Trajectory Prediction with Graph-based Dual-scale Context Fusion.
IEEE/RJS International Conference on Intelligent RObots and Systems (IROS)(2022)
摘要
Motion prediction for traffic participants is essential for a safe and robust automated driving system, especially in cluttered urban environments. However, it is highly challenging due to the complex road topology as well as the uncertain intentions of the other agents. In this paper, we present a graph-based trajectory prediction network named the Dual Scale Predictor (DSP), which encodes both the static and dynamical driving context in a hierarchical manner. Different from methods based on a rasterized map or sparse lane graph, we consider the driving context as a graph with two layers, focusing on both geometrical and topological features. Graph neural networks (GNNs) are applied to extract features with different levels of granularity, and features are subsequently aggregated with attention-based inter-layer networks, realizing better local-global feature fusion. Following the recent goal-driven trajectory prediction pipeline, goal candidates with high likelihood for the target agent are extracted, and predicted trajectories are generated conditioned on these goals. Thanks to the proposed dual-scale context fusion network, our DSP is able to generate accurate and human-like multi-modal trajectories. We evaluate the proposed method on the large-scale Argoverse motion forecasting benchmark, and it achieves promising results, outperforming the recent state-of-the-art methods.
更多查看译文
关键词
trajectory,prediction,fusion,context,graph-based,dual-scale
AI 理解论文
溯源树
样例
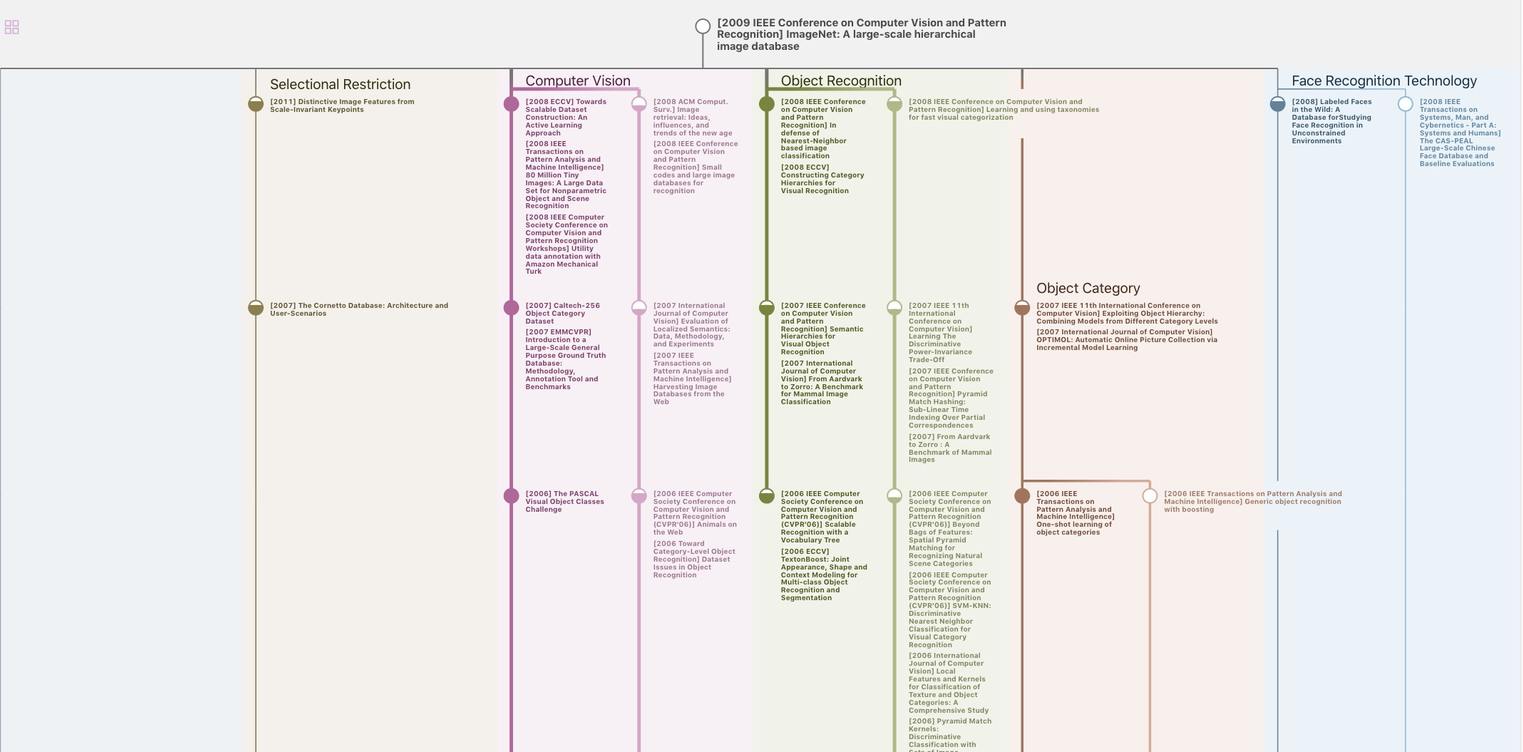
生成溯源树,研究论文发展脉络
Chat Paper
正在生成论文摘要