Stable and compact face recognition via unlabeled data driven sparse representation-based classification
Signal Processing: Image Communication(2023)
摘要
Sparse representation-based classification (SRC) has attracted much attention by casting the recognition problem as simple linear regression problem. SRC methods, however, still is limited to enough labeled samples per category, insufficient use of unlabeled samples, and instability of representation. For tackling these problems, an unlabeled data driven inverse projection pseudo-full-space representation-based classification model is proposed with low-rank sparse constraints. The proposed model aims to mine the hidden semantic information and intrinsic structure information of all available data, which is suitable for few labeled samples and proportion imbalance between labeled samples and unlabeled samples problems in frontal face recognition. The mixed Gauss–Seidel and Jacobian ADMM algorithm is introduced to solve the model. The convergence, representation capability and stability of the model are analyzed. Experiments on three public datasets show that the proposed LR-S-PFSRC model achieves stable results, especially for proportion imbalance of samples.
更多查看译文
关键词
Sparse representation-based classification,Unlabeled data,Sparsity,Low-rank subspace structure,Face recognition
AI 理解论文
溯源树
样例
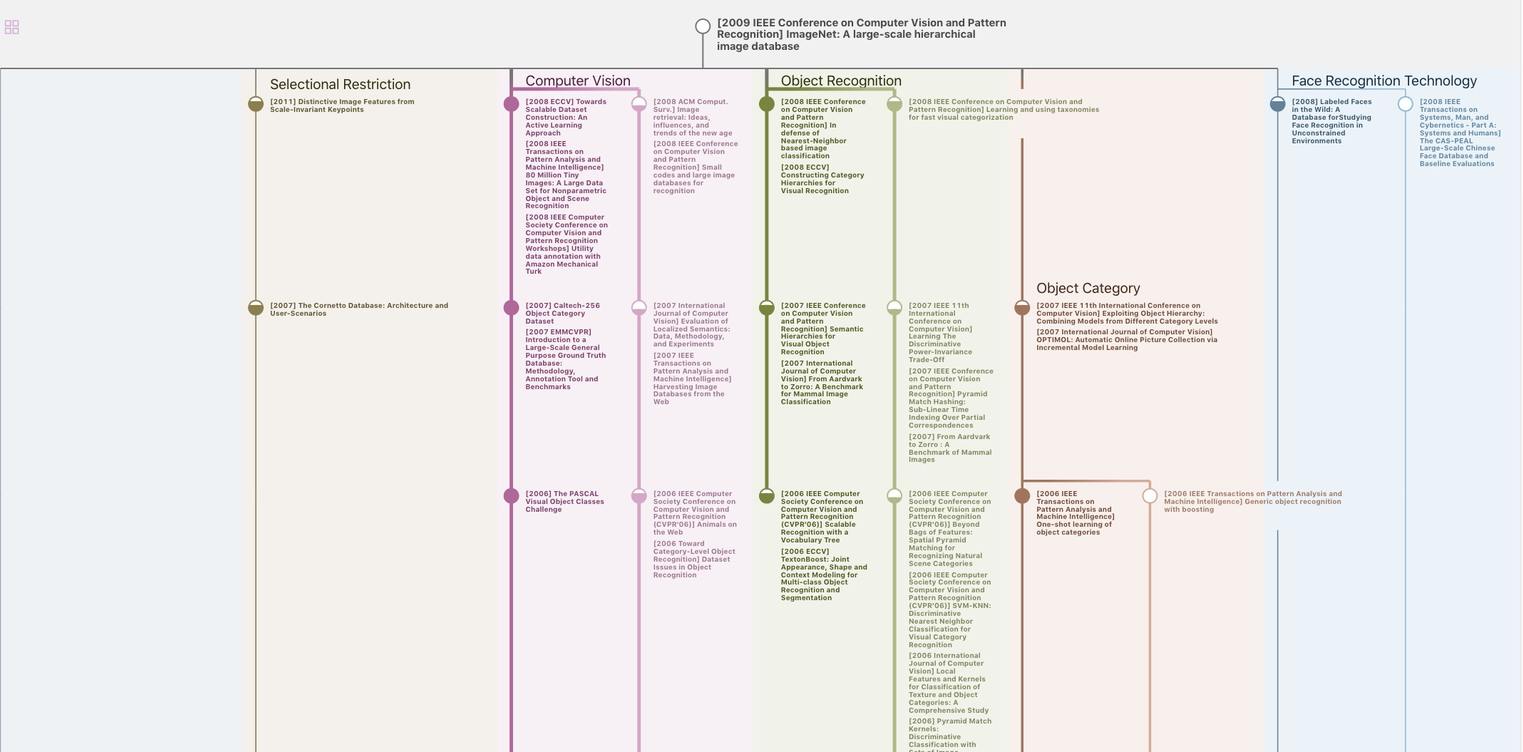
生成溯源树,研究论文发展脉络
Chat Paper
正在生成论文摘要