A reduced order schwarz method for nonlinear multiscale elliptic equations based on two-layer neural networks
JOURNAL OF COMPUTATIONAL MATHEMATICS(2023)
摘要
Neural networks are powerful tools for approximating high dimensional data that have been used in many contexts, including solution of partial differential equations (PDEs). We describe a solver for multiscale fully nonlinear elliptic equations that makes use of domain decomposition, an accelerated Schwarz framework, and two-layer neural networks to approximate the boundary-to-boundary map for the subdomains, which is the key step in the Schwarz procedure. Conventionally, the boundary-to-boundary map requires solution of boundary-value elliptic problems on each subdomain. By leveraging the compressibility of multiscale problems, our approach trains the neural network offline to serve as a surrogate for the usual implementation of the boundary-to-boundary map. Our method is applied to a multiscale semilinear elliptic equation and a multiscale p-Laplace equation. In both cases we demonstrate significant improvement in efficiency as well as good accuracy and generalization performance.
更多查看译文
关键词
Nonlinear homogenization,Multiscale elliptic problem,Neural networks,Domain decomposition
AI 理解论文
溯源树
样例
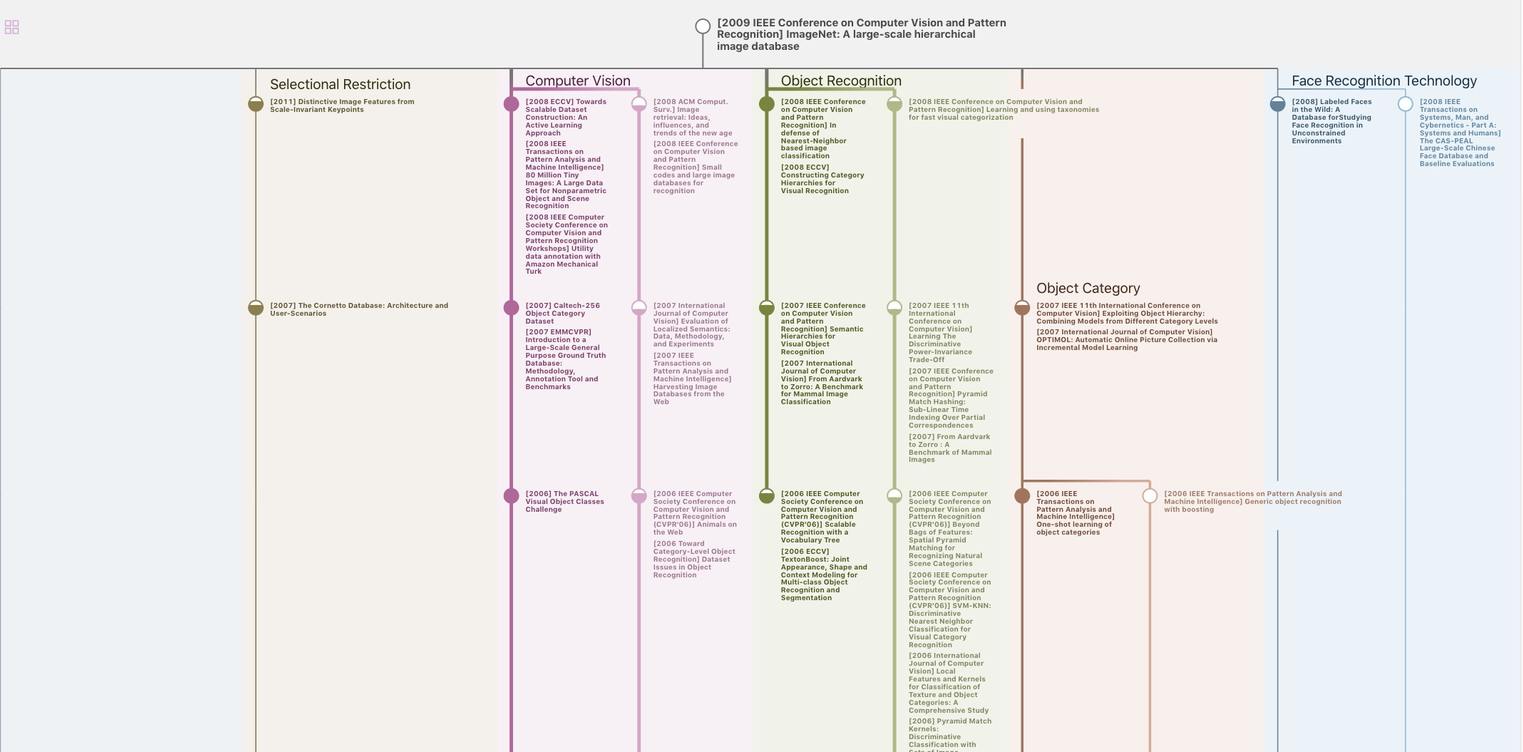
生成溯源树,研究论文发展脉络
Chat Paper
正在生成论文摘要