When is resampling beneficial for feature selection with imbalanced wide data?
Expert Systems with Applications(2022)
摘要
This paper studies the effects that combinations of balancing and feature selection techniques have on wide data (many more attributes than instances) when different classifiers are used. For this, an extensive study is done using 14 datasets, 3 balancing strategies, and 7 feature selection algorithms. The evaluation is carried out using 5 classification algorithms, analyzing the results for different percentages of selected features, and establishing the statistical significance using Bayesian tests.
更多查看译文
关键词
Feature selection,Wide data,High dimensional data,Very low sample size,Unbalanced,Machine learning
AI 理解论文
溯源树
样例
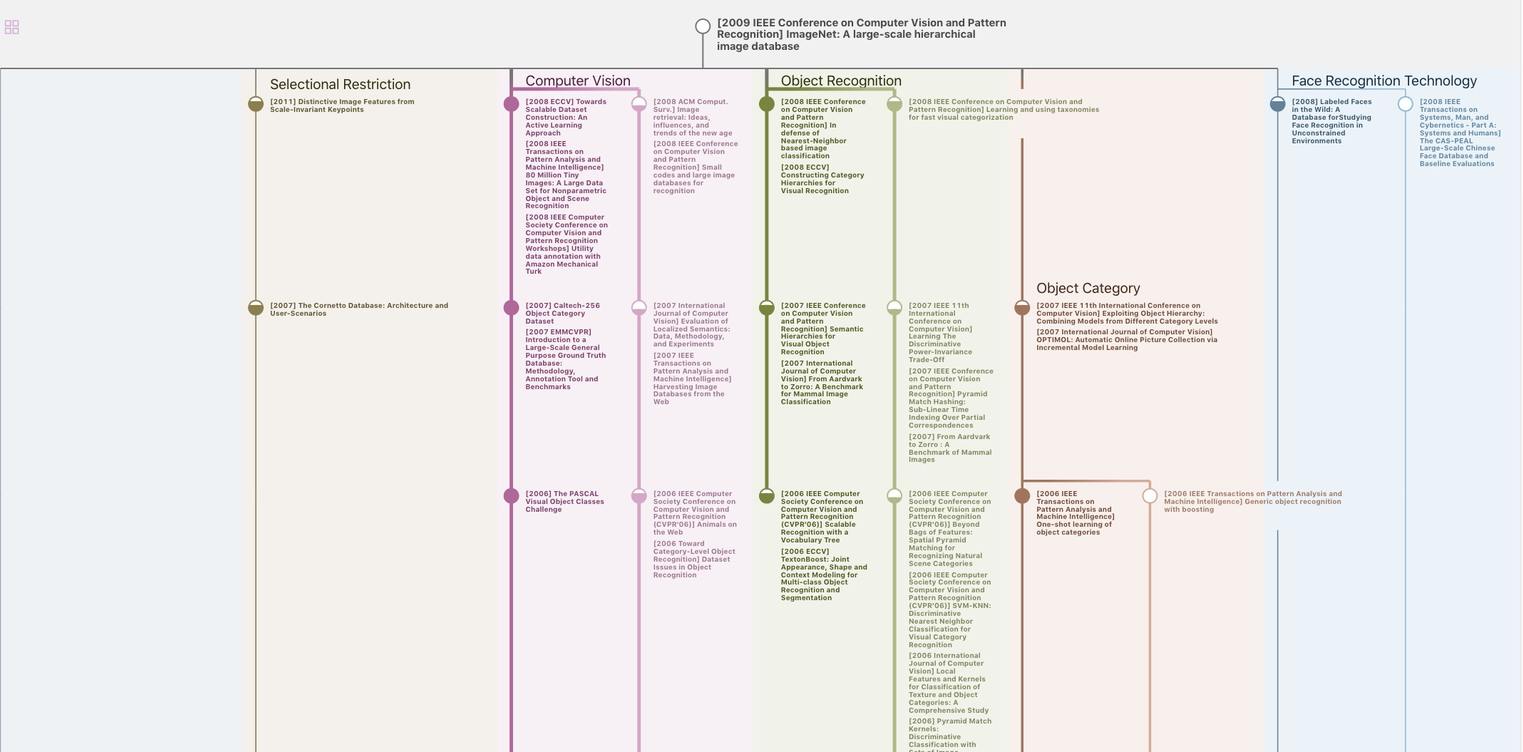
生成溯源树,研究论文发展脉络
Chat Paper
正在生成论文摘要