Optimal Compression of Locally Differentially Private Mechanisms
INTERNATIONAL CONFERENCE ON ARTIFICIAL INTELLIGENCE AND STATISTICS, VOL 151(2022)
摘要
Compressing the output of epsilon-locally differentially private (LDP) randomizers naively leads to suboptimal utility. In this work, we demonstrate the benefits of using schemes that jointly compress and privatize the data using shared randomness. In particular, we investigate a family of schemes based on Minimal Random Coding (Havasi et al., 2019) and prove that they offer optimal privacy-accuracy-communication tradeoffs. Our theoretical and empirical findings show that our approach can compress PrivUnit(2) (Bhowmick et al., 2018) and Subset Selection (Ye and Barg, 2018), the best known LDP algorithms for mean and frequency estimation, to the order of e bits of communication while preserving their privacy and accuracy guarantees.
更多查看译文
关键词
private mechanisms
AI 理解论文
溯源树
样例
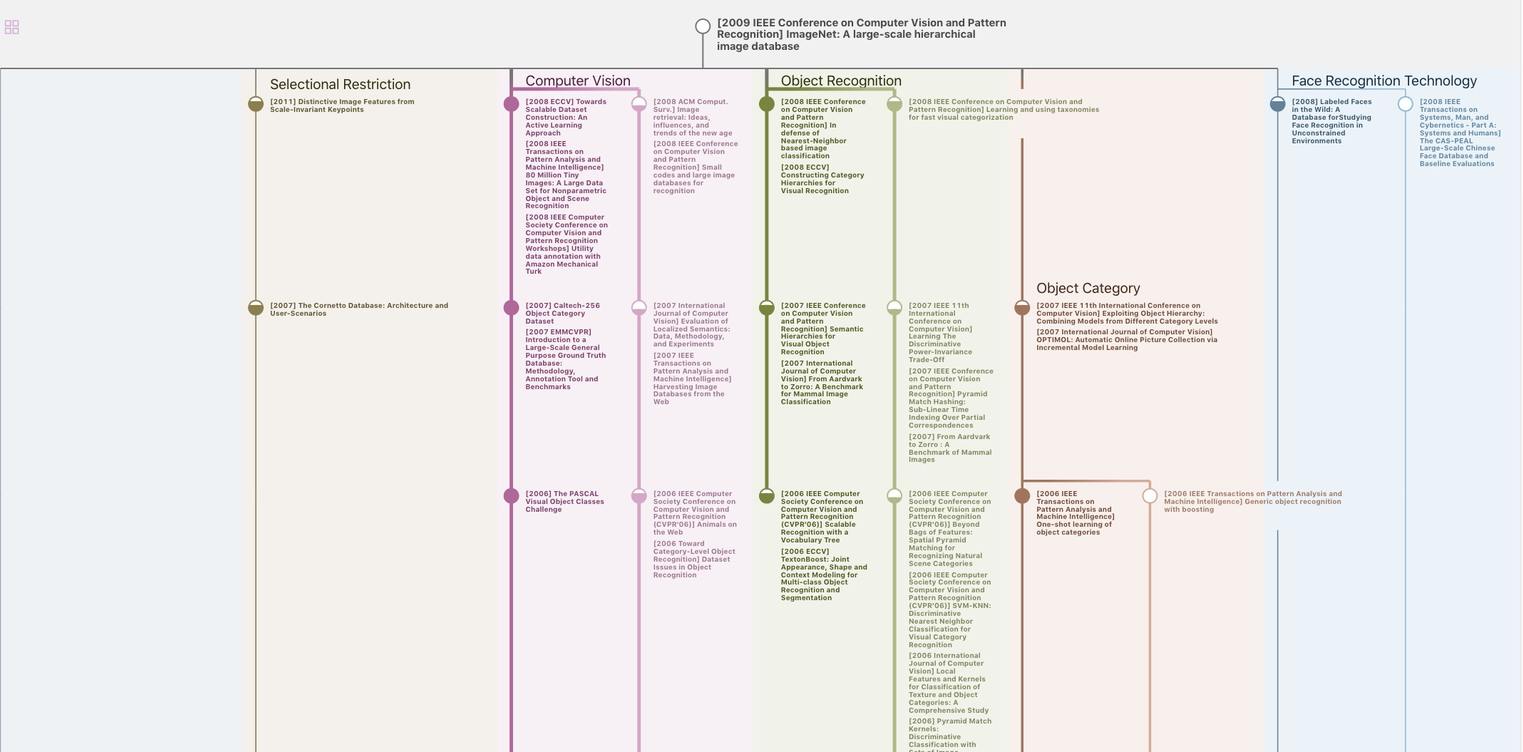
生成溯源树,研究论文发展脉络
Chat Paper
正在生成论文摘要