Epileptic Seizure Prediction Using Deep Transformer Model
INTERNATIONAL JOURNAL OF NEURAL SYSTEMS(2022)
摘要
The electroencephalogram (EEG) is the most promising and efficient technique to study epilepsy and record all the electrical activity going in our brain. Automated screening of epilepsy through data-driven algorithms reduces the manual workload of doctors to diagnose epilepsy. New algorithms are biased either towards signal processing or deep learning, which holds subjective advantages and disadvantages. The proposed pipeline is an end-to-end automated seizure prediction framework with a Fourier transform feature extraction and deep learning-based transformer model, a blend of signal processing and deep learning - this imbibes the potential features to automatically identify the attentive regions in EEG signals for effective screening. The proposed pipeline has demonstrated superior performance on the benchmark dataset with average sensitivity and false-positive rate per hour (FPR/h) as 98.46%, 94.83% and 0.12439, 0, respectively. The proposed work shows great results on the benchmark datasets and a big potential for clinics as a support system with medical experts monitoring the patients.
更多查看译文
关键词
Machine learning, deep learning, seizure prediction, epilepsy, transformer model, intracranial electroencephalogram, scalp electroencephalogram
AI 理解论文
溯源树
样例
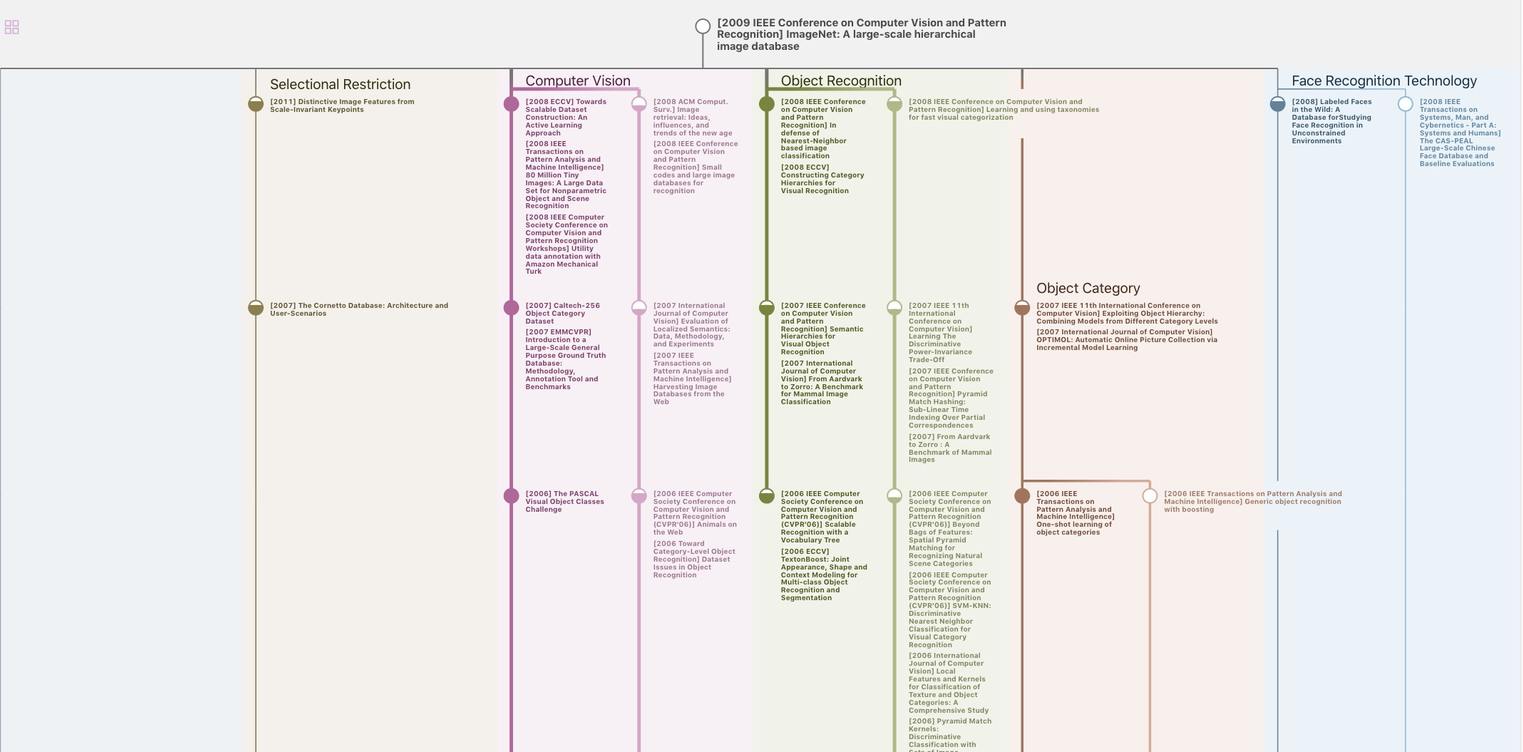
生成溯源树,研究论文发展脉络
Chat Paper
正在生成论文摘要