译文
关键词
drone-based generation,sensor reference,training data,highly automated vehicles,complex sensor systems,necessary perception quality,sensor system,sensor types,resulting complexity,highly accurate reference data,RTK-GNSS-INS,achievable accuracy,drone-based approach,deep learning-based object detection,tracking,derived data,LiDAR-based road user detection system
AI 理解论文
溯源树
样例
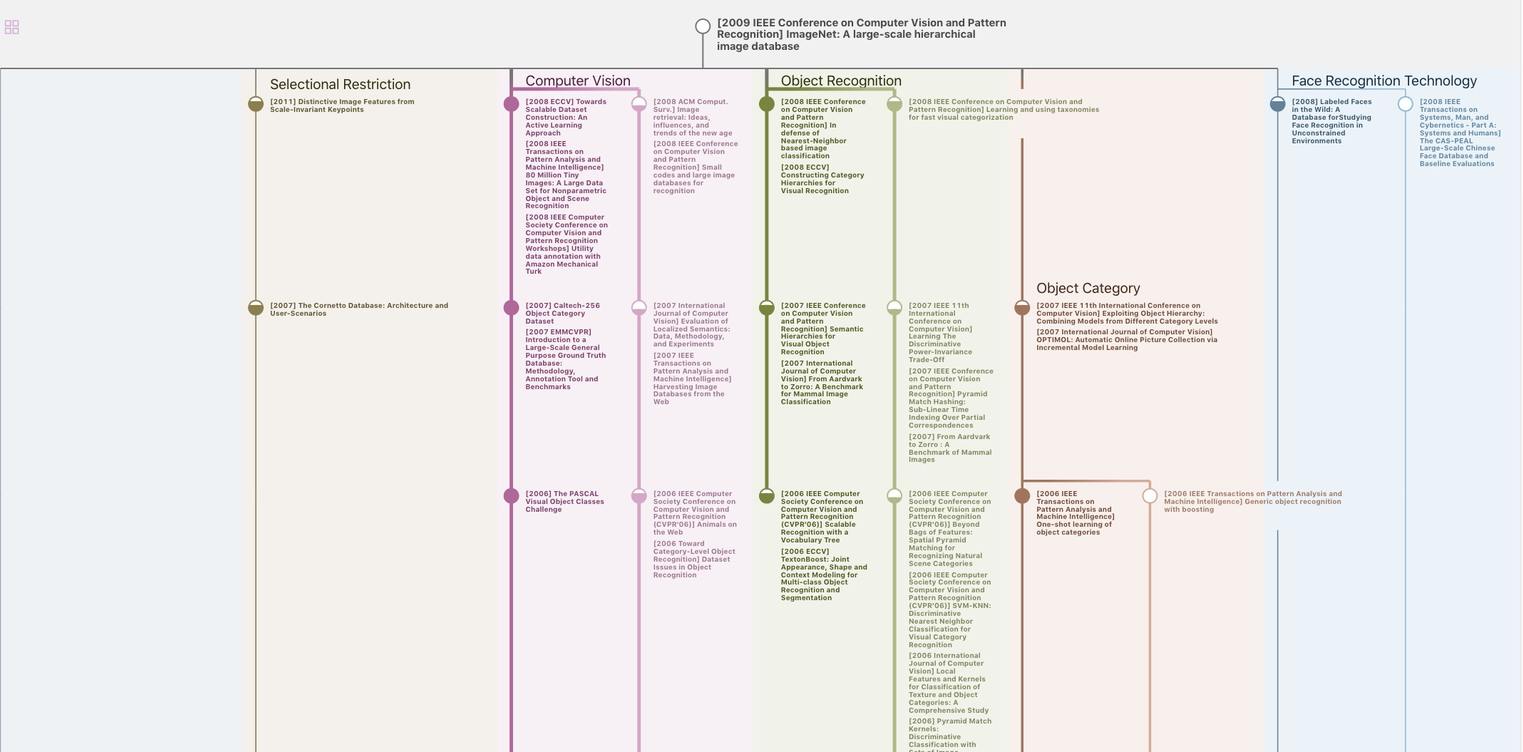
生成溯源树,研究论文发展脉络
Chat Paper
正在生成论文摘要