A machine learning approach to enhance the SUPG stabilization method for advection-dominated differential problems
MATHEMATICS IN ENGINEERING(2023)
摘要
We propose using machine learning and artificial neural networks (ANNs) to enhance residual-based stabilization methods for advection-dominated differential problems. Specifically, in the context of the finite element method, we consider the streamline upwind Petrov-Galerkin (SUPG) stabilization method and we employ ANNs to optimally choose the stabilization parameter on which the method relies. We generate our dataset by solving optimization problems to find the optimal stabilization parameters that minimize the distances among the numerical and the exact solutions for different data of differential problem and the numerical settings of the finite element method, e.g., mesh size and polynomial degree. The dataset generated is used to train the ANN, and we used the latter "online" to predict the optimal stabilization parameter to be used in the SUPG method for any given numerical setting and problem data. We show, by means of 1D and 2D numerical tests for the advection-dominated differential problem, that our ANN approach yields more accurate solution than using the conventional stabilization parameter for the SUPG method.
更多查看译文
关键词
partial differential equations,finite element method,stabilization methods,streamline upwind Petrov-Galerkin,machine learning,artificial neural networks
AI 理解论文
溯源树
样例
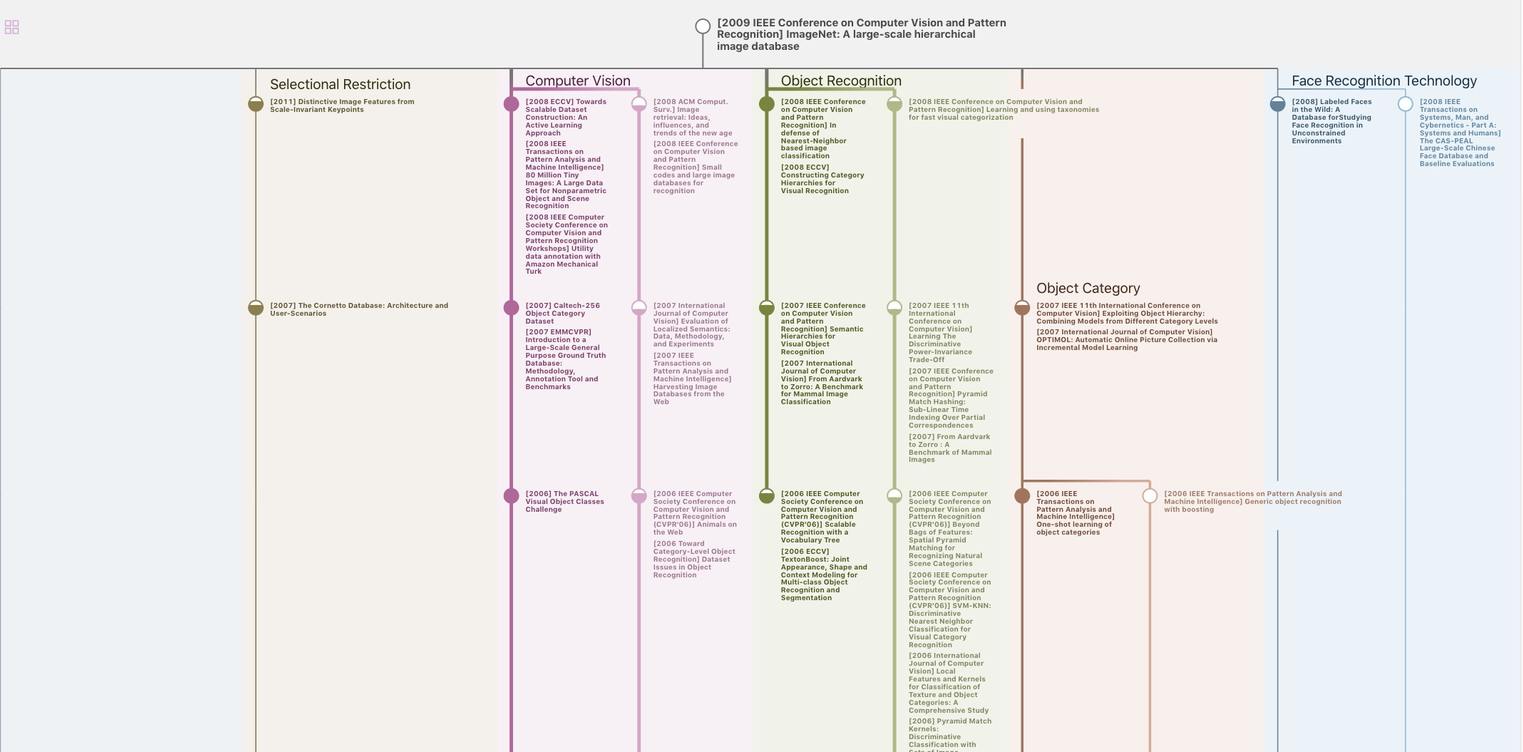
生成溯源树,研究论文发展脉络
Chat Paper
正在生成论文摘要