Mining Software Entities in Scientific Literature: Document-level NER for an Extremely Imbalance and Large-scale Task
Conference on Information and Knowledge Management(2021)
摘要
ABSTRACTWe present a comprehensive information extraction system dedicated to software entities in scientific literature. This task combines the complexity of automatic reading of scientific documents (PDF processing, document structuring, styled/rich text, scaling) with challenges specific to mining software entities: high heterogeneity and extreme sparsity of mentions, document-level cross-references, disambiguation of noisy software mentions and poor portability of Machine Learning approaches between highly specialized domains. While NER is a key component to recognize new and unseen software, considering this task as a simple NER application fails to address most of these issues. In this paper, we propose a multi-model Machine Learning approach where raw documents are ingested by a cascade of document structuring processes applied not to text, but to layout token elements. The cascading process further enriches the relevant structures of the document with a Deep Learning software mention recognizer adapted to the high sparsity of mentions. The Machine Learning cascade culminates with entity disambiguation to alleviate false positives and to provide software entity linking. A bibliographical reference resolution is integrated to the process for attaching references cited alongside the software mentions. Based on the first gold-standard annotated dataset developed for software mentions, this work establishes a new reference end-to-end performance for this task. Experiments with the CORD-19 publications have further demonstrated that our system provides practically usable performance and is scalable to the whole scientific corpus, enabling novel applications for crediting research software and for better understanding the impact of software in science.
更多查看译文
AI 理解论文
溯源树
样例
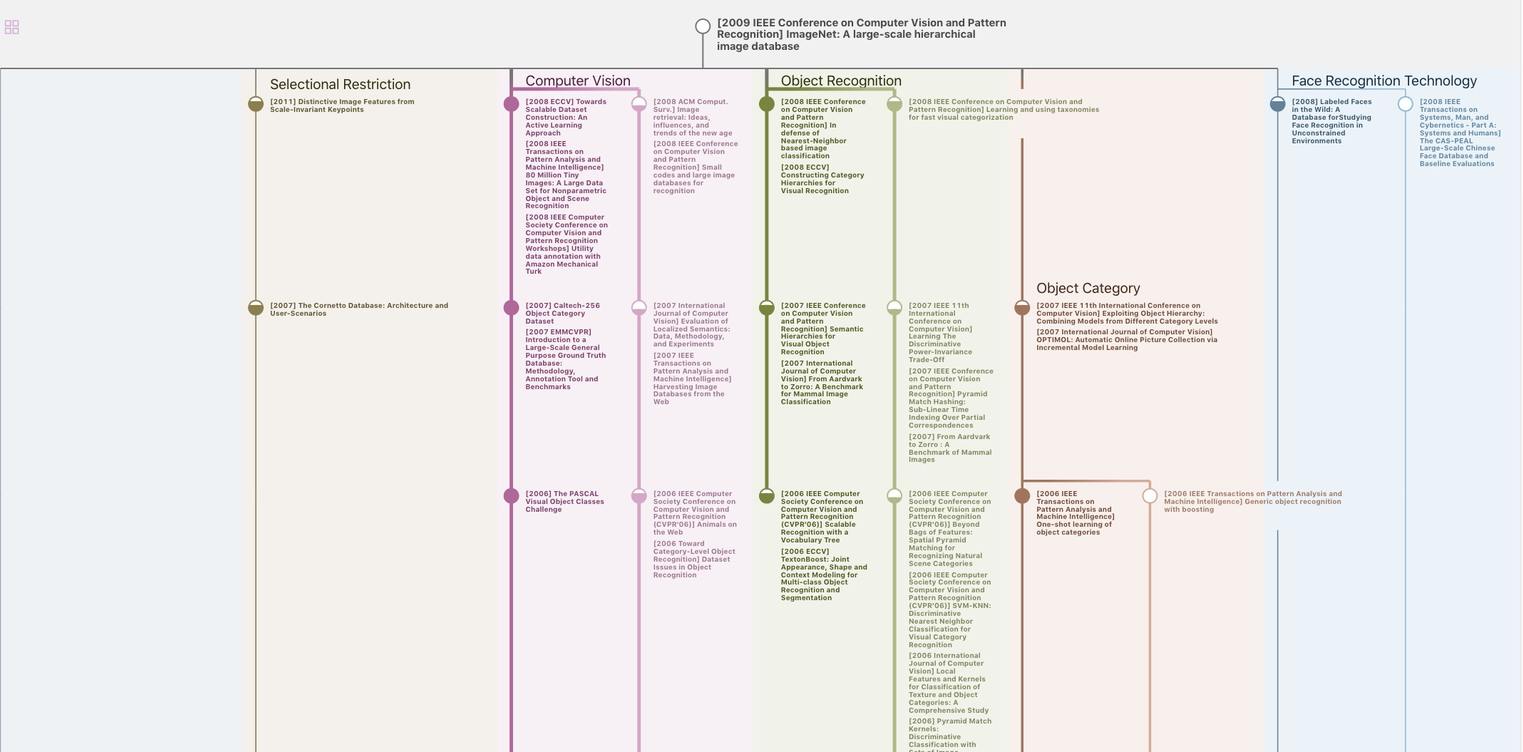
生成溯源树,研究论文发展脉络
Chat Paper
正在生成论文摘要