A Q-learning based SPS Resource Scheduling Algorithm for Reliable C-V2X Communication.
ICDSP(2021)
摘要
In 5G networks, the Ultra Reliable Low Latency Communication (URLLC) scenario is studied specifically to support the Cellular-Vehicle-to-Everything (C-V2X) communication. In Mode 4 of the Third Generation Partnership Project (3GPP), the vehicles are enabled to transmit the messages in the decentralized mode without a central network controlling the radio resources by using the Semi-Persistent Scheduling (SPS) algorithm. In SPS, the channel sensing information is used for the resource reservation process by the vehicle itself in order to achieve a fast access to the vehicle network. However, the conventional SPS with a fixed reservation process would lead to a high collision probability of the packets in the case of high traffic density. Based on the study of impacts of SPS with reservation probabilities to both collision ratio and delay through simulations, a Q-learning based SPS (Q-SPS) algorithm is proposed in order to make an intelligent decision on the reservation probability with a reward feedback corresponding to the dynamic change of C-V2X network environment. The simulation results show that the update delay can be reduced by 32% while the packet reception rate is maintained at 95%, when compared with the conventional SPS algorithm in high traffic density.
更多查看译文
AI 理解论文
溯源树
样例
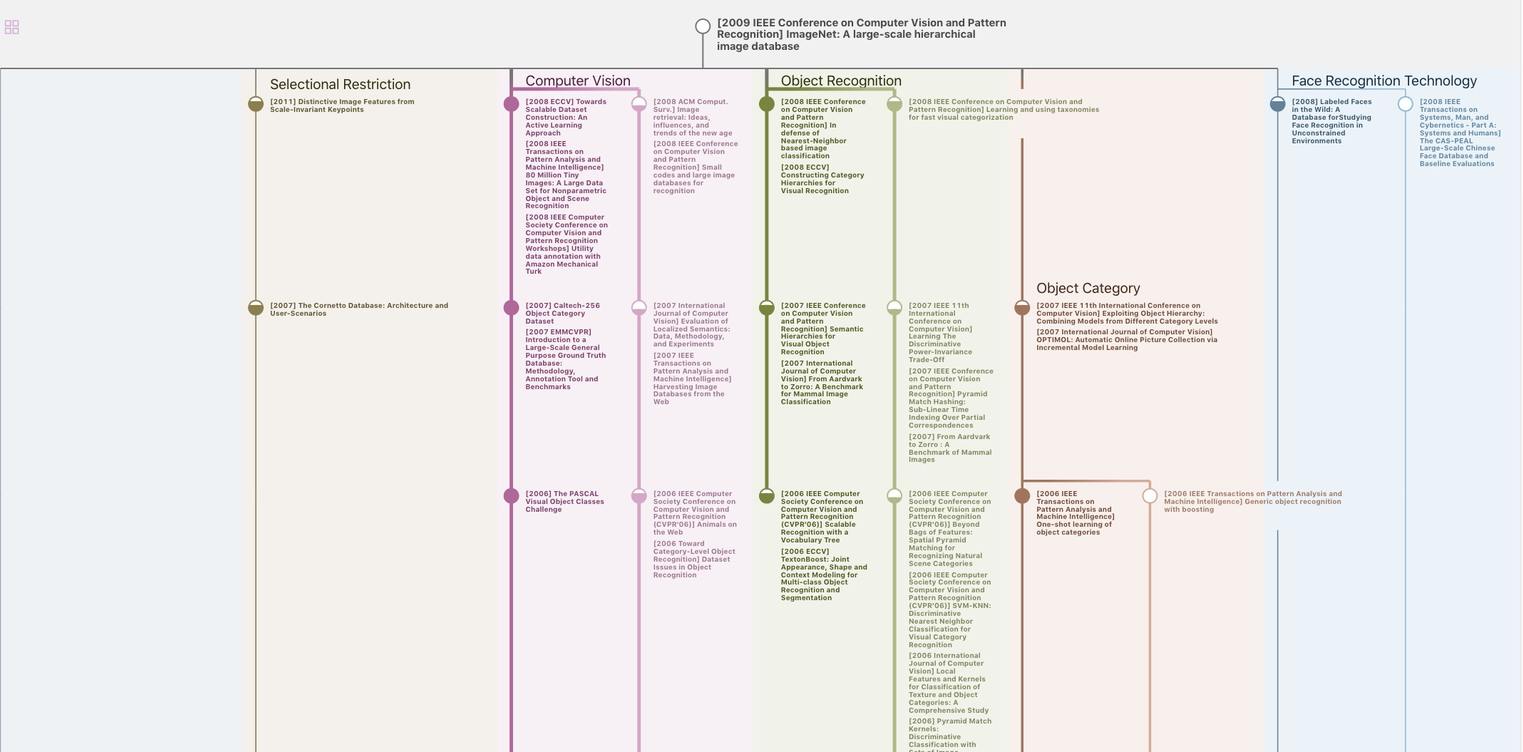
生成溯源树,研究论文发展脉络
Chat Paper
正在生成论文摘要