A joint model for multivariate longitudinal and survival data to discover the conversion to Alzheimer's disease
STATISTICS IN MEDICINE(2022)
摘要
Alzheimer's disease (AD) is an incurable and progressive disease that starts from mild cognitive impairment and deteriorates over time. Examining the effects of patients' longitudinal cognitive decline on time to conversion to AD and obtaining a reliable diagnostic model are therefore critical to the evaluation of AD prognosis and early treatment. Previous studies either assess patients' cognitive impairment through a single cognitive test or assume it changes linearly across time, thereby leading to an incomplete measure of cognitive decline or overlooking the subtle trajectory pattern of patients' cognitive impairment. This study develops a new joint model to address these shortcomings. First, a dynamic factor analysis model is adopted to characterize cognitive impairment through multiple cognitive measures in a comprehensive manner. Second, a spline-based random coefficient model is proposed to reveal possibly nonlinear trajectories of patients' cognitive decline. Finally, a proportional hazard model is considered to examine the effects of time-invariant markers and time-variant cognitive impairment on AD hazards. A Bayesian approach coupled with spline approximation techniques and MCMC methods is developed to conduct statistical inference. The application of the proposed method to the Alzheimer's Disease Neuroimaging Initiative study provides new insights into the prevention of AD and shows a high prediction capacity of the proposed method.
更多查看译文
关键词
factor analysis, latent trajectory model, longitudinal responses, MCMC methods, time-to-event outcome
AI 理解论文
溯源树
样例
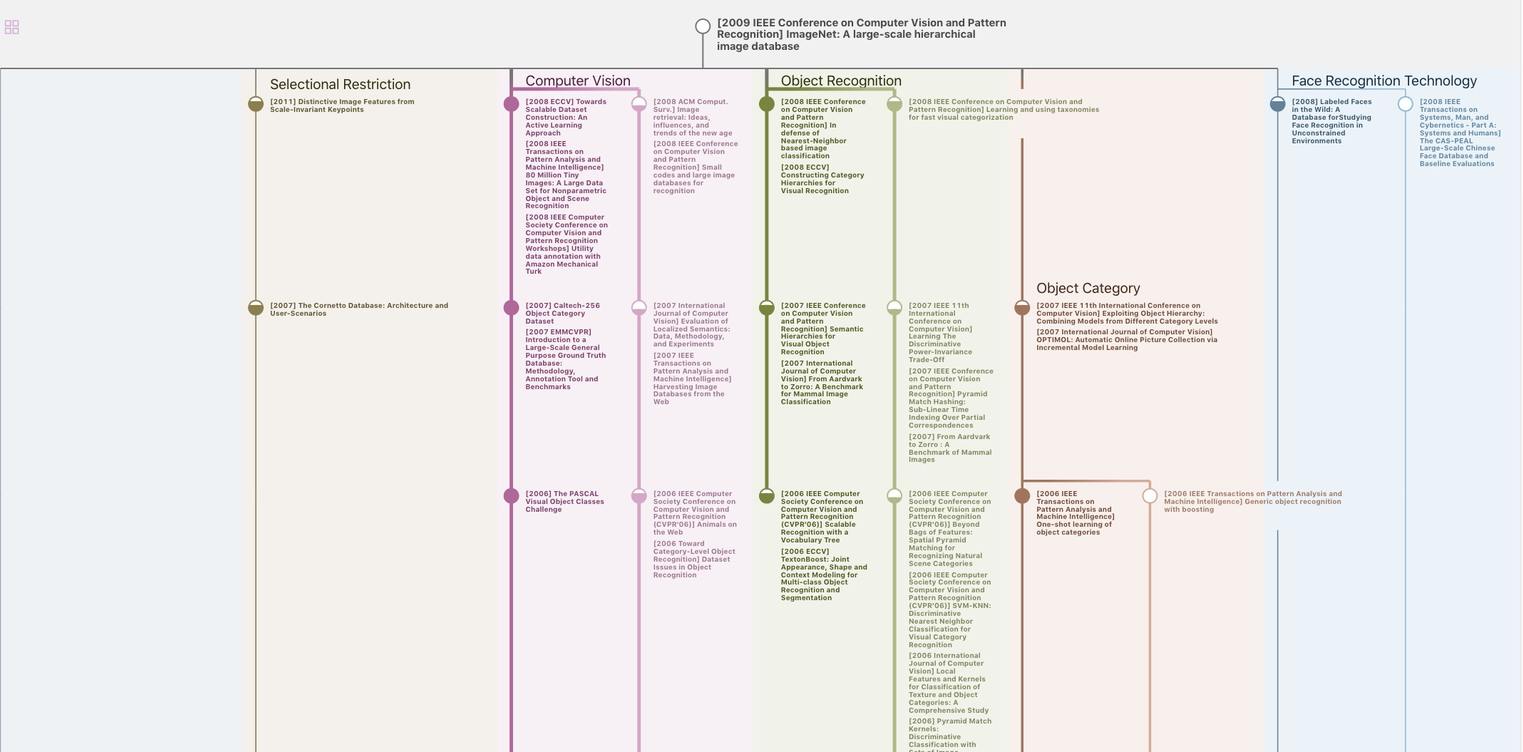
生成溯源树,研究论文发展脉络
Chat Paper
正在生成论文摘要