Parameter Sharing Reinforcement Learning for Modeling Multi-Agent Driving Behavior in Roundabout Scenarios.
ITSC(2021)
摘要
Modeling other drivers' behavior in highly interactive traffic situations, such as roundabouts, is a challenging task. We address this task using a Multi-Agent Reinforcement Learning (MARL) approach that learns a driving policy based on a minimal set of assumptions: drivers want to move forward and avoid collisions while maintaining low accelerations. Each agent's actions depend only on his observation of the local environment; no explicit communication between agents is possible. In order to teach the agents to safely interact with each other, and for example, respect right-of-way rules, we use parameter sharing: During training all vehicles are controlled by the same policy and the aggregated experiences are used to improve the policy. Moreover, parameter sharing enables us to use the efficient Soft Actor Critic (SAC) algorithm for training. The approach is evaluated in a roundabout setting with different traffic densities. Furthermore, the ability of the model to generalize is assessed in an untrained roundabout. In both settings, success rates above 97% demonstrate that a safe and transferable policy is learned.
更多查看译文
关键词
multiagent reinforcement learning,driving policy,explicit communication,soft actor critic,traffic densities,untrained roundabout,safe policy,transferable policy,multiagent driving behavior,parameter sharing,interactive traffic situations,driver behavior,MARL,collision avoidance,accelerations,right-of-way rules,vehicles,SAC
AI 理解论文
溯源树
样例
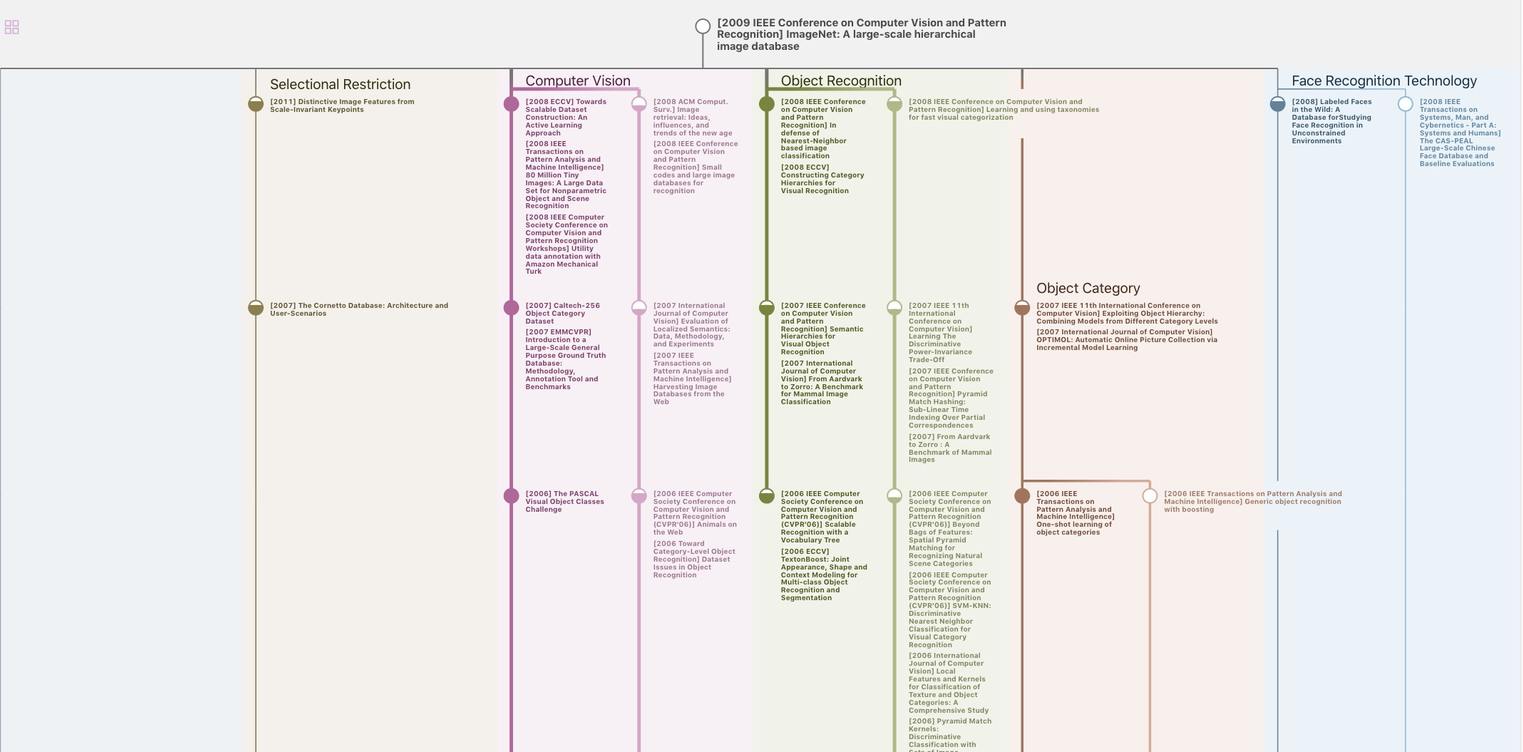
生成溯源树,研究论文发展脉络
Chat Paper
正在生成论文摘要