Diverse Distributions of Self-Supervised Tasks for Meta-Learning in NLP.
EMNLP(2021)
摘要
Meta-learning considers the problem of learning an efficient learning process that can leverage its past experience to accurately solve new tasks. However, the efficacy of meta-learning crucially depends on the distribution of tasks available for training, and this is often assumed to be known a priori or constructed from limited supervised datasets. In this work, we aim to provide task distributions for meta-learning by considering self-supervised tasks automatically proposed from unlabeled text, to enable large-scale meta-learning in NLP. We design multiple distributions of self-supervised tasks by considering important aspects of task diversity, difficulty, type, domain, and curriculum, and investigate how they affect meta-learning performance. Our analysis shows that all these factors meaningfully alter the task distribution, some inducing significant improvements in downstream few-shot accuracy of the meta-learned models. Empirically, results on 20 downstream tasks show significant improvements in few-shot learning -- adding up to +4.2% absolute accuracy (on average) to the previous unsupervised meta-learning method, and perform comparably to supervised methods on the FewRel 2.0 benchmark.
更多查看译文
关键词
nlp,tasks,self-supervised,meta-learning
AI 理解论文
溯源树
样例
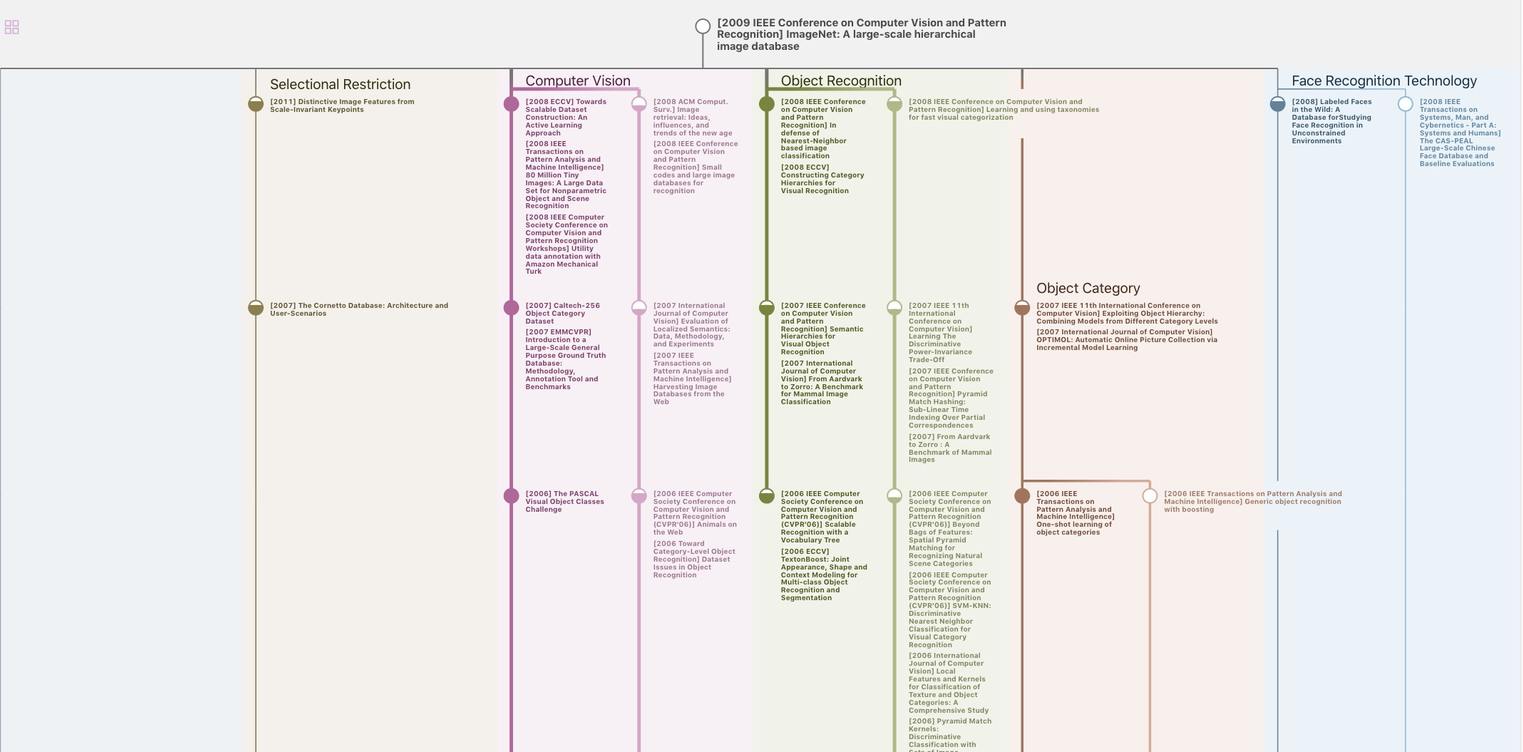
生成溯源树,研究论文发展脉络
Chat Paper
正在生成论文摘要