A Unified Approach to Coreset Learning.
IEEE transactions on neural networks and learning systems(2022)
摘要
Coreset of a given dataset and loss function is usually a small weighed set that approximates this loss for every query from a given set of queries. Coresets have shown to be very useful in many applications. However, coresets' construction is done in a problem-dependent manner and it could take years to design and prove the correctness of a coreset for a specific family of queries. This could limit coresets' use in practical applications. Moreover, small coresets provably do not exist for many problems. To address these limitations, we propose a generic, learning-based algorithm for construction of coresets. Our approach offers a new definition of coreset, which is a natural relaxation of the standard definition and aims at approximating the average loss of the original data over the queries. This allows us to use a learning paradigm to compute a small coreset of a given set of inputs with respect to a given loss function using a training set of queries. We derive formal guarantees for the proposed approach. Experimental evaluation on deep networks and classic machine learning problems show that our learned coresets yield comparable or even better results than the existing algorithms with worst case theoretical guarantees (that may be too pessimistic in practice). Furthermore, our approach applied to deep network pruning provides the first coreset for a full deep network, i.e., compresses all the networks at once, and not layer by layer or similar divide-and-conquer methods.
更多查看译文
关键词
Training,Standards,Q measurement,Machine learning algorithms,Computational modeling,Weight measurement,Support vector machines,Coresets,data summarization,generalization,learning
AI 理解论文
溯源树
样例
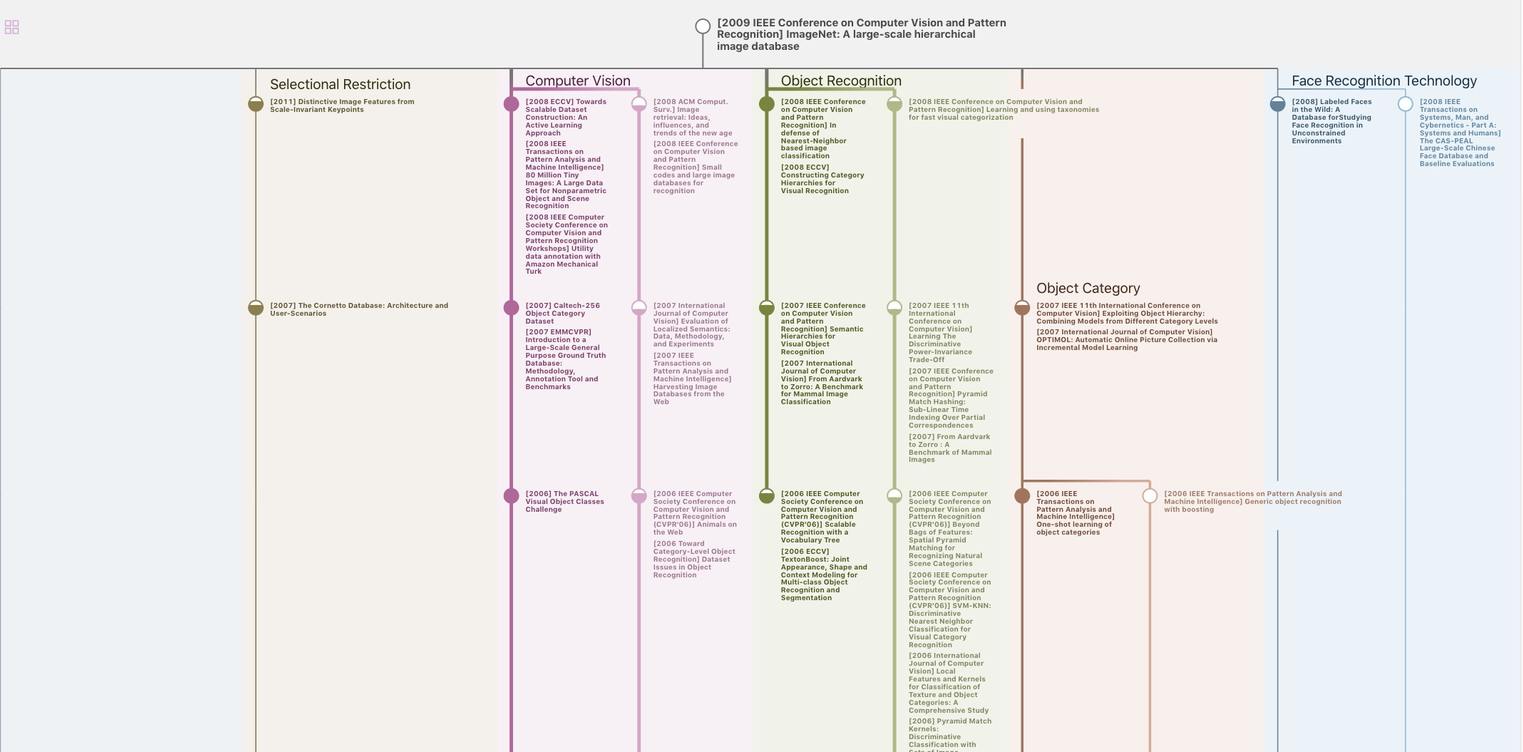
生成溯源树,研究论文发展脉络
Chat Paper
正在生成论文摘要