Contrastive Prediction Strategies for Unsupervised Segmentation and Categorization of Phonemes and Words
IEEE International Conference on Acoustics, Speech, and Signal Processing (ICASSP)(2022)
摘要
We investigate the performance on phoneme categorization and phoneme and word segmentation of several self-supervised learning (SSL) methods based on Contrastive Predictive Coding (CPC). Our experiments show that with the existing algorithms there is a trade off between categorization and segmentation performance. We investigate the source of this conflict and conclude that the use of context building networks, albeit necessary for superior performance on categorization tasks, harms segmentation performance by causing a temporal shift on the learned representations. Aiming to bridge this gap, we take inspiration from the leading approach on segmentation, which simultaneously models the speech signal at the frame and phoneme level, and incorporate multi-level modelling into Aligned CPC (ACPC), a variation of CPC which exhibits the best performance on categorization tasks. Our multi-level ACPC (mACPC) improves in all categorization metrics and achieves state-of-the-art performance in word segmentation.
更多查看译文
关键词
self-supervised learning,Contrastive Predictive,Coding,unsupervised phoneme segmentation,unsupervised word segmentation,phoneme classification
AI 理解论文
溯源树
样例
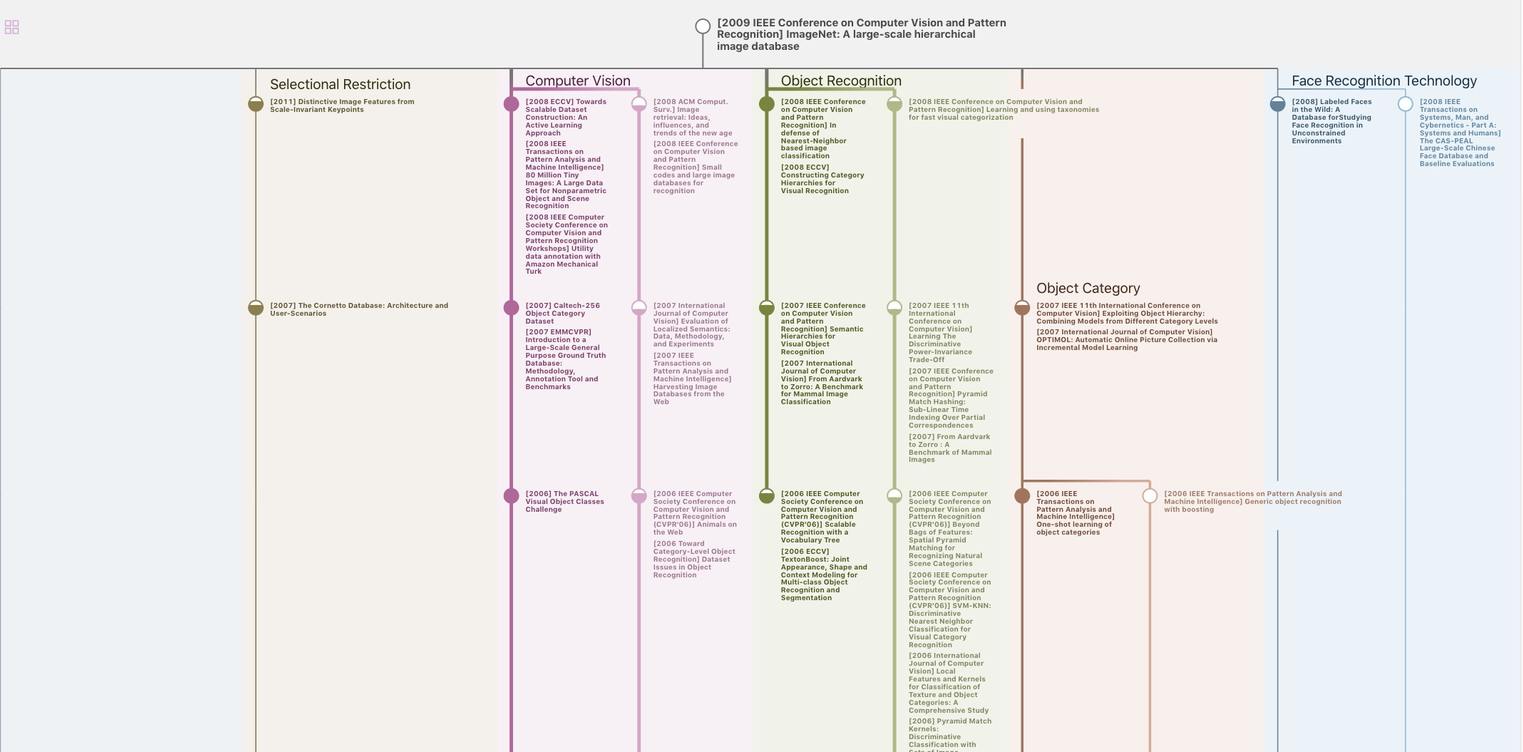
生成溯源树,研究论文发展脉络
Chat Paper
正在生成论文摘要