Can Vision Transformers Perform Convolution?
arXiv (Cornell University)(2021)
Abstract
Several recent studies have demonstrated that attention-based networks, such as Vision Transformer (ViT), can outperform Convolutional Neural Networks (CNNs) on several computer vision tasks without using convolutional layers. This naturally leads to the following questions: Can a self-attention layer of ViT express any convolution operation? In this work, we prove that a single ViT layer with image patches as the input can perform any convolution operation constructively, where the multi-head attention mechanism and the relative positional encoding play essential roles. We further provide a lower bound on the number of heads for Vision Transformers to express CNNs. Corresponding with our analysis, experimental results show that the construction in our proof can help inject convolutional bias into Transformers and significantly improve the performance of ViT in low data regimes.
MoreTranslated text
Key words
vision transformers perform convolution
AI Read Science
Must-Reading Tree
Example
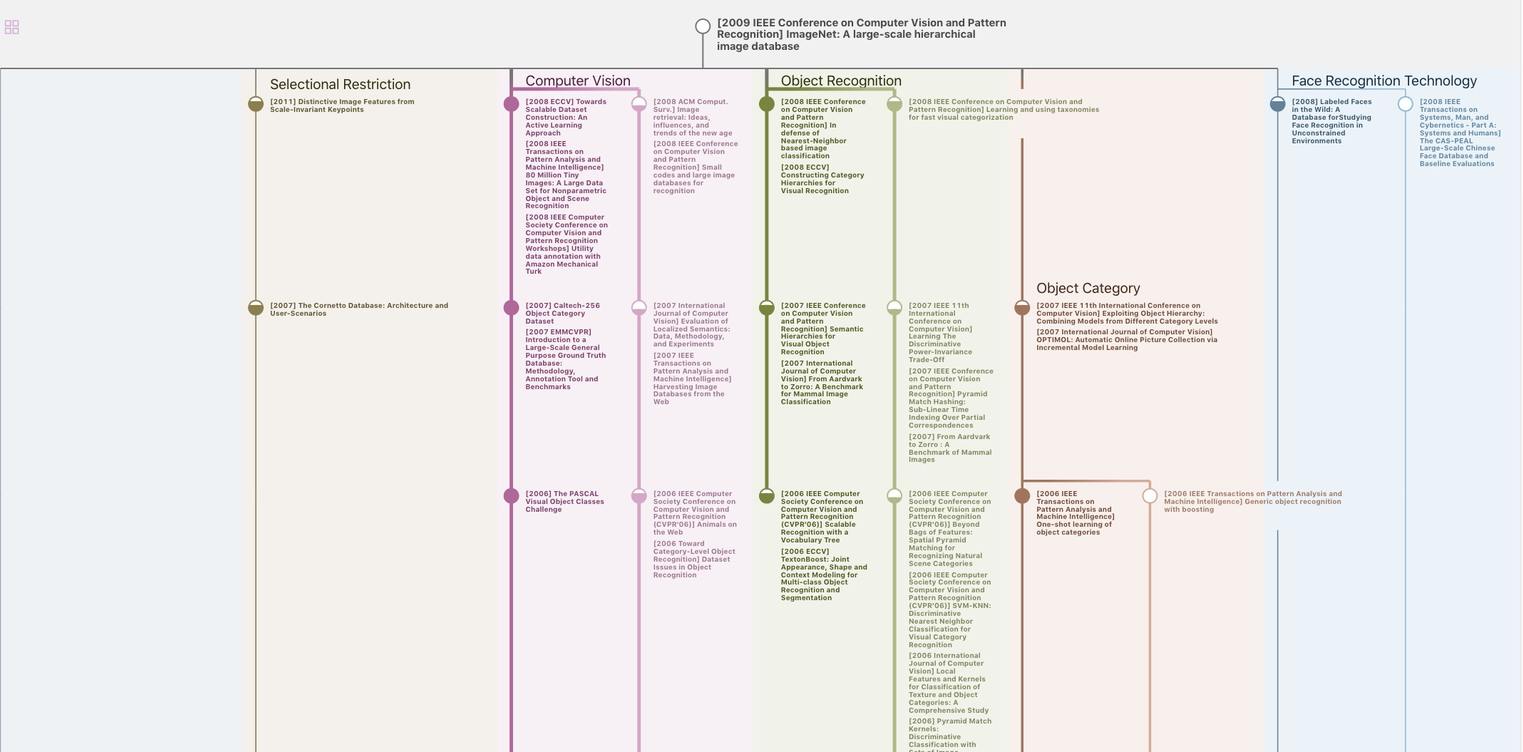
Generate MRT to find the research sequence of this paper
Chat Paper
Summary is being generated by the instructions you defined