Learning policy scheduling for text augmentation
Neural Networks(2022)
摘要
When training deep learning models, data augmentation is an important technique to improve the performance and alleviate overfitting. In natural language processing (NLP), existing augmentation methods often use fixed strategies. However, it might be preferred to use different augmentation policies in different stage of training, and different datasets may require different augmentation policies. In this paper, we take dynamic policy scheduling into consideration. We design a search space over augmentation policies by integrating several common augmentation operations. Then, we adopt a population based training method to search the best augmentation schedule. We conduct extensive experiments on five text classification and two machine translation tasks. The results show that the optimized dynamic augmentation schedules achieve significant improvements against previous methods.
更多查看译文
关键词
Data augmentation,Text classification
AI 理解论文
溯源树
样例
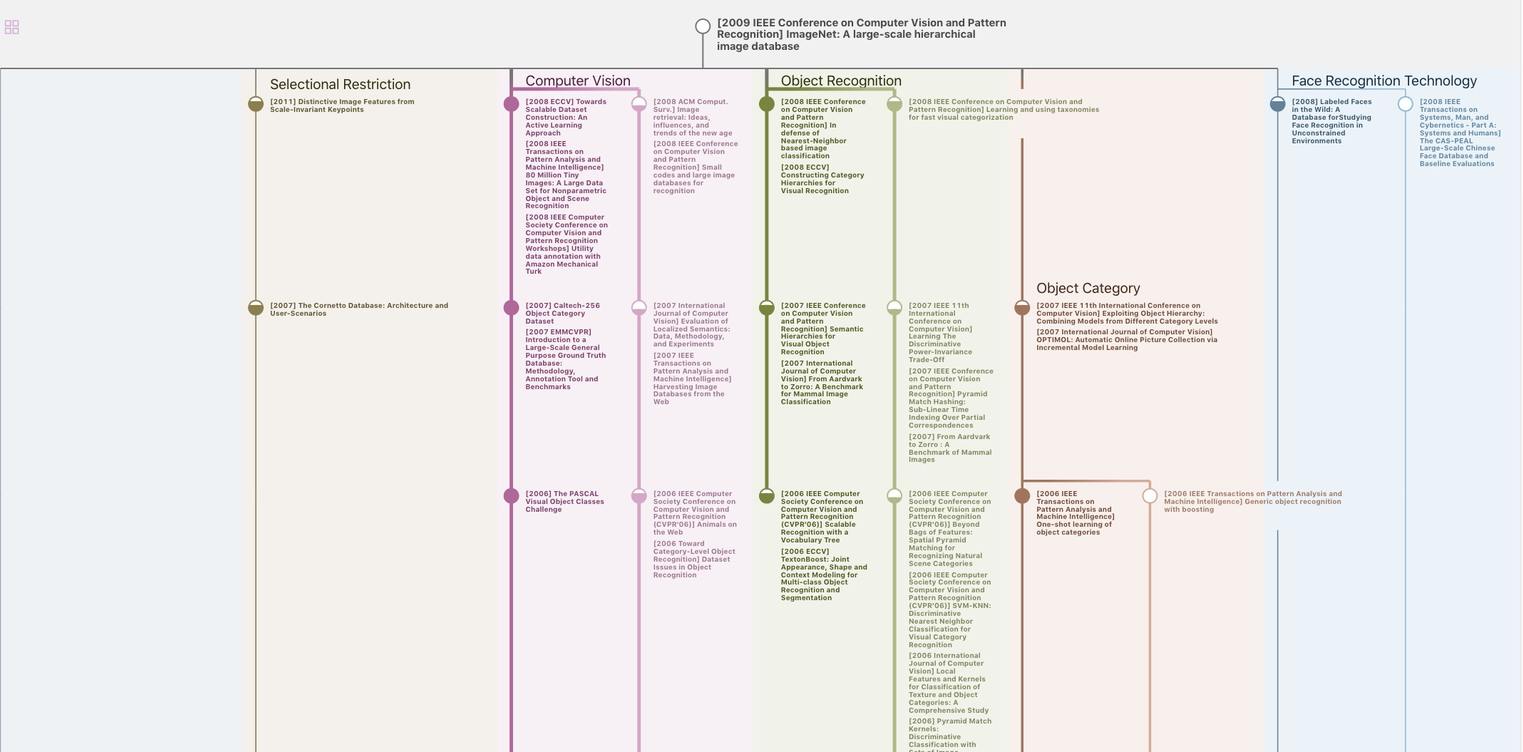
生成溯源树,研究论文发展脉络
Chat Paper
正在生成论文摘要