Fast sky localization of gravitational waves using deep learning seeded importance sampling
arxiv(2022)
摘要
Fast, highly accurate, and reliable inference of the sky origin of gravitational waves would enable real-time multimessenger astronomy. Current Bayesian inference methodologies, although highly accurate and reliable, are slow. Deep learning models have shown themselves to be accurate and extremely fast for inference tasks on gravitational waves, but their output is inherently questionable due to the blackbox nature of neural networks. In this work, we merge Bayesian inference and deep learning by applying importance sampling on an approximate posterior generated by a multiheaded convolutional neural network. The neural network parametrizes Von Mises-Fisher and Gaussian distributions for the sky coordinates and two masses for given simulated gravitational wave injections in the LIGO and Virgo detectors. We generate skymaps for unseen gravitational-wave events that highly resemble predictions generated using Bayesian inference in a few minutes. Furthermore, we can detect poor predictions from the neural network, and quickly flag them.
更多查看译文
关键词
swift sky localization,gravitational waves
AI 理解论文
溯源树
样例
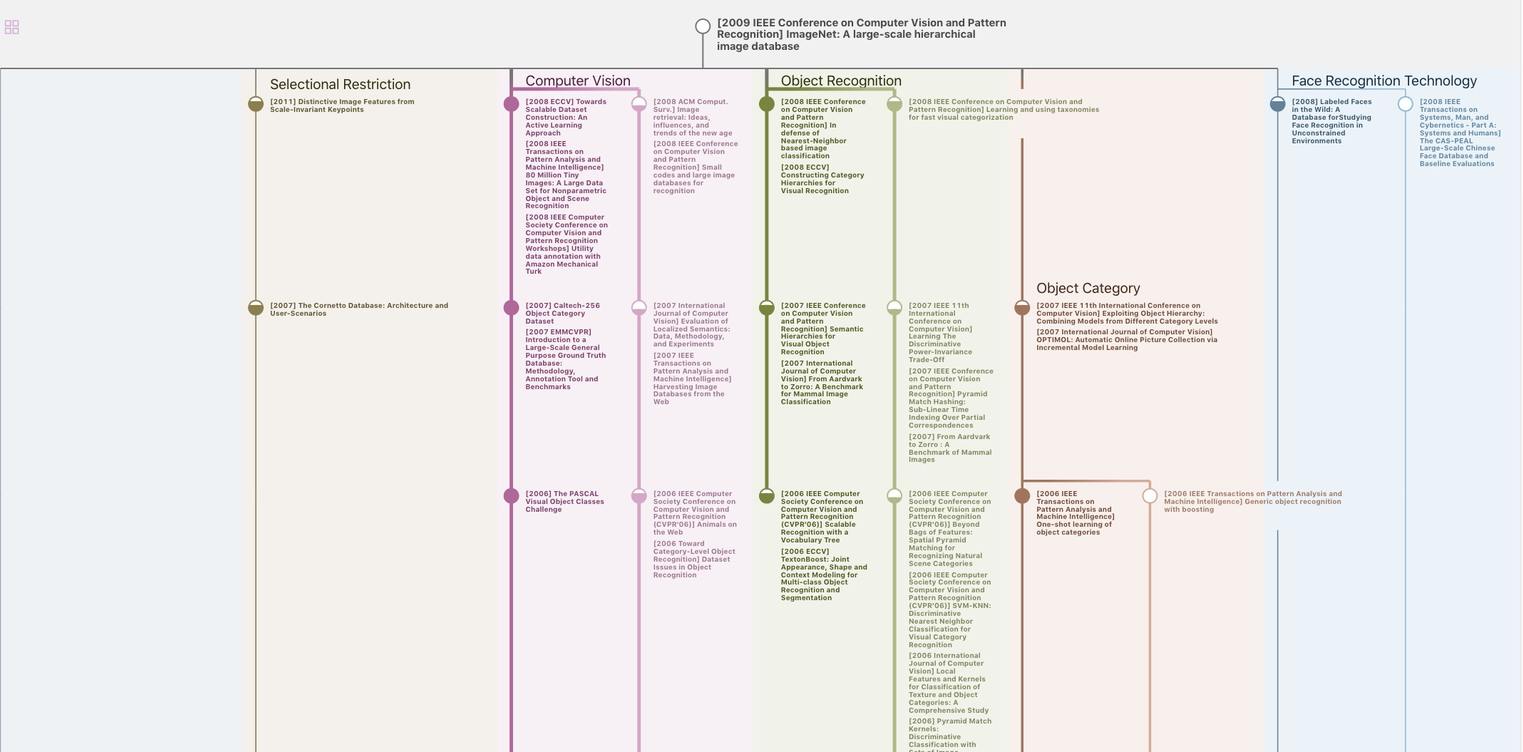
生成溯源树,研究论文发展脉络
Chat Paper
正在生成论文摘要