Spatiotemporal variable selection and air quality impact assessment of COVID-19 lockdown
Spatial Statistics(2022)
摘要
During the first wave of the COVID-19 pandemics in 2020, lockdown policies reduced human mobility in many countries globally. This significantly reduces car traffic-related emissions. In this paper, we consider the impact of the Italian restrictions (lockdown) on the air quality in the Lombardy Region. In particular, we consider public data on concentrations of particulate matters (PM10 and PM2.5) and nitrogen dioxide, pre/during/after lockdown. To reduce the effect of confounders, we use detailed regression function based on meteorological, land and calendar information. Spatial and temporal correlations are handled using a multivariate spatiotemporal model in the class of hidden dynamic geostatistical models (HDGM). Due to the large size of the design matrix, variable selection is made using a hybrid approach coupling the well known LASSO algorithm with the cross-validation performance of HDGM.
更多查看译文
关键词
Multivariate spatiotemporal models,Model selection,Particulate matters,Nitrogen dioxide,COVID-19
AI 理解论文
溯源树
样例
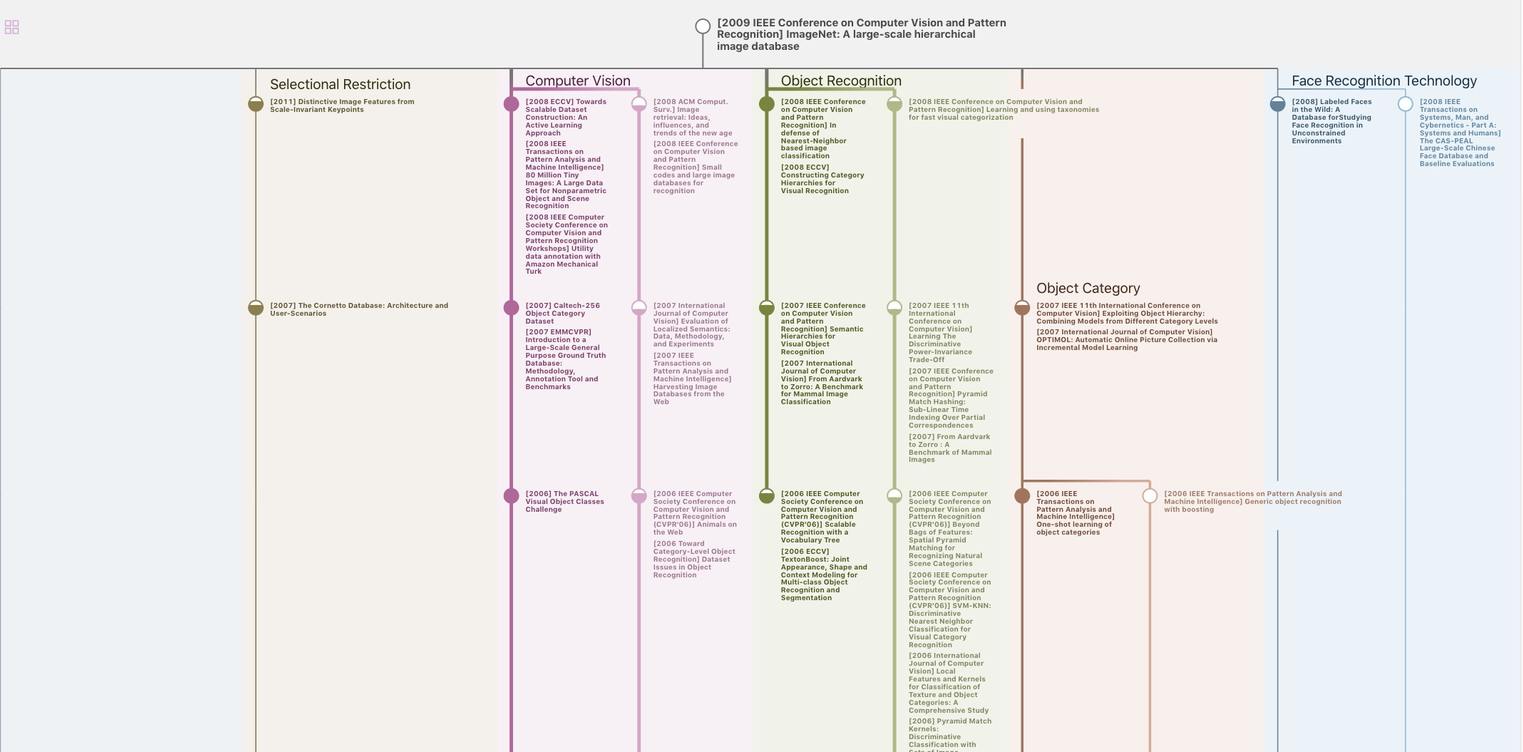
生成溯源树,研究论文发展脉络
Chat Paper
正在生成论文摘要