Signal Denoising Using 1D Convolutional Neural Network for Compressed Sensing SAR Imaging Improvement.
ICDSP(2021)
摘要
Compressed sensing (CS) is currently utilized in the application of Synthetic Aperture Radar (SAR) imaging. Unfortunately, the CS SAR imaging performance degrades as SNR decreases. However, there exists a denoising convolutional neural network (DnCNN) based on 2D convolution (DnCNN-2D) in the field of image denoising. Traditional DnCNN-2D is not directly applicable to SAR imaging. Thus, a DnCNN based on 1D convolution (DnCNN-1D) for signal denoising is proposed and employed to denoise the SAR signal. The denoised SAR signal is subsequently utilized for CS SAR imaging. Each convolutional layer of DnCNN-1D has a fixed number and fixed size of filters and activation functions. The residual learning strategy and batch normalization are adopted in the DnCNN-1D. The segmental signal-to-noise ratio (SEGSNR) and the normalized mean square error (NMSE) are selected to evaluate signal denoising and SAR imaging performance, respectively. The experimental results indicate that imaging performance is significantly improved after denoising the SAR signal by the proposed method.
更多查看译文
AI 理解论文
溯源树
样例
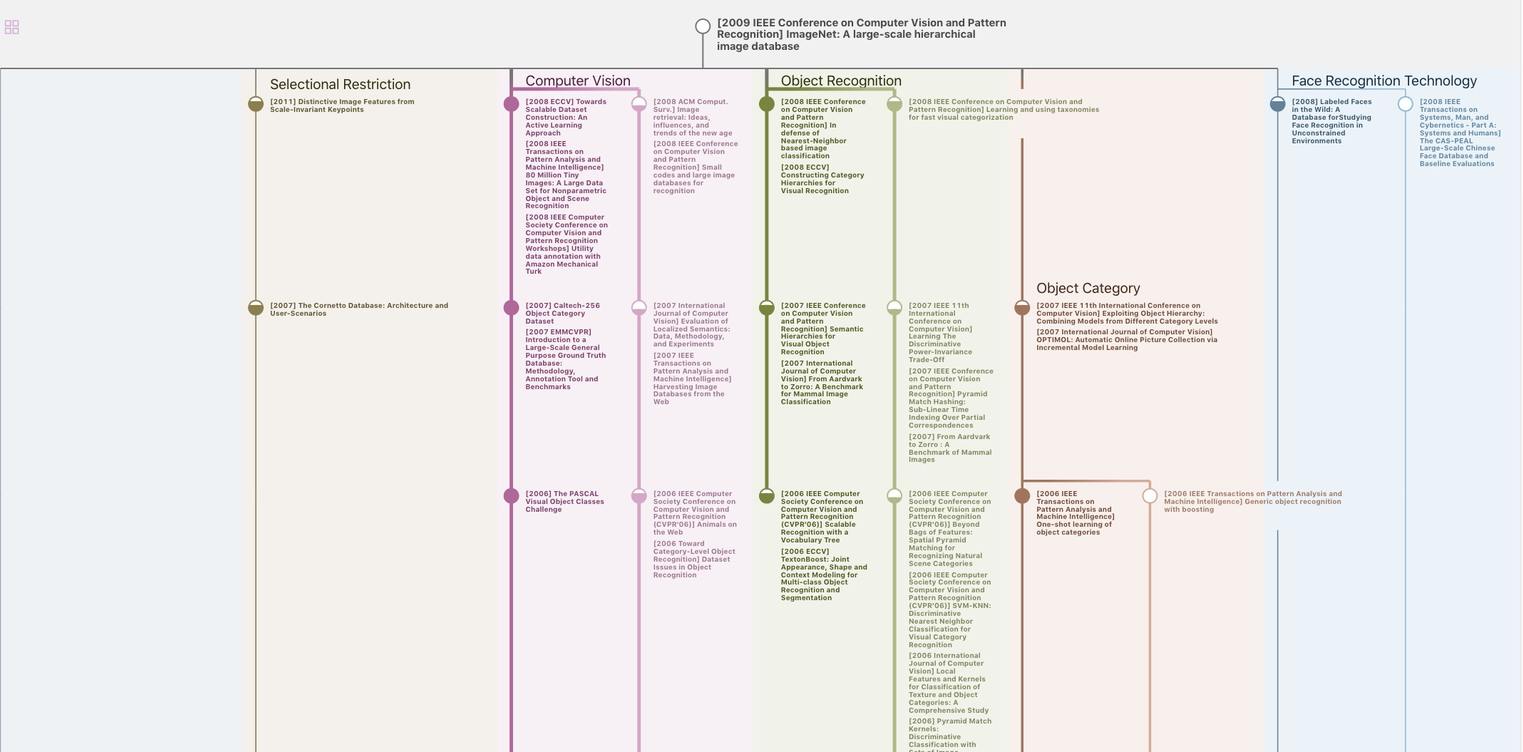
生成溯源树,研究论文发展脉络
Chat Paper
正在生成论文摘要