Closed- and Open-Vocabulary Approaches to Text Analysis: A Review, Quantitative Comparison, and Recommendations
PSYCHOLOGICAL METHODS(2021)
摘要
Technology now makes it possible to understand efficiently and at large scale how people use language to reveal their everyday thoughts, behaviors, and emotions. Written text has been analyzed through both theory-based, closed-vocabulary methods from the social sciences as well as datadriven, open-vocabulary methods from computer science, but these approaches have not been comprehensively compared. To provide guidance on best practices for automatically analyzing written text, this narrative review and quantitative synthesis compares five predominant closed- and open-vocabulary methods: Linguistic Inquiry and Word Count (LIWC), the General Inquirer, DICTION, Latent Dirichlet Allocation, and Differential Language Analysis. We compare the linguistic features associated with gender, age, and personality across the five methods using an existing dataset of Facebook status updates and self-reported survey data from 65,896 users. Results are fairly consistent across methods. The closed-vocabulary approaches efficiently summarize concepts and are helpful for understanding how people think, with LIWC2015 yielding the strongest, most parsimonious results. Open- vocabulary approaches reveal more specific and concrete patterns across a broad range of content domains, better address ambiguous word senses, and are less prone to misinterpretation, suggesting that they are well-suited for capturing the nuances of everyday psychological processes. We detail several errors that can occur in closed-vocabulary analyses, the impact of sample size, number of words per user and number of topics included in open-vocabulary analyses, and implications of different analytical decisions. We conclude with recommendations for researchers, advocating for a complementary approach that combines closed- and open-vocabulary methods. Translational Abstract A considerable amount of text data exists online that capture people's everyday thoughts, emotions, and behaviors. Technological advances now make it possible to analyze such data efficiently and at large scale, providing insights into everyday psychological processes as they occur in the real world. To provide guidance on best practice approaches for using such data effectively, this synthesis reviews and quantitively compares the main closed-vocabulary approaches (theoretically derived lists of words from the social sciences) and open-vocabulary approaches (data-driven techniques from computer science that explore many words, phrases, and topics) for automated text analysis. We find that the different methods are complementary; closed-vocabulary approaches provide a way to study the fundamental patterns of how people think and feel, whereas open-vocabulary approaches best elucidate what people think and feel.
更多查看译文
关键词
text analysis, computational social science, method comparison, language, natural language processing
AI 理解论文
溯源树
样例
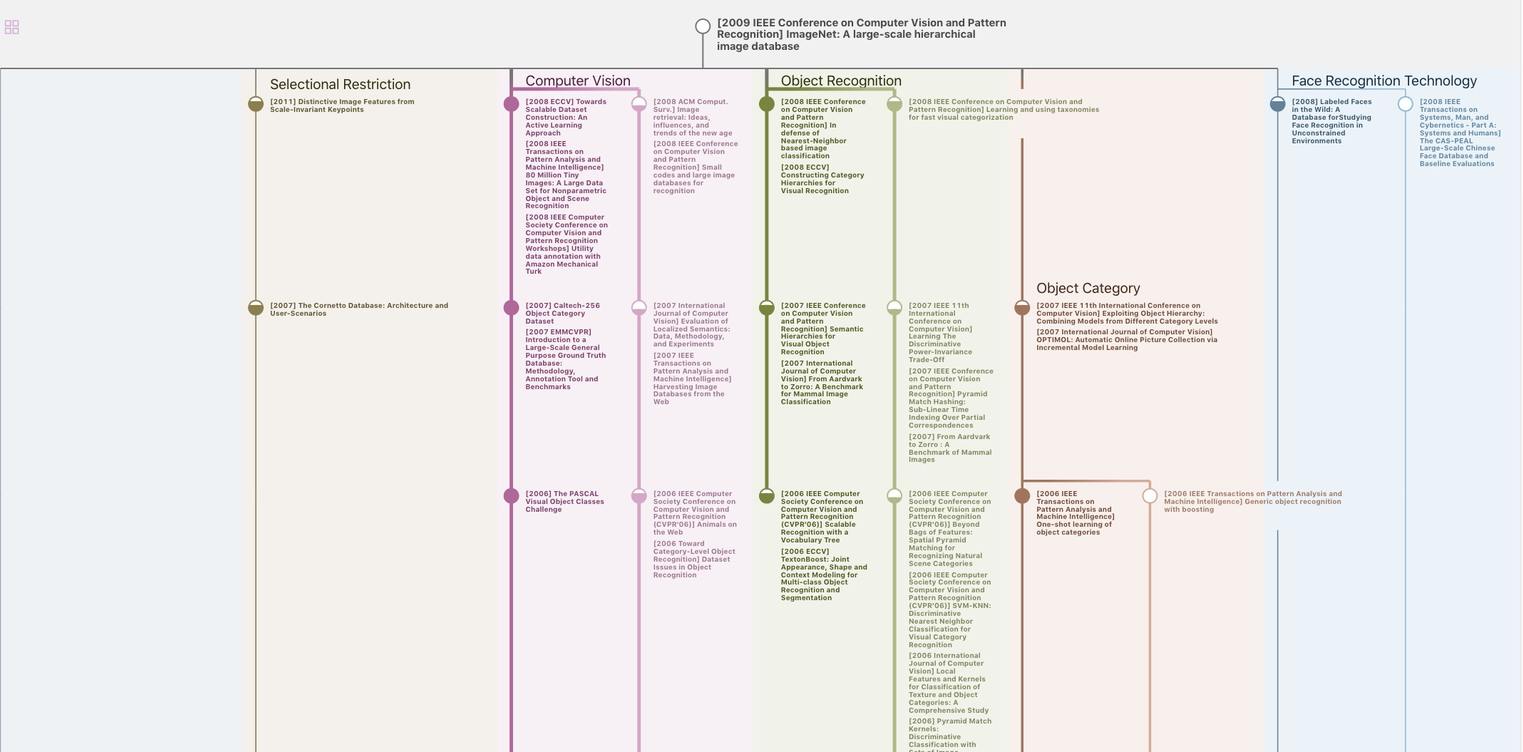
生成溯源树,研究论文发展脉络
Chat Paper
正在生成论文摘要