SVDNVLDA: predicting lncRNA-disease associations by Singular Value Decomposition and node2vec
BMC BIOINFORMATICS(2021)
摘要
Background Numerous studies on discovering the roles of long non-coding RNAs (lncRNAs) in the occurrence, development and prognosis progresses of various human diseases have drawn substantial attentions. Since only a tiny portion of lncRNA-disease associations have been properly annotated, an increasing number of computational methods have been proposed for predicting potential lncRNA-disease associations. However, traditional predicting models lack the ability to precisely extract features of biomolecules, it is urgent to find a model which can identify potential lncRNA-disease associations with both efficiency and accuracy. Results In this study, we proposed a novel model, SVDNVLDA, which gained the linear and non-linear features of lncRNAs and diseases with Singular Value Decomposition (SVD) and node2vec methods respectively. The integrated features were constructed from connecting the linear and non-linear features of each entity, which could effectively enhance the semantics contained in ultimate representations. And an XGBoost classifier was employed for identifying potential lncRNA-disease associations eventually. Conclusions We propose a novel model to predict lncRNA-disease associations. This model is expected to identify potential relationships between lncRNAs and diseases and further explore the disease mechanisms at the lncRNA molecular level.
更多查看译文
关键词
node2vec
AI 理解论文
溯源树
样例
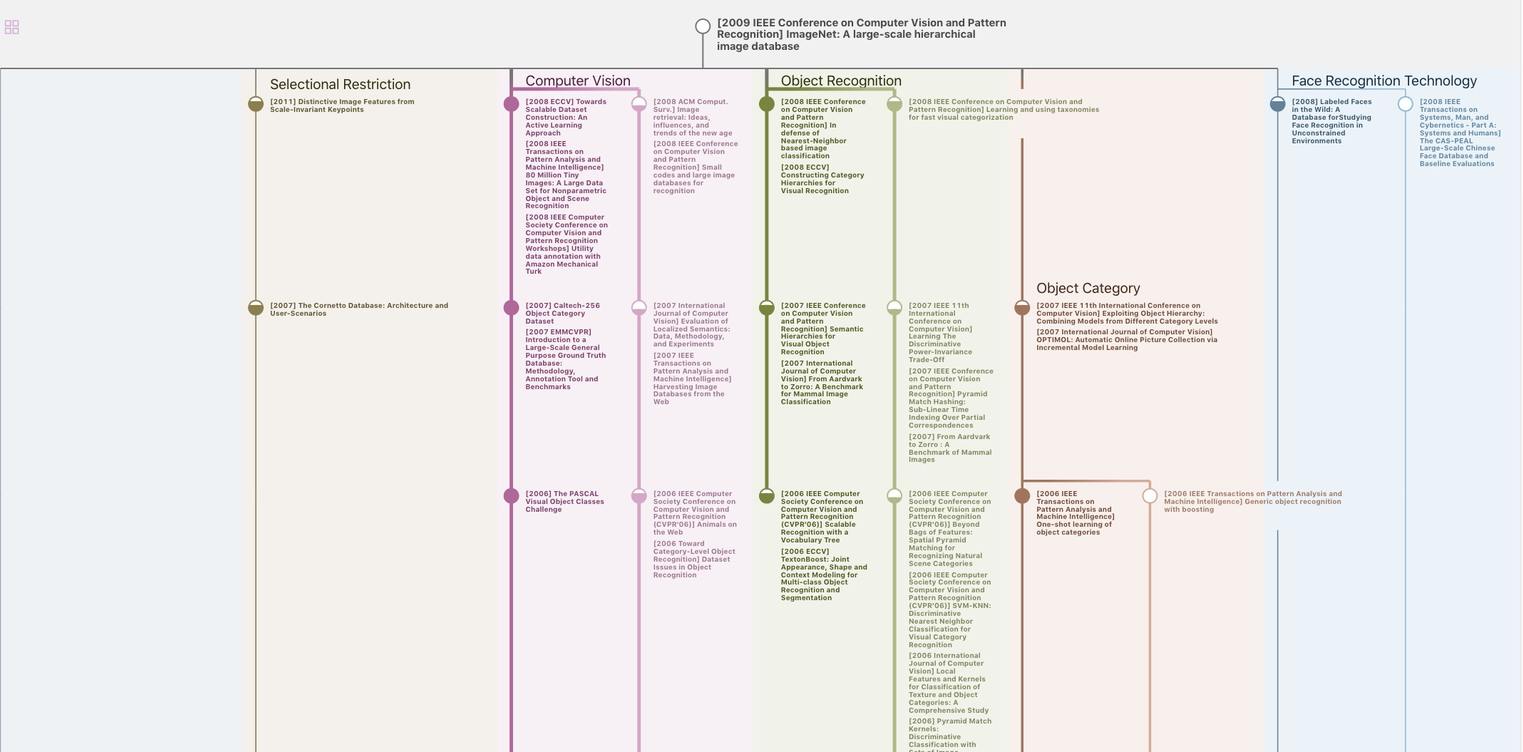
生成溯源树,研究论文发展脉络
Chat Paper
正在生成论文摘要