Popcorn: Human-in-the-loop Popularity Debiasing in Conversational Recommender Systems
Conference on Information and Knowledge Management(2021)
摘要
ABSTRACTRecent conversational recommender systems (CRS) provide a promising solution to accurately capture a user's preferences by communicating with users in natural language to interactively guide them while pro-actively eliciting their current interests. Previous research on this mainly focused on either learning a supervised model with semantic features extracted from the user's responses, or training a policy network to control the dialogue state. However, none of them has considered the issue of popularity bias in a CRS. This paper proposes a human-in-the-loop popularity debiasing framework that integrates real-time semantic understanding of open-ended user utterances as well as historical records, while also effectively managing the dialogue with the user. This allows the CRS to balance the recommendation performance as well as the item popularity so as to avoid the well-known "long-tail'' effect. We demonstrate the effectiveness of our approach via experiments on two conversational recommendation datasets, and the results confirm that our proposed approach achieves high-accuracy recommendation while mitigating popularity bias.
更多查看译文
AI 理解论文
溯源树
样例
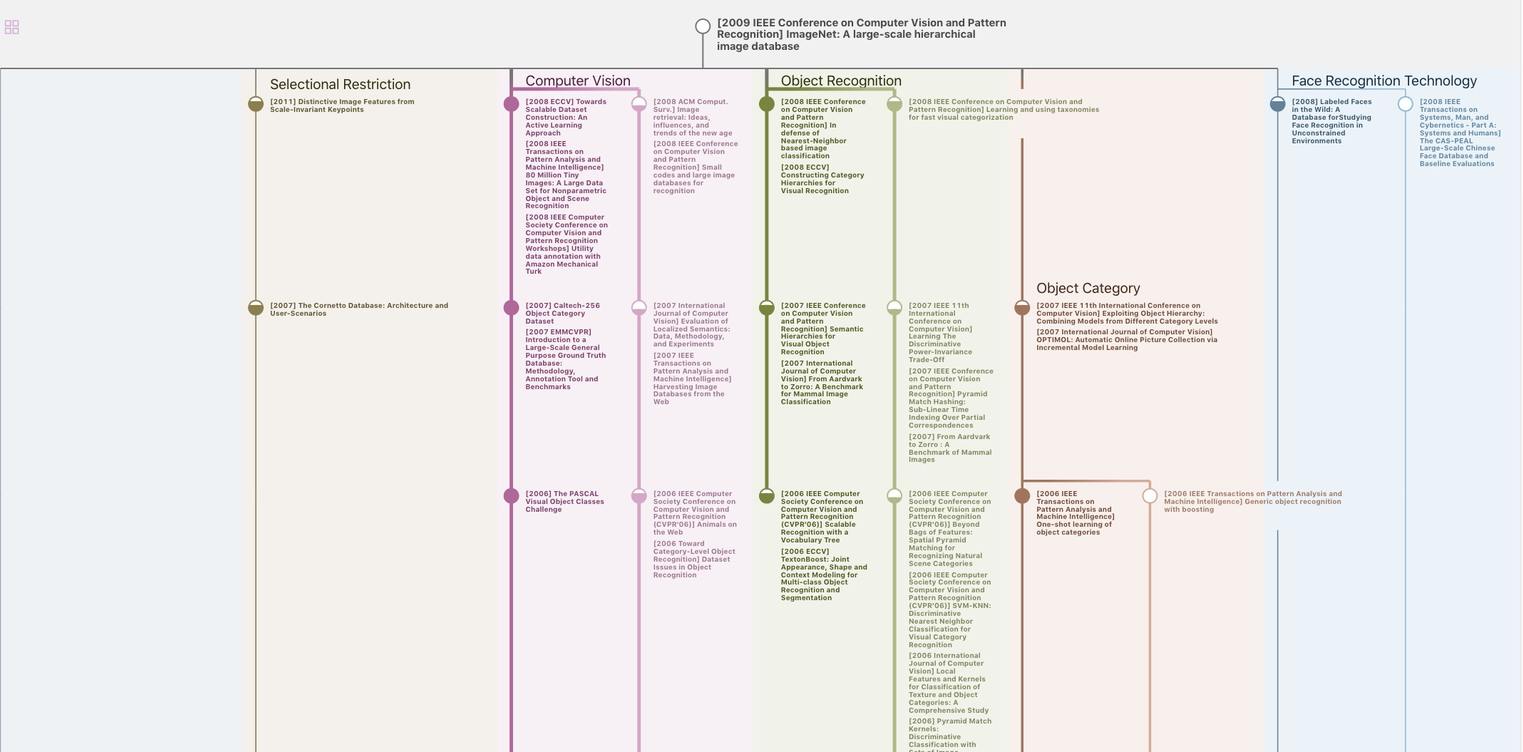
生成溯源树,研究论文发展脉络
Chat Paper
正在生成论文摘要