Data augmentation based on dynamical systems for the classification of brain states
bioRxiv (Cold Spring Harbor Laboratory)(2020)
摘要
The application of machine learning algorithms to neuroimaging data shows great promise for the classification of physiological and pathological brain states. However, classifiers trained on high dimensional data are prone to overfitting, especially for a low number of training samples. We describe the use of whole-brain computational models for data augmentation in brain state classification. Our low dimensional model is based on nonlinear oscillators coupled by the empirical SC of the brain. We use this model to enhance a dataset consisting of functional magnetic resonance imaging recordings acquired during all stages of the human wake-sleep cycle. After fitting the model to the average FC of each state, we show that the synthetic data generated by the model yields classification accuracies comparable to those obtained from the empirical data. We also show that models fitted to individual subjects generate surrogates with enough information to train classifiers that present significant transfer learning accuracy to the whole sample. Whole-brain computational modeling represents a useful tool to produce large synthetic datasets for data augmentation in the classification of certain brain states, with potential applications to computer-assisted diagnosis and prognosis of neuropsychiatric disorders.
更多查看译文
关键词
brain states,classification,dynamical systems,data
AI 理解论文
溯源树
样例
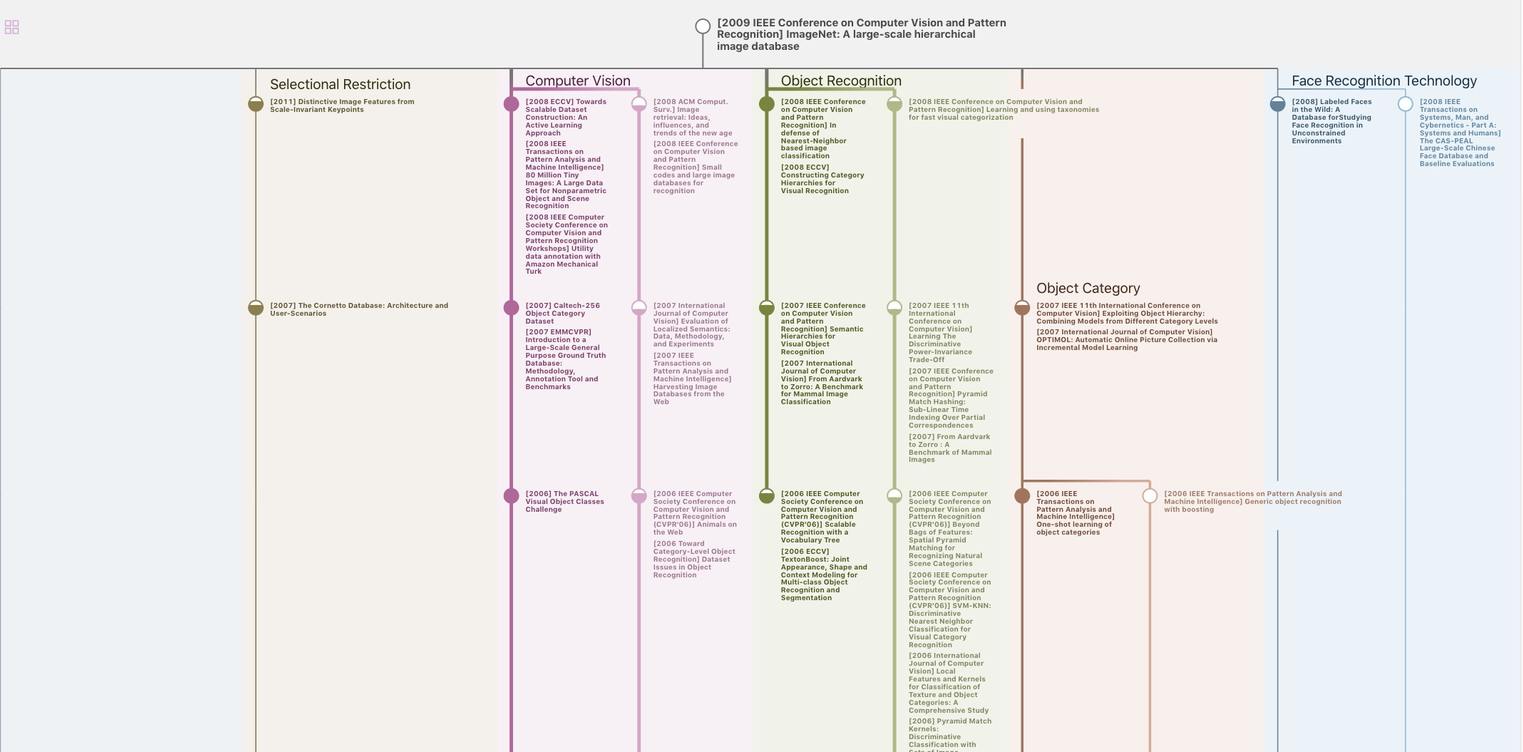
生成溯源树,研究论文发展脉络
Chat Paper
正在生成论文摘要