Pansharpening approach via two-stream detail injection based on relativistic generative adversarial networks
Expert Systems with Applications(2022)
摘要
The goal of pansharpening technique is to reconstruct a high resolution spectral image (HRMS) from a low spatial resolution multispectral image (MS) and a high spatial resolution single band panchromatic (PAN) image. As the efficacy of such a technique relies upon its capability to reinforce the spatial information of the MS image while conserving its spectral signature, in this paper, we propose DI-GAN, an effective Detail Injection Generative Adversarial Network for remote sensing image pansharpening. DI-GAN employs a deep two-stream Convolutional Neural Network (CNN) generator model to combine the spatial information available on the PAN image and the spectral characteristic belonging to the MS image, in order to predict the high-frequency detail to be accurately injected into the MS image to reconstruct the desired HRMS. An original loss function is particularly proposed to encourage the network to predict only high-frequency detail. DI-GAN incorporates in parallel a Relativistic Discriminator that allows the pansharpening products to be more realistic. Experimental results at degraded and full scale on three different datasets were showed that the proposed fusion approach can produce superior pansharpening performances in terms of spatial and spectral fidelities with respect to the literature techniques, including CNN- and GAN-based methods.
更多查看译文
关键词
Pansharpening,CNN,Detail injection,Relativistic GAN
AI 理解论文
溯源树
样例
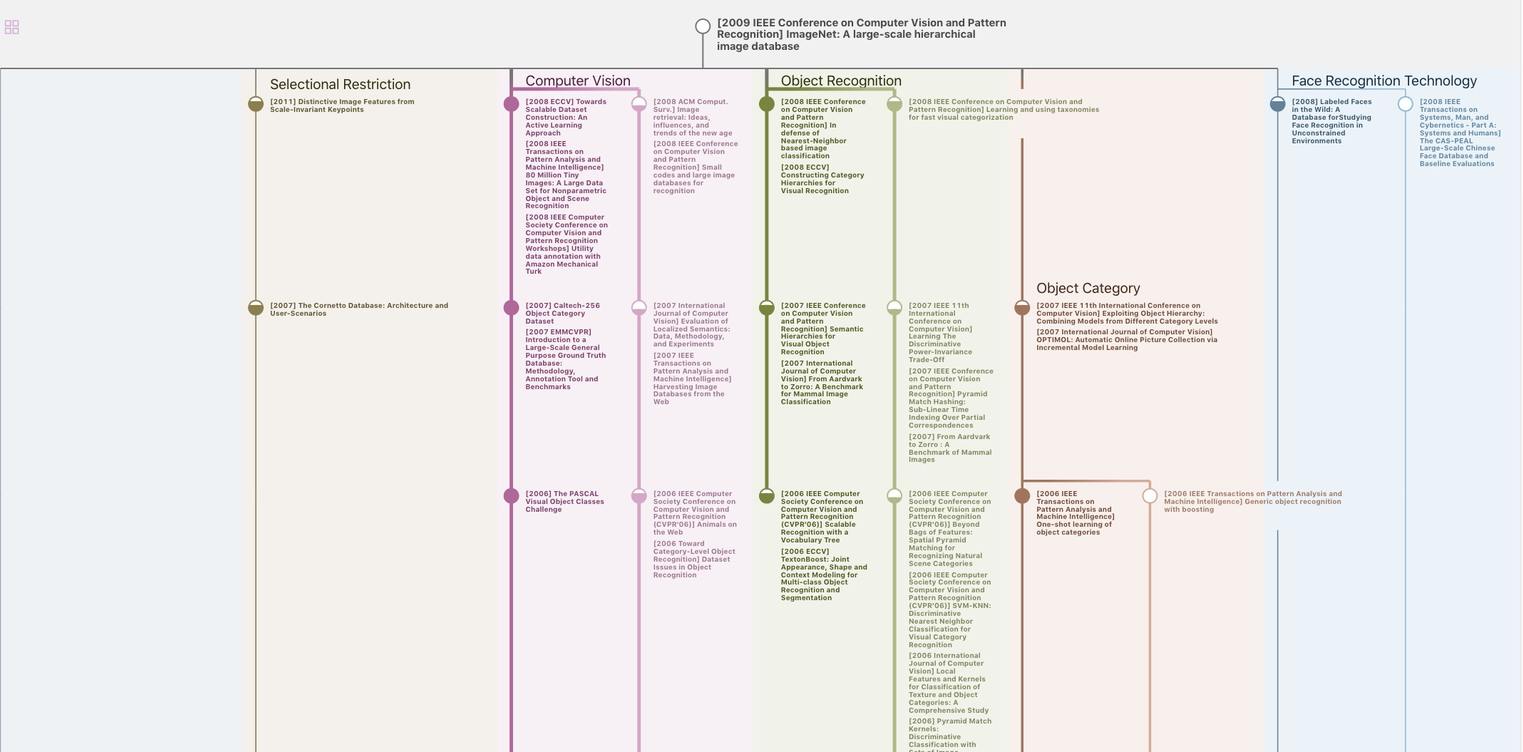
生成溯源树,研究论文发展脉络
Chat Paper
正在生成论文摘要