A proof-of-principle study on implementing polymerase chain displacement reaction (PCDR) to improve forensic low-template DNA analysis
Forensic Science International: Genetics(2022)
摘要
Polymerase chain reaction (PCR) plays an important role in forensic DNA analysis. However, the amplification of low-template DNA (LTDNA) samples usually encounters unsatisfactory results for the limited efficiency of PCR, which would interfere with the subsequent profile interpretation. Polymerase chain displacement reaction (PCDR) is a highly-efficient technique characterized by combining PCR and strand displacement reaction into a single PCDR cycle. This study explored the feasibility of PCDR for improving forensic LTDNA analysis. STR markers commonly used in forensic genetics were subjected to PCDR amplification and capillary electrophoresis detection. The results of singleplex reactions indicated that PCDR surpassed original PCR in efficiency for STR amplification. The average peak height of alleles in PCDR profiles was linearly correlated to the number of outer primers adopted for initiating the strand displacement process. Further, we assessed the multiplexing potential of PCDR by incorporating 17 STRs included in the expanded CODIS core loci and Amelogenin gene into a multiplex PCDR system. For pristine DNA templates ranged from 200 pg to 12.5 pg, the multiplex PCDR system consistently exhibited higher allele peak height as well as less allele dropout compared to the multiplex PCR references. Meanwhile, a significant reduction of stutter ratio was extensively observed in PCDR profiles. We also tested mock casework samples to verify the practical ability of multiplex PCDR for LTDNA detection. With DNA input varying from 48.1 pg to 6.6 pg, the multiplex PCDR system consistently obtained more allelic information than multiplex PCR methods. Our data collectively suggested that it is feasible to apply PCDR in forensic LTDNA analysis.
更多查看译文
关键词
PCR,Strand displacement reaction,Low-template DNA,STR,Stutter artifact
AI 理解论文
溯源树
样例
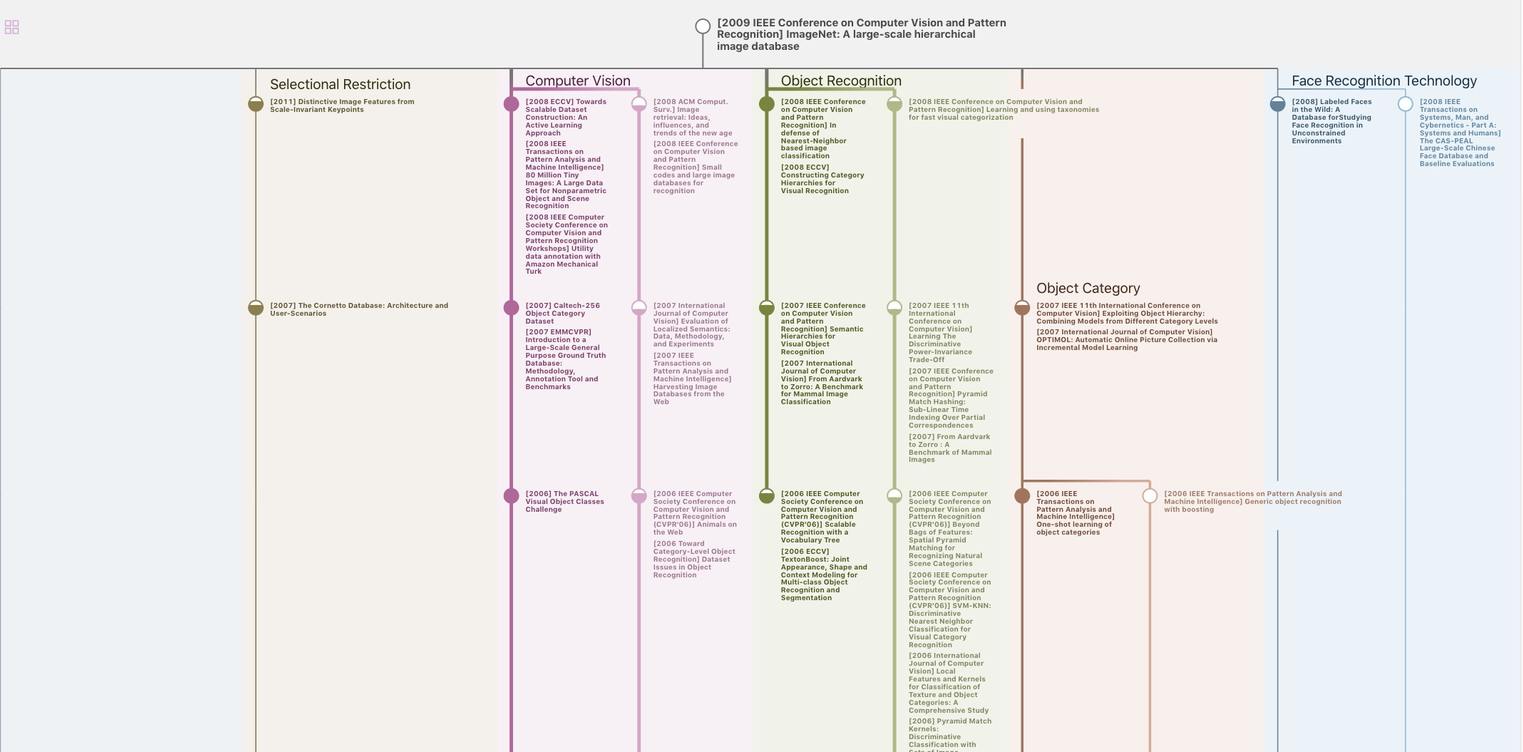
生成溯源树,研究论文发展脉络
Chat Paper
正在生成论文摘要