Bayesian U-Net: Estimating Uncertainty in Semantic Segmentation of Earth Observation Images
REMOTE SENSING(2021)
摘要
In recent years, numerous deep learning techniques have been proposed to tackle the semantic segmentation of aerial and satellite images, increase trust in the leaderboards of main scientific contests and represent the current state-of-the-art. Nevertheless, despite their promising results, these state-of-the-art techniques are still unable to provide results with the level of accuracy sought in real applications, i.e., in operational settings. Thus, it is mandatory to qualify these segmentation results and estimate the uncertainty brought about by a deep network. In this work, we address uncertainty estimations in semantic segmentation. To do this, we relied on a Bayesian deep learning method, based on Monte Carlo Dropout, which allows us to derive uncertainty metrics along with the semantic segmentation. Built on the most widespread U-Net architecture, our model achieves semantic segmentation with high accuracy on several state-of-the-art datasets. More importantly, uncertainty maps are also derived from our model. While they allow for the performance of a sounder qualitative evaluation of the segmentation results, they also include valuable information to improve the reference databases.
更多查看译文
关键词
deep neural network, semantic segmentation, bayesian network, optical imagery, uncertainty estimation
AI 理解论文
溯源树
样例
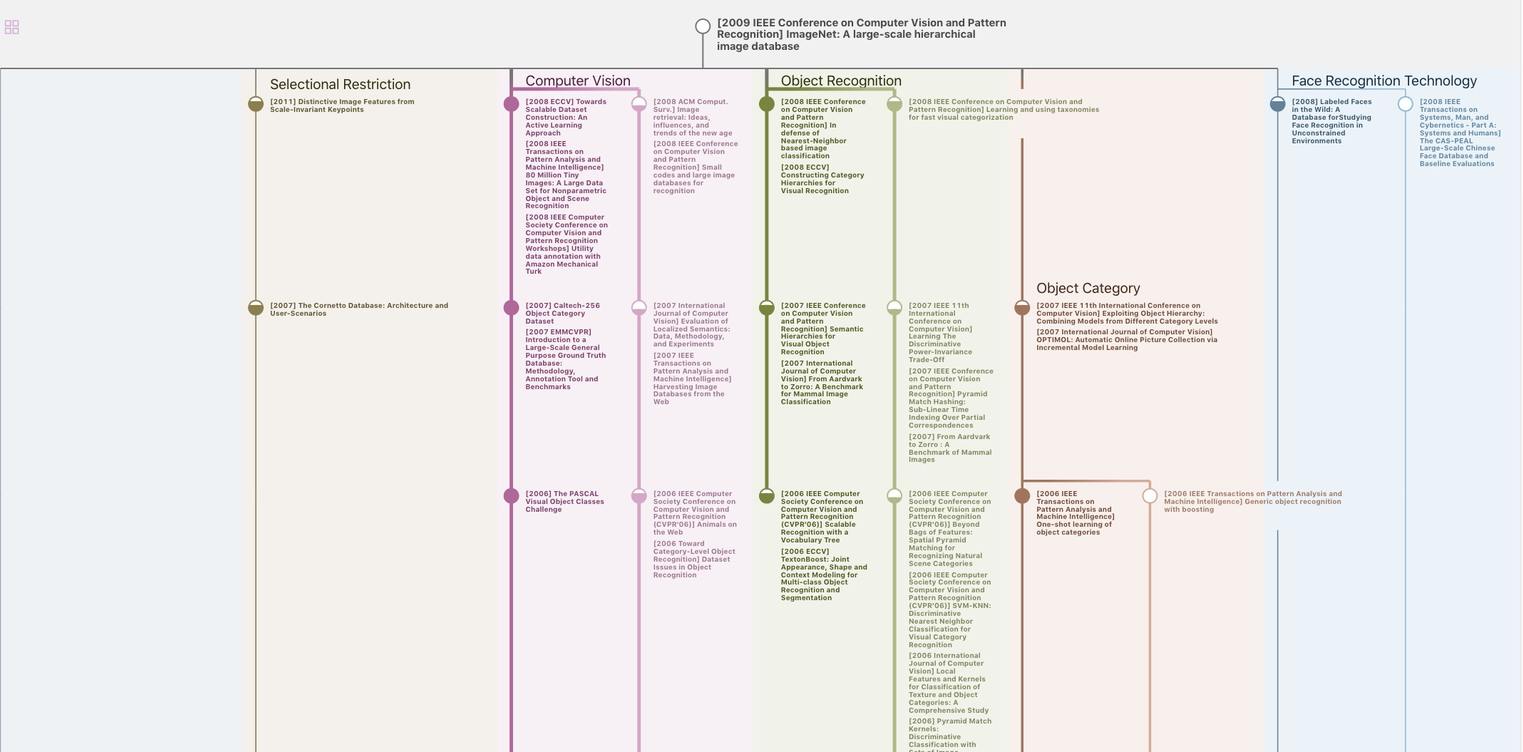
生成溯源树,研究论文发展脉络
Chat Paper
正在生成论文摘要